Federated Learning With Privacy-Preserving Ensemble Attention Distillation
IEEE transactions on medical imaging(2023)
摘要
Federated Learning (FL) is a machine learning paradigm where many local nodes collaboratively train a central model while keeping the training data decentralized. This is particularly relevant for clinical applications since patient data are usually not allowed to be transferred out of medical facilities, leading to the need for FL. Existing FL methods typically share model parameters or employ co-distillation to address the issue of unbalanced data distribution. However, they also require numerous rounds of synchronized communication and, more importantly, suffer from a privacy leakage risk. We propose a privacy-preserving FL framework leveraging unlabeled public data for one-way offline knowledge distillation in this work. The central model is learned from local knowledge via ensemble attention distillation. Our technique uses decentralized and heterogeneous local data like existing FL approaches, but more importantly, it significantly reduces the risk of privacy leakage. We demonstrate that our method achieves very competitive performance with more robust privacy preservation based on extensive experiments on image classification, segmentation, and reconstruction tasks.
更多查看译文
关键词
Privacy,federated learning,distillation
AI 理解论文
溯源树
样例
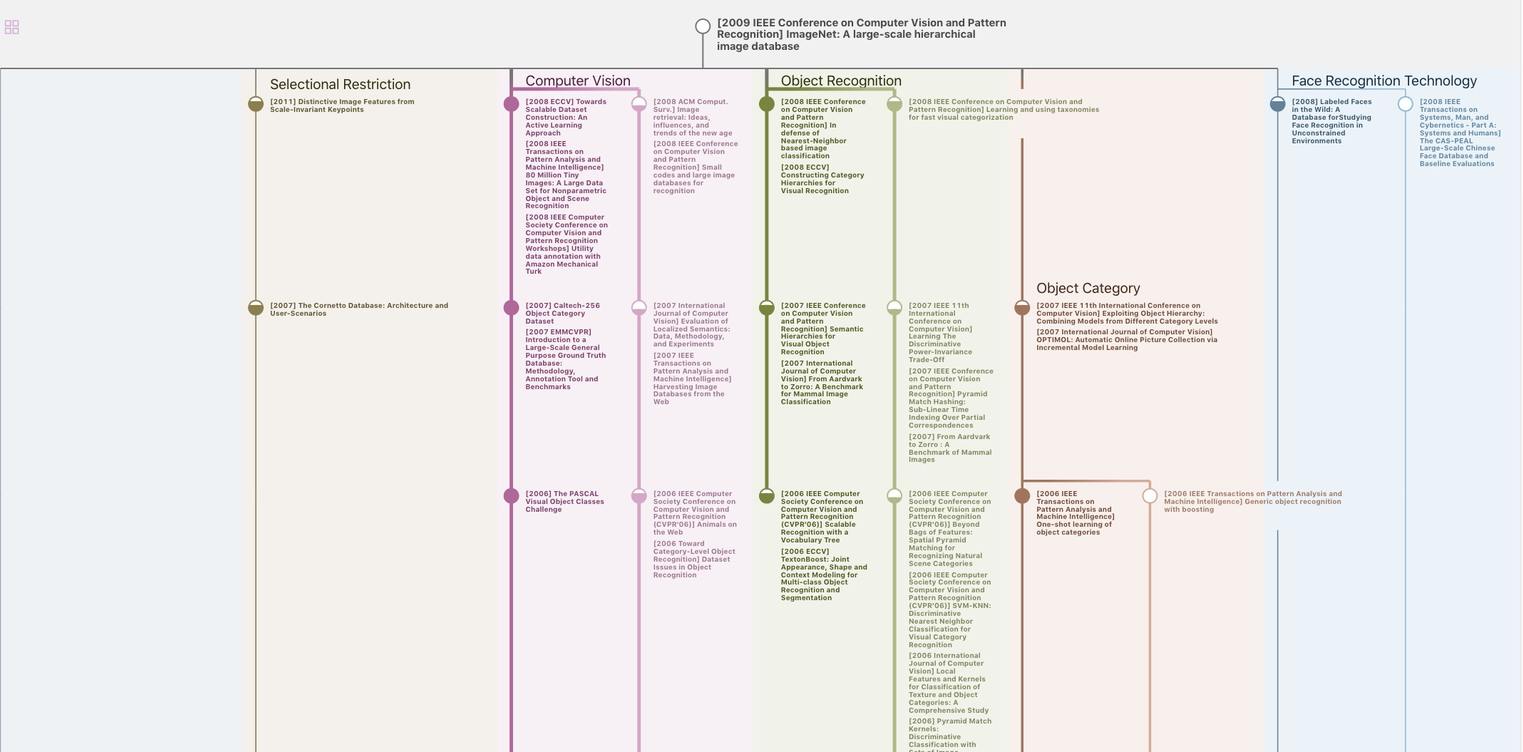
生成溯源树,研究论文发展脉络
Chat Paper
正在生成论文摘要