LogST: Log Semi-supervised Anomaly Detection Based on Sentence-BERT
2022 7th International Conference on Signal and Image Processing (ICSIP)(2022)
摘要
Semantics extraction is a very important part in the field of log anomaly detection, how to accurately obtain the semantics representation of log events will have a direct impact on the final anomaly detection results. However, existing research focuses on using word embedding to extract the semantics of log events, this method based on weighted aggregation of all word vectors may lose the semantic relationship of word order, and does not consider the interaction between words in log events, which cannot fully represent the semantics of log events. To solve this problem and further improve the anomaly detection performance, this paper proposes a novel practical log-based anomaly detection approach, LogST, which uses the SBERT model to extract the semantics representation of log events, considering the semantic and word order relationship of each word of log events, which is convenient to understand the log sequence context, and then design a GRU model for anomaly detection. Experimental results conducted on the public HDFS datasets demonstrate that LogST outperforms other methods in the case of a sufficient number of labeled normal logs, and can still guarantee the stability of anomaly detection accuracy in the case of a small number of labeled normal logs.
更多查看译文
关键词
anomaly detection,log analysis,deep learning,semantics extraction
AI 理解论文
溯源树
样例
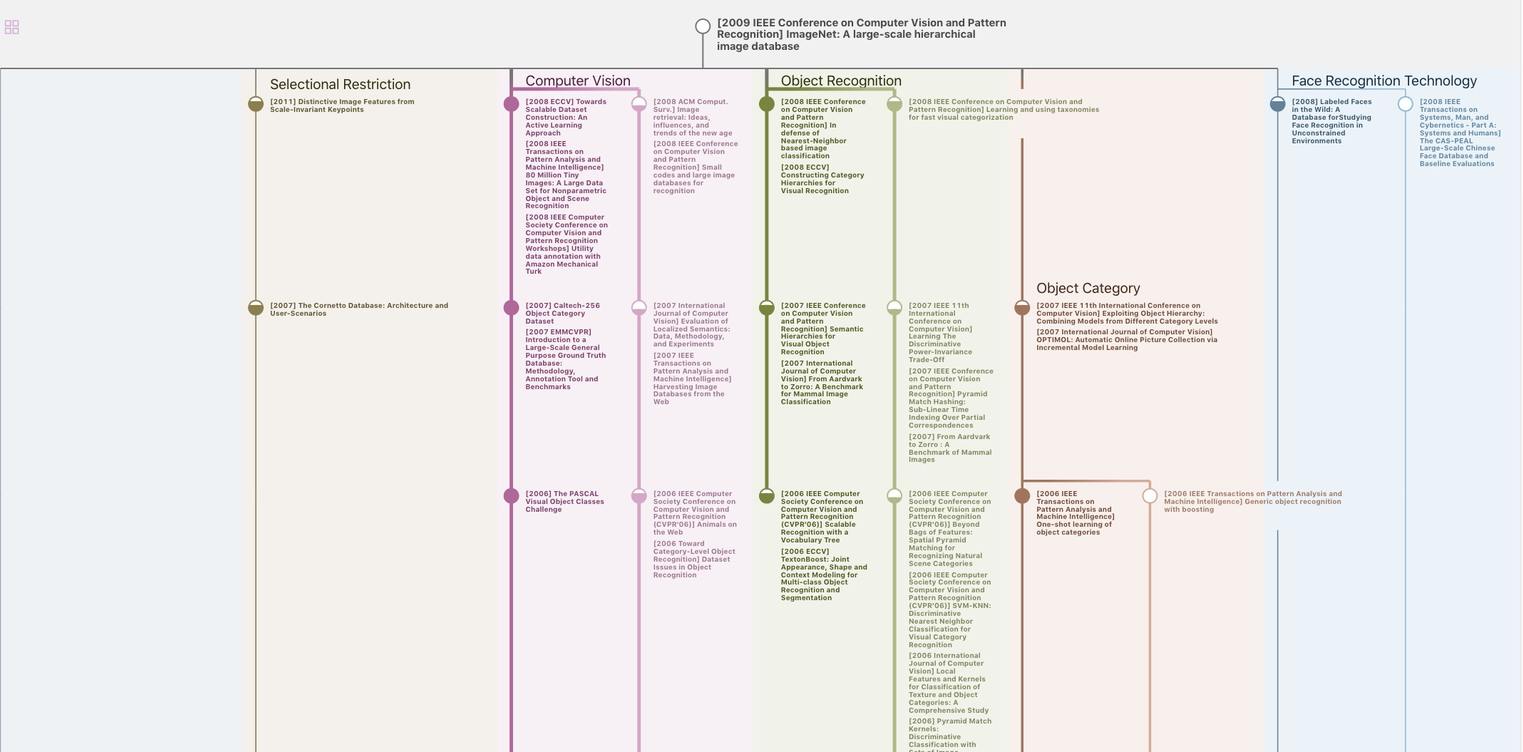
生成溯源树,研究论文发展脉络
Chat Paper
正在生成论文摘要