A Semantically Driven Hybrid Network for Unsupervised Entity Alignment
ACM Transactions on Intelligent Systems and Technology(2023)
摘要
The major challenge in the task of entity alignment (EA) lies in the heterogeneity of the knowledge graph. The traditional solution to EA is to first map entities to the same space via knowledge embedding and then calculate the similarity between entities from different knowledge graphs. However, these methods mainly rely on manually labeled seeds of EA, which limits their applicability. Some researchers have begun using pseudo-labels rather than seeds for unsupervised EA. However, directly using pseudo-labels causes new problems, such as noise in the pseudo-labels. In this article, we propose a model called the Semantically Driven Hybrid Network (SDHN) to reduce the impact of noise in the pseudo-labels on the performance of EA models. The SDHN consists of two modules: a Teacher-Student Network (TSN) and a Rotation and Penalty (RAP) module. The TSN module reduces the impact of noise in two ways: (1) The TSN's teacher network guides its student network to construct pseudo-labels based on semantic information instead of directly creating pseudo-labels. (2) It adaptively fuses semantic information into student networks to improve the final representation of entity embedding. Finally, the TSN enhances the performance of models of entity alignment via the RAP module. The results of experiments on multiple benchmark datasets showed that the SDHN outperforms state-of-the-art models.
更多查看译文
关键词
Knowledge graph,graph neural networks,entity alignment
AI 理解论文
溯源树
样例
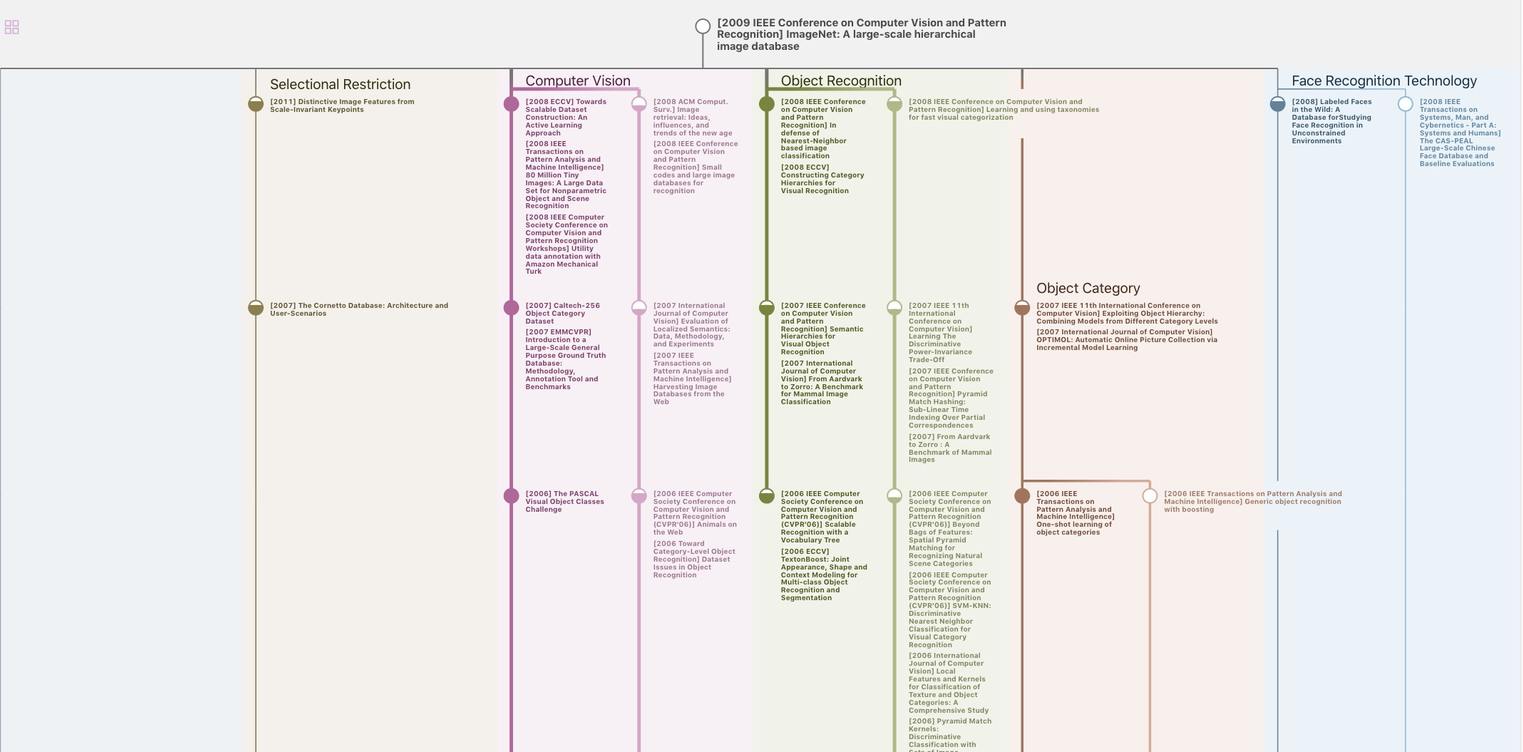
生成溯源树,研究论文发展脉络
Chat Paper
正在生成论文摘要