Neural Network-Augmented Locally Adaptive Linear Regression Model for Tabular Data
Social Science Research Network(2022)
摘要
Creating an interpretable model with high predictive performance is crucial in eXplainable AI (XAI) field. We introduce an interpretable neural network-based regression model for tabular data in this study. Our proposed model uses ordinary least squares (OLS) regression as a base-learner, and we re-update the parameters of our base-learner by using neural networks, which is a meta-learner in our proposed model. The meta-learner updates the regression coefficients using the confidence interval formula. We extensively compared our proposed model to other benchmark approaches on public datasets for regression task. The results showed that our proposed neural network-based interpretable model showed outperformed results compared to the benchmark models. We also applied our proposed model to the synthetic data to measure model interpretability, and we showed that our proposed model can explain the correlation between input and output variables by approximating the local linear function for each point. In addition, we trained our model on the economic data to discover the correlation between the central bank policy rate and inflation over time. As a result, it is drawn that the effect of central bank policy rates on inflation tends to strengthen during a recession and weaken during an expansion. We also performed the analysis on CO2 emission data, and our model discovered some interesting explanations between input and target variables, such as a parabolic relationship between CO2 emissions and gross national product (GNP). Finally, these experiments showed that our proposed neural network-based interpretable model could be applicable for many real-world applications where data type is tabular and explainable models are required.
更多查看译文
关键词
interpretable model,linear regression,neural network,adaptive learning,tabular data,economic management,environmental economics
AI 理解论文
溯源树
样例
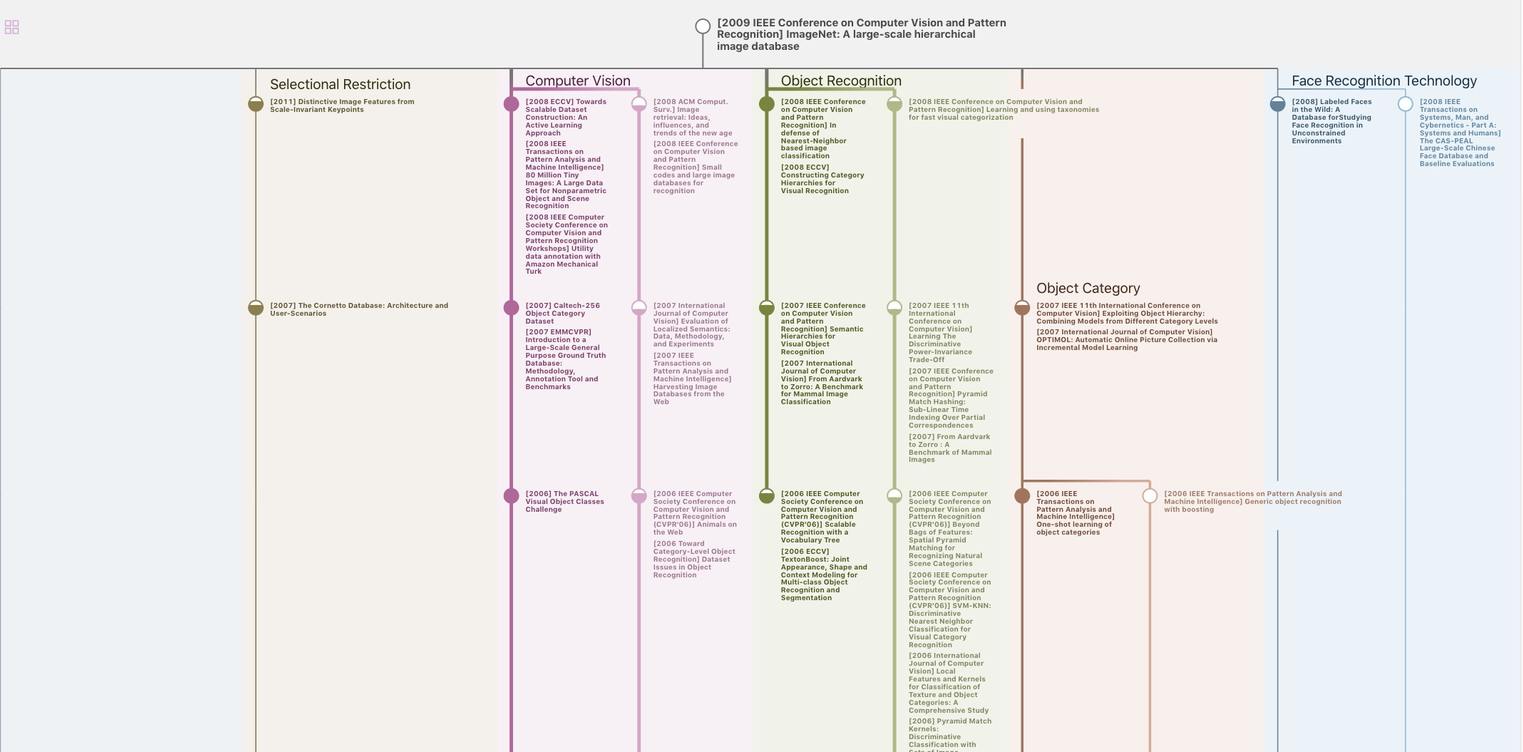
生成溯源树,研究论文发展脉络
Chat Paper
正在生成论文摘要