Clustering ensemble based on approximate accuracy of the equivalence granularity
Applied Soft Computing(2022)
摘要
Clustering ensemble deals with the inconsistent parts of base clusterings while maintaining consistency in the clustering results. The cluster core samples generally have more consistent neighbor relationship in base clusterings than the cluster edge samples, and these two types of samples have different contributions to the determination of the underlying data structure. In addition, in clustering ensemble, some samples that are equivalent in base clusterings can be merged into equivalence granularity to reduce the size of input data. Based on these ideas and combined with rough set theory, we propose a novel clustering ensemble algorithm based on the approximate accuracy of equivalence granularity. We initially define the clustering equivalence relation, extract the consistent results of base clusterings based on this relation, and upgrade the clustering ensemble from the sample level to the equivalence granularity level. Afterward, we use the approximate accuracy of the rough set to quantify the contribution of equivalence granularity in discovering the underlying data structure, divide the equivalence granularities into core and edge equivalence granularities, and implement different clustering processing strategies. The visual experiments on four synthetic data sets and comparison experiments with 14 state-of-the-art clustering ensemble algorithms on 21 data sets respectively prove the rationality and excellent performance of our proposed algorithm.
更多查看译文
关键词
Clustering ensemble,Rough set theory,Equivalence granularity,Approximate accuracy
AI 理解论文
溯源树
样例
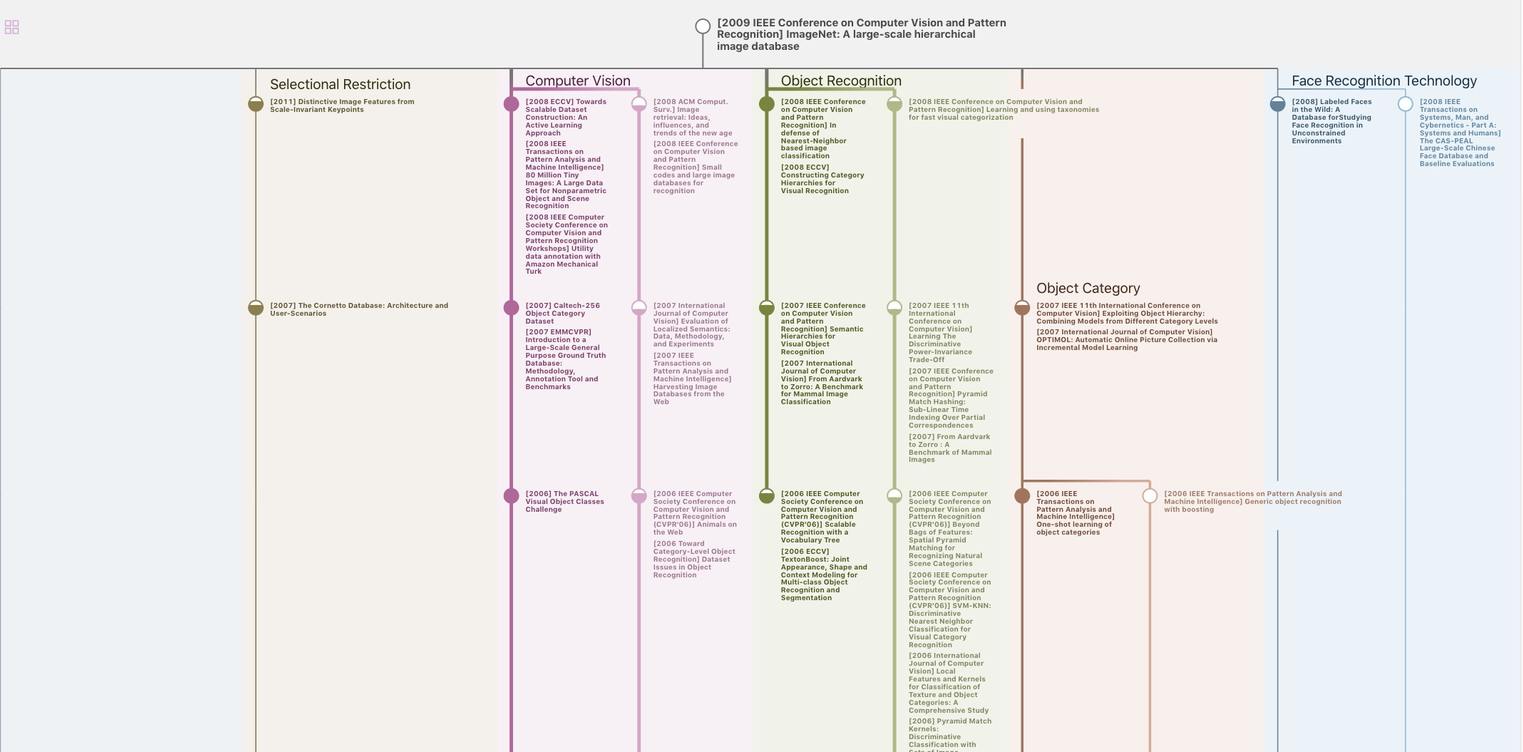
生成溯源树,研究论文发展脉络
Chat Paper
正在生成论文摘要