Spectral Sparsification for Communication-Efficient Collaborative Rotation and Translation Estimation
IEEE TRANSACTIONS ON ROBOTICS(2024)
摘要
We propose fast and communication-efficient optimization algorithms for multirobot rotation averaging and translation estimation problems that arise from collaborative simultaneous localization and mapping (SLAM), structure-from-motion (SfM), and camera network localization applications. Our methods are based on theoretical relations between the Hessians of the underlying Riemannian optimization problems and the Laplacians of suitably weighted graphs. We leverage these results to design a collaborative solver in which robots coordinate with a central server to perform approximate second-order optimization, by solving a Laplacian system at each iteration. Crucially, our algorithms permit robots to employ spectral sparsification to sparsify intermediate dense matrices before communication, and hence provide a mechanism to tradeoff accuracy with communication efficiency with provable guarantees. We perform rigorous theoretical analysis of our methods and prove that they enjoy (local) linear rate of convergence. Furthermore, we show that our methods can be combined with graduated nonconvexity to achieve outlier-robust estimation. Extensive experiments on real-world SLAM and SfM scenarios demonstrate the superior convergence rate and communication efficiency of our methods.
更多查看译文
关键词
Multirobot systems,optimization,simultaneous localization and mapping (SLAM)
AI 理解论文
溯源树
样例
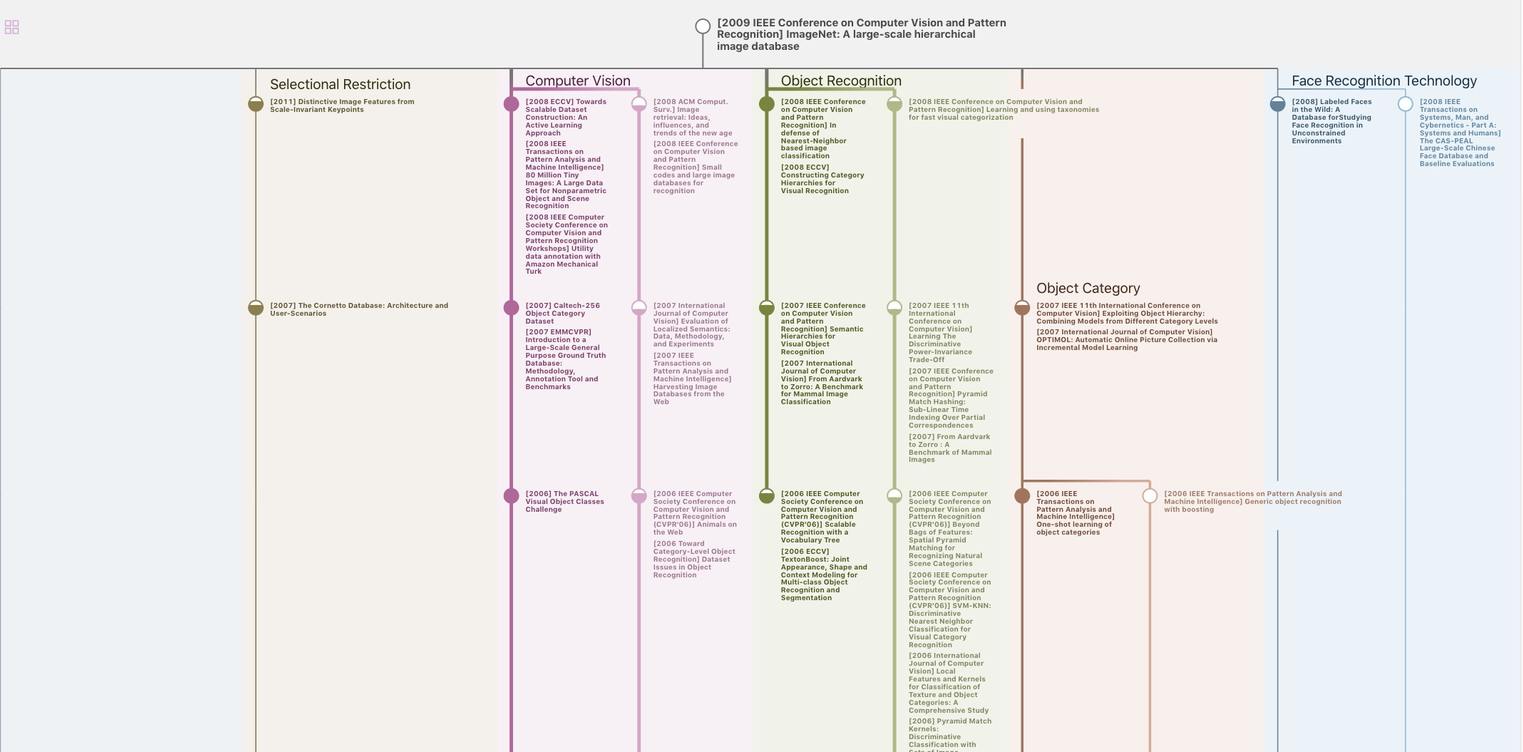
生成溯源树,研究论文发展脉络
Chat Paper
正在生成论文摘要