Phenotyping in clinical text with unsupervised numerical reasoning for patient stratification.
Experimental biology and medicine (Maywood, N.J.)(2022)
摘要
Phenotypic information of patients, as expressed in clinical text, is important in many clinical applications such as identifying patients at risk of hard-to-diagnose conditions. Extracting and inferring some phenotypes from clinical text requires numerical reasoning, for example, a temperature of 102°F suggests the phenotype Fever. However, while current state-of-the-art phenotyping models using natural language processing (NLP) are in general very efficient in extracting phenotypes, they struggle to extract phenotypes that require numerical reasoning. In this article, we propose a novel unsupervised method that leverages external clinical knowledge and contextualized word embeddings by ClinicalBERT for numerical reasoning in different phenotypic contexts. Experiments show that the proposed method achieves significant improvement against unsupervised baseline methods with absolute increase in generalized Recall and F1 scores of up to 79% and 71%, respectively. Also, the proposed method outperforms supervised baseline methods with absolute increase in generalized Recall and F1 scores of up to 70% and 44%, respectively. In addition, we validate the methodology on clinical use cases where the detected phenotypes significantly contribute to patient stratification systems for a set of diseases, namely, HIV and myocardial infarction (heart attack). Moreover, we find that these phenotypes from clinical text can be used to impute the missing values in structured data, which enrich and improve data quality.
更多查看译文
关键词
Numerical reasoning,contextualized word embeddings,deep learning,natural language processing,patient stratification,phenotyping,unsupervised learning
AI 理解论文
溯源树
样例
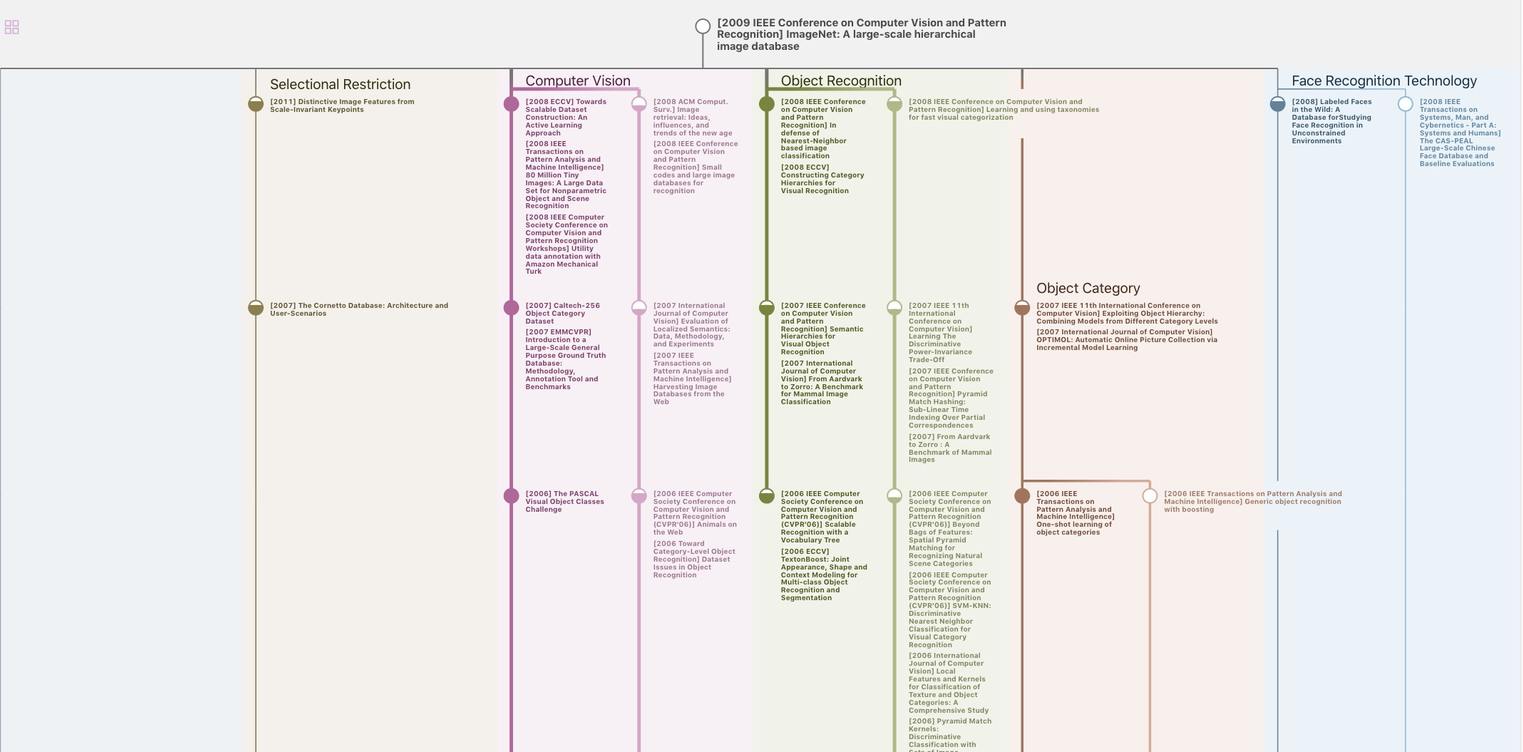
生成溯源树,研究论文发展脉络
Chat Paper
正在生成论文摘要