Multi-heterogeneous sensor data fusion method via convolutional neural network for fault diagnosis of wheeled mobile robot
Applied Soft Computing(2022)
摘要
Wheeled mobile robots usually operate in a variety of human–robot coexisting environments. It is vital to improve their safety and reliability by investigating fault diagnosis methods. Multi-sensor data can provide a comprehensive description of a robot’s running state. However, wheeled mobile robots are generally equipped with multi-heterogeneous sensors, and extracting high-level fault features from multi-heterogeneous sensor data is difficult. This study proposes a novel channel-wise convolutional neural network with feature augmentation (CWCNN-FA) for the fault diagnosis of wheeled mobile robots. First, the proposed CWCNN-FA directly uses multi-heterogeneous sensor data as the input. Then, the channel-wise convolution mechanism is introduced to independently extract the heterogeneous fault features from the multi-channel input, which prevents these features from being mixed by the conventional convolution mechanism. Moreover, a feature augmentation layer was developed to highlight important features by adaptively weighting the multi-heterogeneous feature maps. Finally, all the feature maps were fed into the fully connected layer to perform data fusion and fault classification. Comparison experiments were conducted between CWCNN-FA and several commonly used fault diagnosis methods to verify the validity of the proposed method. The experimental results revealed that the proposed CWCNN-FA exhibits superior performance in terms of fault diagnosis accuracy and robustness.
更多查看译文
关键词
Intelligent fault diagnosis,Convolutional neural network,Multi-heterogeneous sensor data,Data fusion,Wheeled mobile robot
AI 理解论文
溯源树
样例
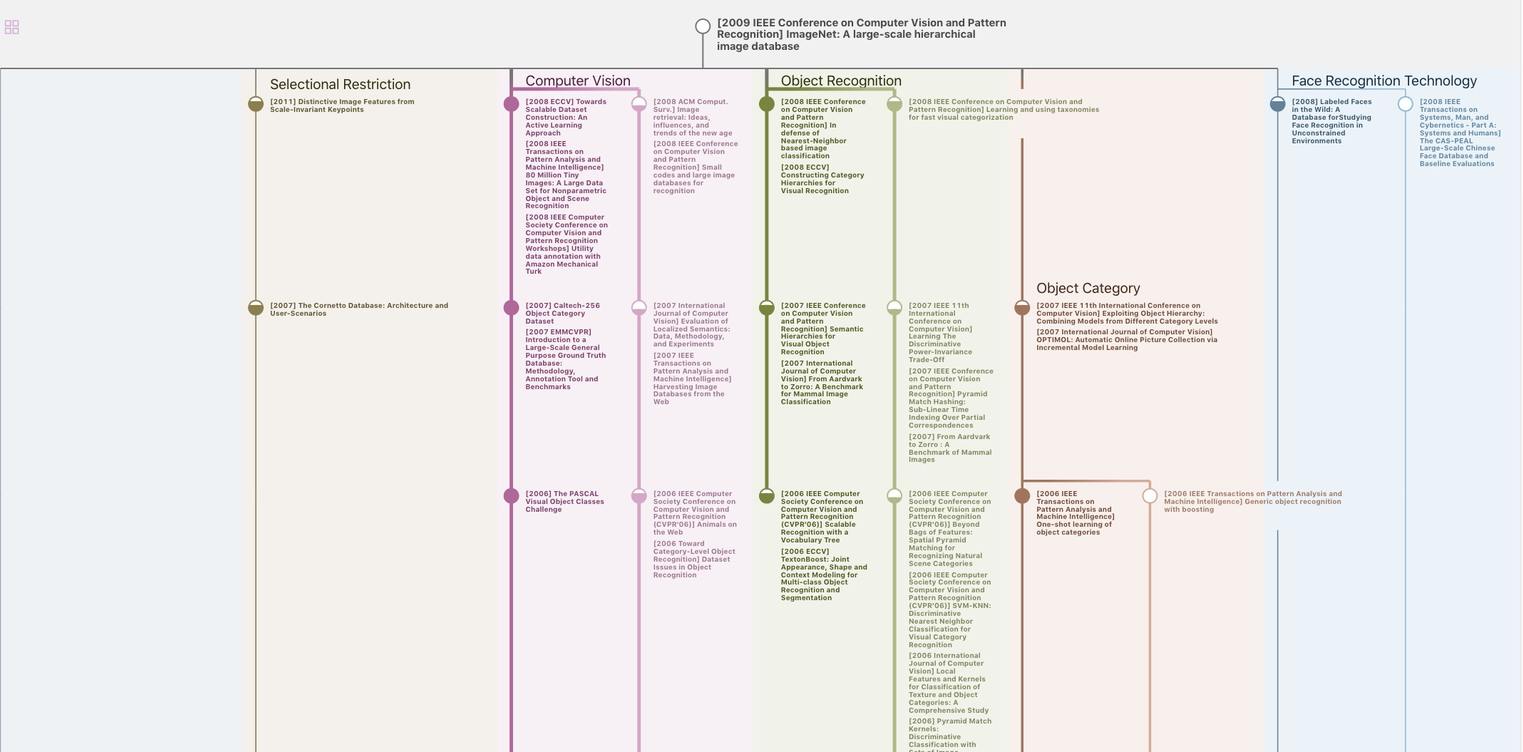
生成溯源树,研究论文发展脉络
Chat Paper
正在生成论文摘要