Impersonation Attack Detection in Mobile Edge Computing by Levering SARSA Technique in Physical Layer Security
Applied Sciences(2022)
摘要
Smart health systems typically integrate sensor technology with the Internet of Things, enabling healthcare systems to monitor patients. These biomedical applications collect healthcare data through remote sensors and transfer the data to a centralized system for analysis. However, the communication between the edge node and the mobile user is susceptible to impersonation attacks in mobile edge computing (MEC) for the biomedical application. For this purpose, we propose a detection mechanism for medical and healthcare services, i.e., reinforcement learning for impersonation attacks. We construct a system model of MEC, a key generation model (KGM), and an impersonation attack model (IAM). In addition, we also design an impersonation attack detection algorithm based on the SARSA technique under the IAM. In our proposed work, the SARSA-based method outplays the detection of impersonation attacks in the dynamic environment compared to the traditional Q-learning technique. Finally, we evaluate the false alarm rate (FAR), miss detection rate (MDR), and average error rate (AER) in the hypothesis tests to compare the performance of our proposed method with the traditional Q-learning. In comparison to the classic Q-learning based technique, simulation experiments show that the suggested approach can avoid impersonation attacks in a dynamic environment for medical and healthcare services. The results also indicate that the SARSA technique has a high detection accuracy and low average error rate compared to the conventional Q-learning based approach.
更多查看译文
关键词
network security, encrypted traffic identification, deep learning, convolutional neural network
AI 理解论文
溯源树
样例
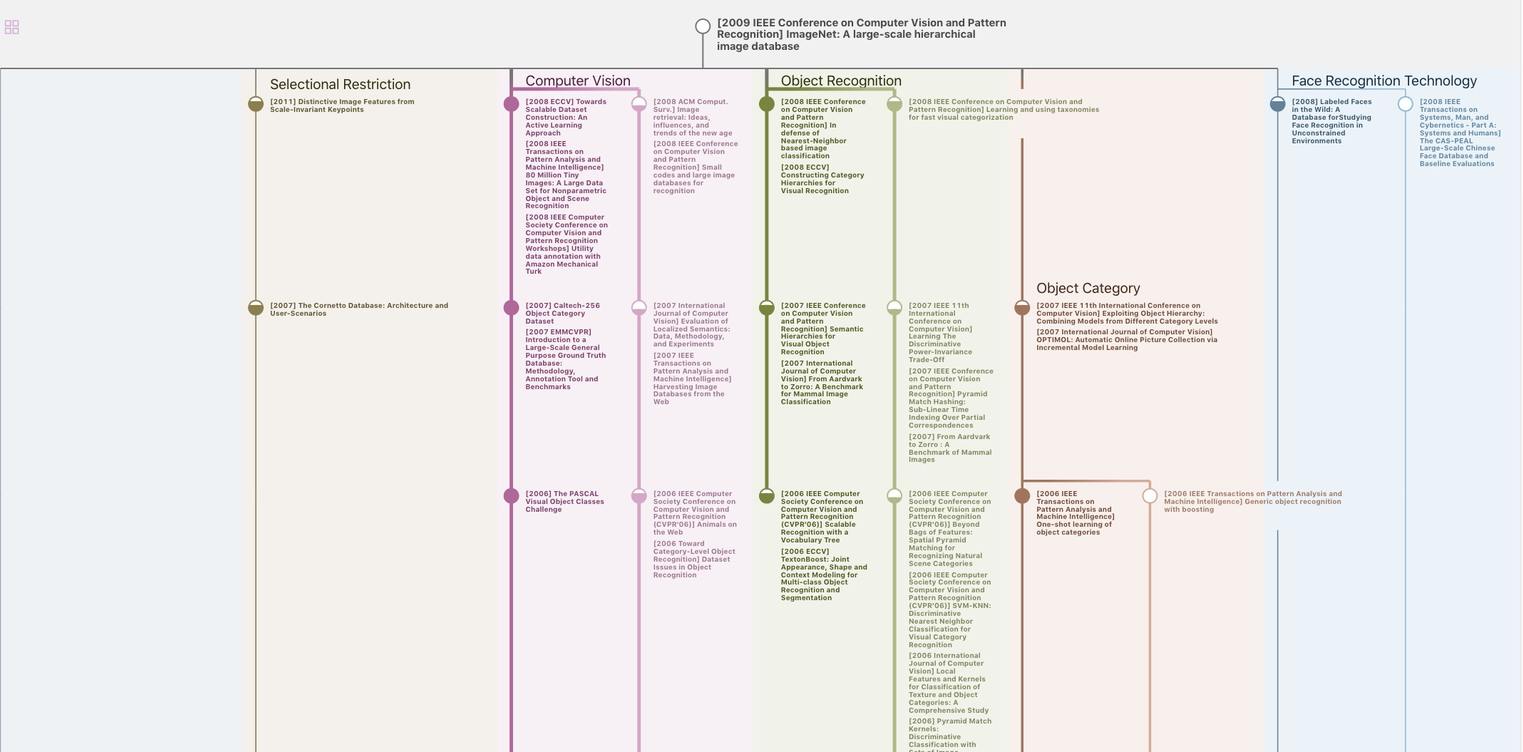
生成溯源树,研究论文发展脉络
Chat Paper
正在生成论文摘要