Uncertainty-aware pseudo-label and consistency for semi-supervised medical image segmentation
Biomedical Signal Processing and Control(2023)
摘要
In medical image segmentation tasks, fully-supervised learning has been a huge success by using abundant labeled data. However, it is time-consuming and expensive for technicians to label medical images. In this paper, we propose a novel framework for semi-supervised medical image segmentation, named Uncertainty-aware Pseudo-label and Consistency. Our framework is made up of the student–teacher models. The supervised loss on labeled data and the consistency loss on both labeled and unlabeled data are weighted and combined to optimize the models. Our method combines the recent state-of-the-art semi-supervised methods, which are consistency regularization and pseudo-labeling. More importantly, we calculate the Kullback–Leibler variance between the student model’s prediction and the teacher model’s prediction as uncertainty estimation, and directly use the uncertainty to rectify the learning of noisy pseudo-labels, instead of setting a fixed threshold to filter the pseudo-labels. Experiments on the Left Atrium dataset show that our method can efficiently utilize unlabeled data to achieve high performance and outperform other state-of-the-art semi-supervised methods. In addition, we have also analyzed its difference from conventional methods of consistency regularization and pseudo-labeling in semi-supervised medical image segmentation. Code is available in https://github.com/GXU-GMU-MICCAI/UPC-Pytorch.
更多查看译文
关键词
Semi-supervised learning,Medical image segmentation,Pseudo-labeling,Consistency regularization,Uncertainty estimation
AI 理解论文
溯源树
样例
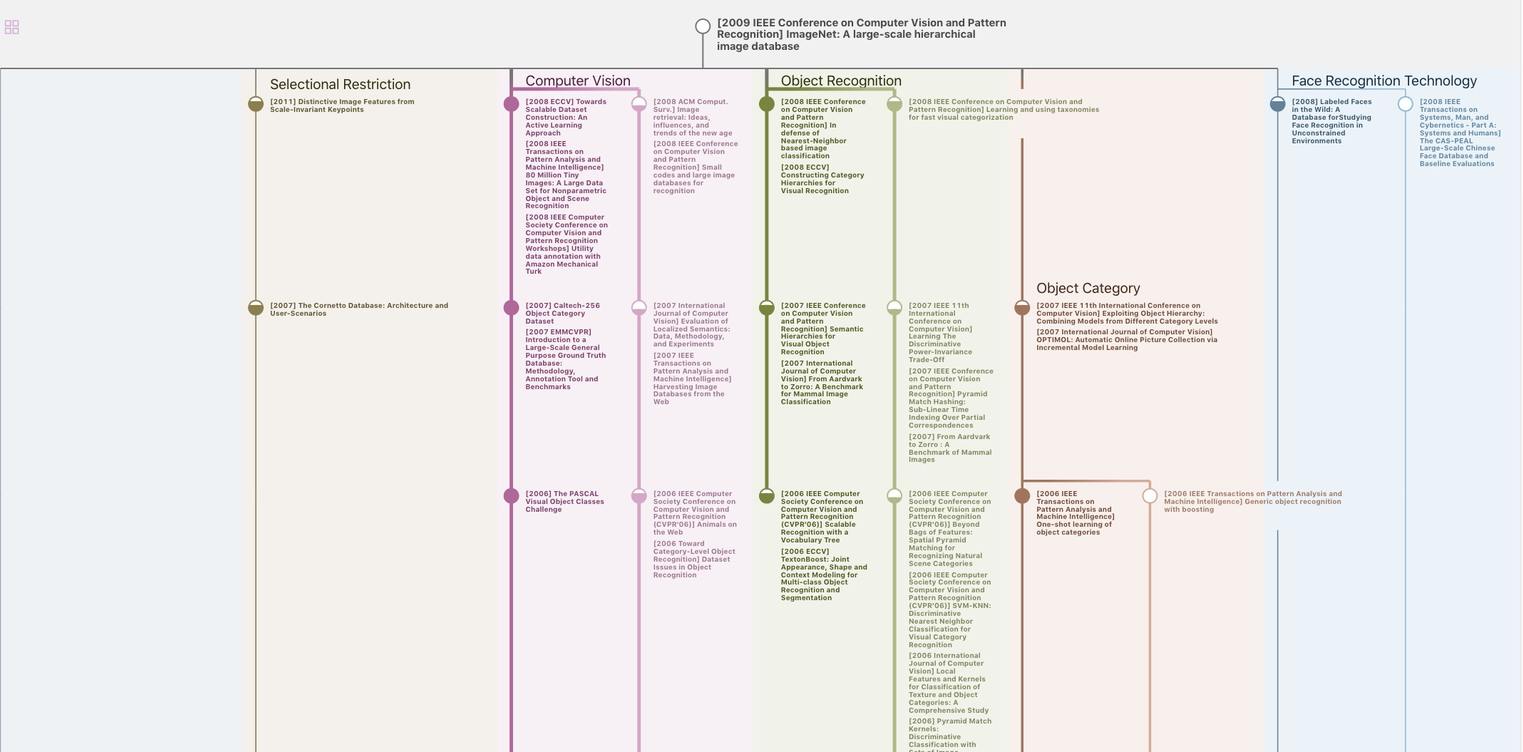
生成溯源树,研究论文发展脉络
Chat Paper
正在生成论文摘要