A Generative Cramér-Rao Bound on Frequency Estimation with Learned Measurement Distribution
2022 IEEE 12th Sensor Array and Multichannel Signal Processing Workshop (SAM)(2022)
摘要
The estimation of the frequency of a single tone signal is a classical problem. The Cramér-Rao lower bound (CRB) on the frequency estimates has been well studied for the case of additive Gaussian noise. In practical applications, however, the probability density function of the noise is rarely Gaussian, or known. Moreover, non-linear effects, as quantization, are often present, making the Gaussian CRB unreachable. In this paper we propose a data-driven approach for evaluating the CRB on frequency estimation with unknown noise and other degradation. Using a learned normalizing flow model, we model the distribution of the measurements by a neural network and obtain an approximate CRB, referred to as a Generative CRB (GCRB). We demonstrate the GCRB on frequency estimation both in cases where the CRB has been previously evaluated, showing the accuracy of the GCRB empirically, and on complex cases where the CRB cannot be evaluated analytically or numerically.
更多查看译文
关键词
Generative model,CRB,normalizing flow,parameter estimation,deep learning
AI 理解论文
溯源树
样例
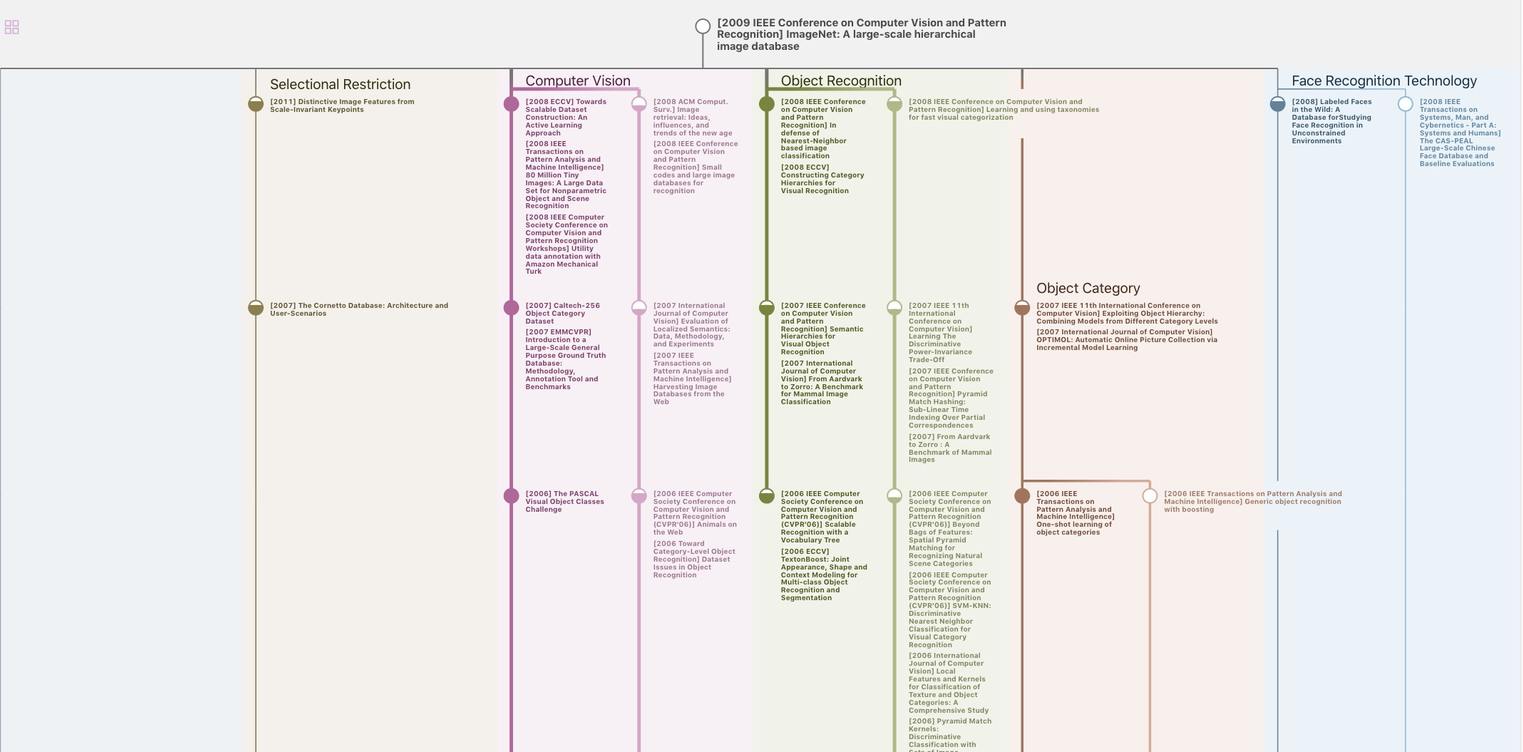
生成溯源树,研究论文发展脉络
Chat Paper
正在生成论文摘要