Emotional Climate Recognition in Interactive Conversational Speech Using Deep Learning
2022 IEEE International Conference on Digital Health (ICDH)(2022)
摘要
Emotions play a pivotal role in the individual's overall physical health. Therefore, there has been a steadily increasing interest towards emotion recognition in conversation (ERC). In this work, we propose bidirectional long short term memory (Bi-LSTM), convolutional neural network (CNN), and CNN-BiLSTM based models to predict the emotional climate established during the conversation by peers. Their speech signals across their conversation are analyzed using Mel frequency cepstral coefficients (MFCCs) that are then fed to the Bi-LSTM, CNN and CNN-BiLSTM models to predict the valence and arousal emotional climate cues. The proposed approach was tested on a publicly available dataset, namely K-EmoCon, that includes emotion labeling and peers' speech signals, during their conversation. The obtained results show that Bi-LSTM, CNN and CNN-BiLSTM models achieved a classification accuracy (arousal/valence) of 67.5%/57.7%, 73.3%/66.9%, and 75.1%/68.3%, respectively. These encouraging results show that a combination of deep learning schemes could increase the classification accuracy and provide efficient emotional climate recognition in naturalistic conversation environments.
更多查看译文
关键词
Emotion recognition in conversations,emotional climate,deep learning,MFCC,CNN,Bi-LSTM,CNN-BiLSTM,conversational speech signals
AI 理解论文
溯源树
样例
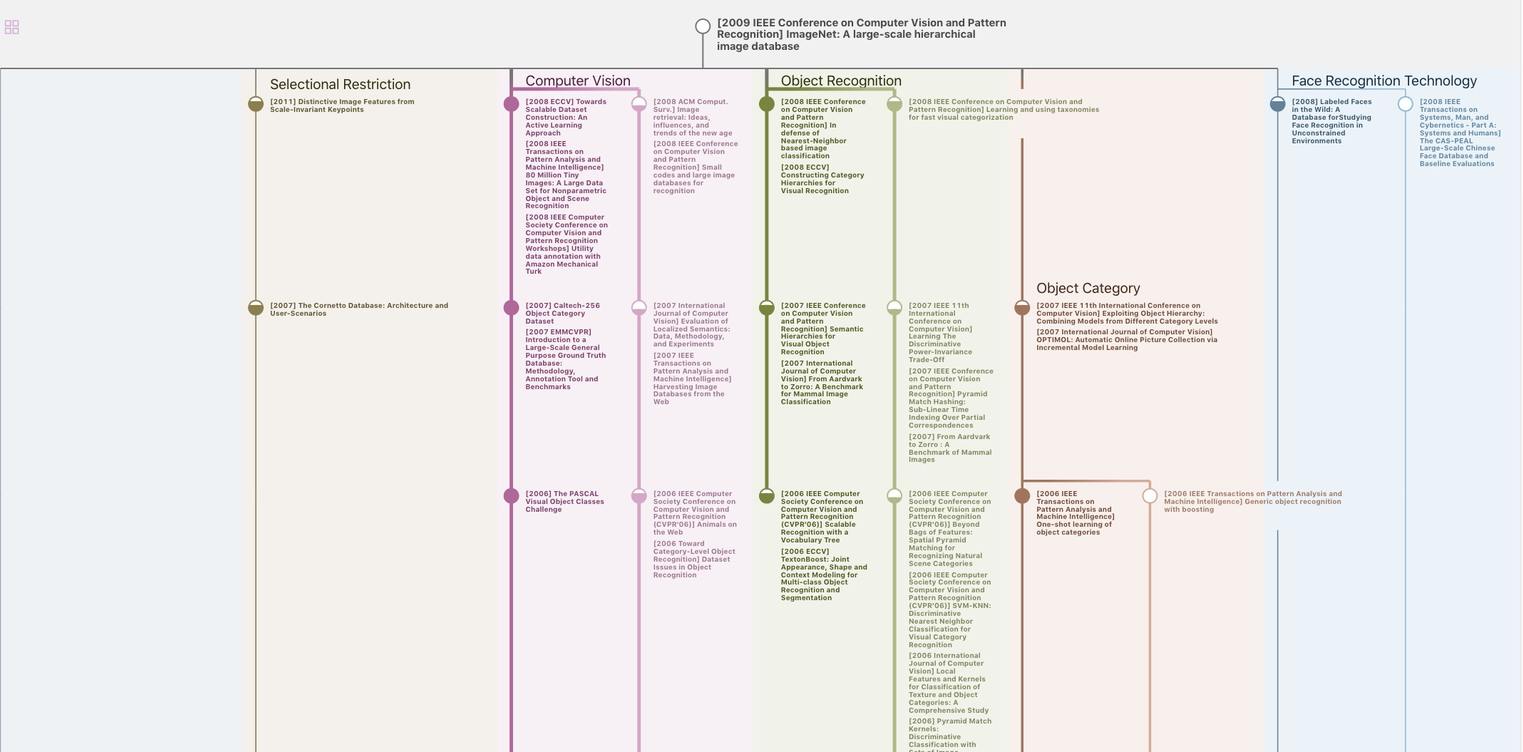
生成溯源树,研究论文发展脉络
Chat Paper
正在生成论文摘要