Amplitude-Time Dual-View Fused EEG Temporal Feature Learning for Automatic Sleep Staging
IEEE TRANSACTIONS ON NEURAL NETWORKS AND LEARNING SYSTEMS(2022)
摘要
Electroencephalogram (EEG) plays an important role in studying brain function and human cognitive performance, and the recognition of EEG signals is vital to develop an automatic sleep staging system. However, due to the complex nonstationary characteristics and the individual difference between subjects, how to obtain the effective signal features of the EEG for practical application is still a challenging task. In this article, we investigate the EEG feature learning problem and propose a novel temporal feature learning method based on amplitude-time dual-view fusion for automatic sleep staging. First, we explore the feature extraction ability of convolutional neural networks for the EEG signal from the perspective of interpretability and construct two new representation signals for the raw EEG from the views of amplitude and time. Then, we extract the amplitude-time signal features that reflect the transformation between different sleep stages from the obtained representation signals by using conventional 1-D CNNs. Furthermore, a hybrid dilation convolution module is used to learn the long-term temporal dependency features of EEG signals, which can overcome the shortcoming that the small-scale convolution kernel can only learn the local signal variation information. Finally, we conduct attention-based feature fusion for the learned dual-view signal features to further improve sleep staging performance. To evaluate the performance of the proposed method, we test 30-s-epoch EEG signal samples for healthy subjects and subjects with mild sleep disorders. The experimental results from the most commonly used datasets show that the proposed method has better sleep staging performance and has the potential for the development and application of an EEG-based automatic sleep staging system.
更多查看译文
关键词
Sleep,Electroencephalography,Feature extraction,Convolution,Representation learning,Learning systems,Kernel,Amplitude-time dual-view fusion,attention,automatic sleep staging,electroencephalogram (EEG),feature learning,hybrid dilation convolution
AI 理解论文
溯源树
样例
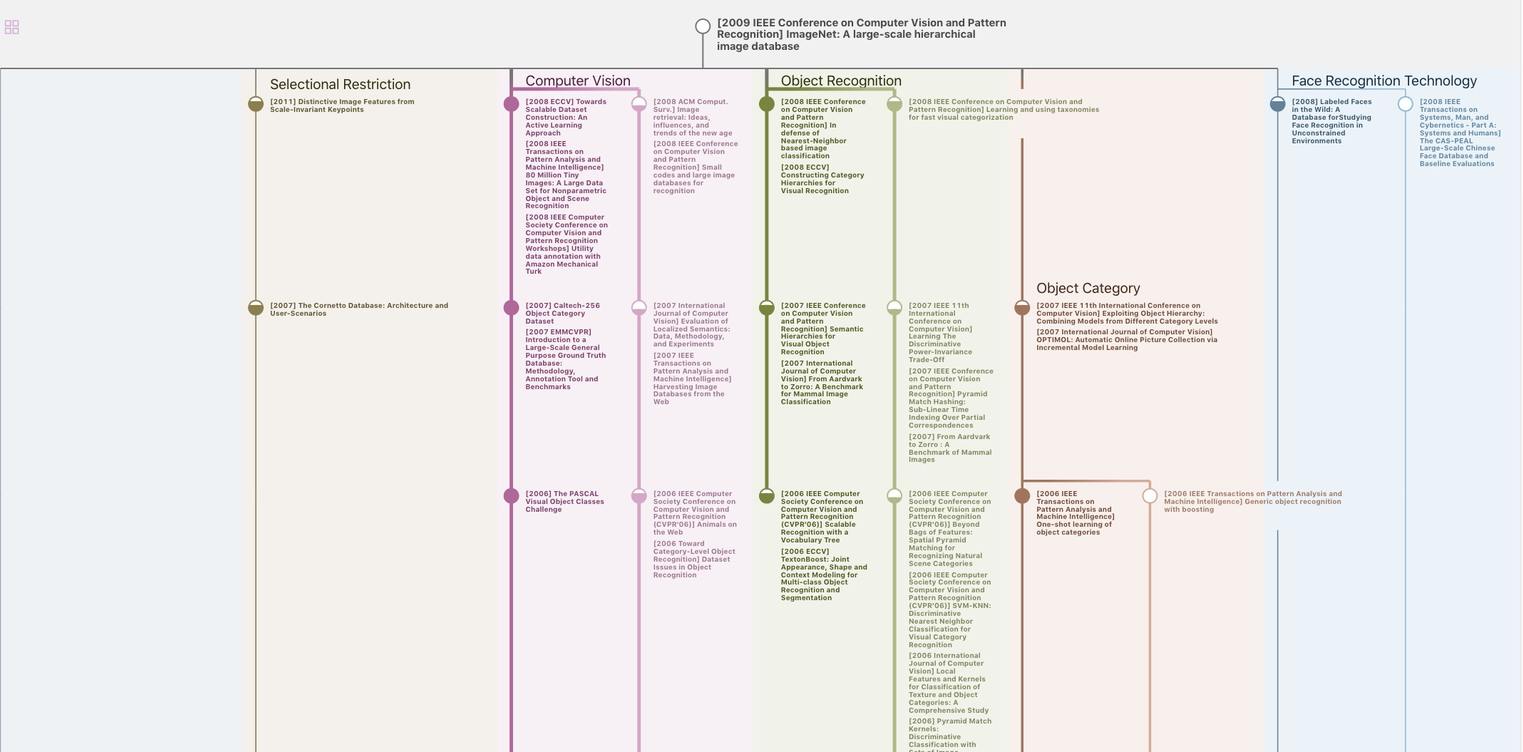
生成溯源树,研究论文发展脉络
Chat Paper
正在生成论文摘要