Disentangling Causal Effects from Sets of Interventions in the Presence of Unobserved Confounders
NeurIPS 2022(2022)
摘要
The ability to answer causal questions is crucial in many domains, as causal inference allows one to understand the impact of interventions. In many applications, only a single intervention is possible at a given time. However, in some important areas, multiple interventions are concurrently applied. Disentangling the effects of single interventions from jointly applied interventions is a challenging task -- especially as simultaneously applied interventions can interact. This problem is made harder still by unobserved confounders, which influence both treatments and outcome. We address this challenge by aiming to learn the effect of a single-intervention from both observational data and sets of interventions. We prove that this is not generally possible, but provide identification proofs demonstrating that it can be achieved under non-linear continuous structural causal models with additive, multivariate Gaussian noise -- even when unobserved confounders are present. Importantly, we show how to incorporate observed covariates and learn heterogeneous treatment effects. Based on the identifiability proofs, we provide an algorithm that learns the causal model parameters by pooling data from different regimes and jointly maximizing the combined likelihood. The effectiveness of our method is empirically demonstrated on both synthetic and real-world data.
更多查看译文
关键词
Causal Inference,Additive Noise Models,Joint Interventions
AI 理解论文
溯源树
样例
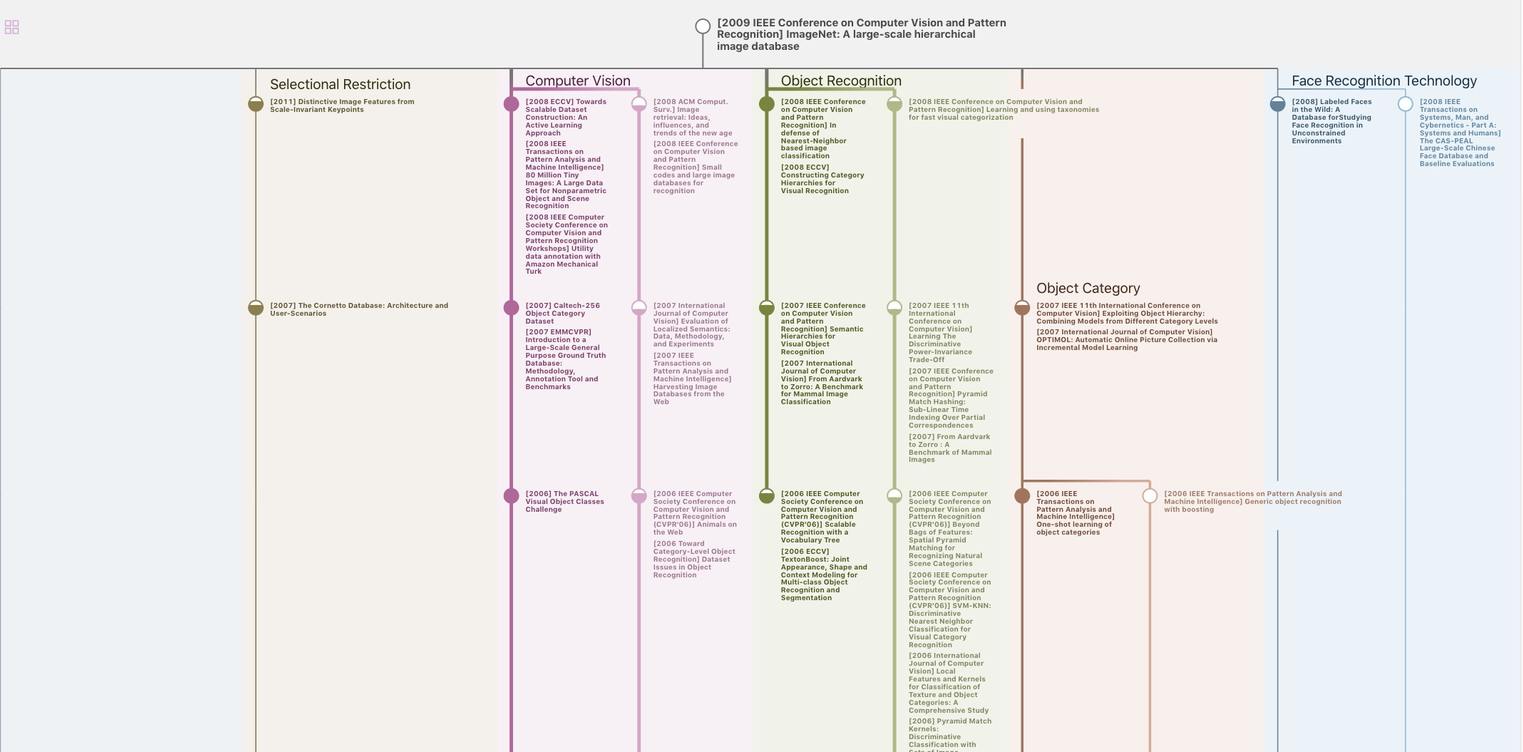
生成溯源树,研究论文发展脉络
Chat Paper
正在生成论文摘要