Deep Neural Networks for Rapid Simulation of Planar Microwave Circuits Based on Their Layouts
IEEE Transactions on Microwave Theory and Techniques(2022)
摘要
This article demonstrates a deep learning (DL)-based methodology for the rapid simulation of planar microwave circuits based on their layouts. We train convolutional neural networks (CNNs) to compute the scattering parameters of general, two-port circuits consisting of a metallization layer printed on a grounded dielectric substrate, by processing the metallization pattern along with the thickness and dielectric permittivity of the substrate. This approach harnesses the efficiency of CNNs with pattern recognition tasks and extends previous efforts to employ neural networks for the simulation of parameterized circuit geometries. Furthermore, we integrate this CNN in a hybrid network with a long-short term memory (LSTM) module that uses coarse mesh finite-difference time-domain (FDTD) simulation data as an additional input. We show that this hybrid network is computationally efficient and generalizable, accurately modeling geometries well beyond those that the network has previously seen.
更多查看译文
关键词
Artificial neural networks,finite difference methods,microwave circuits
AI 理解论文
溯源树
样例
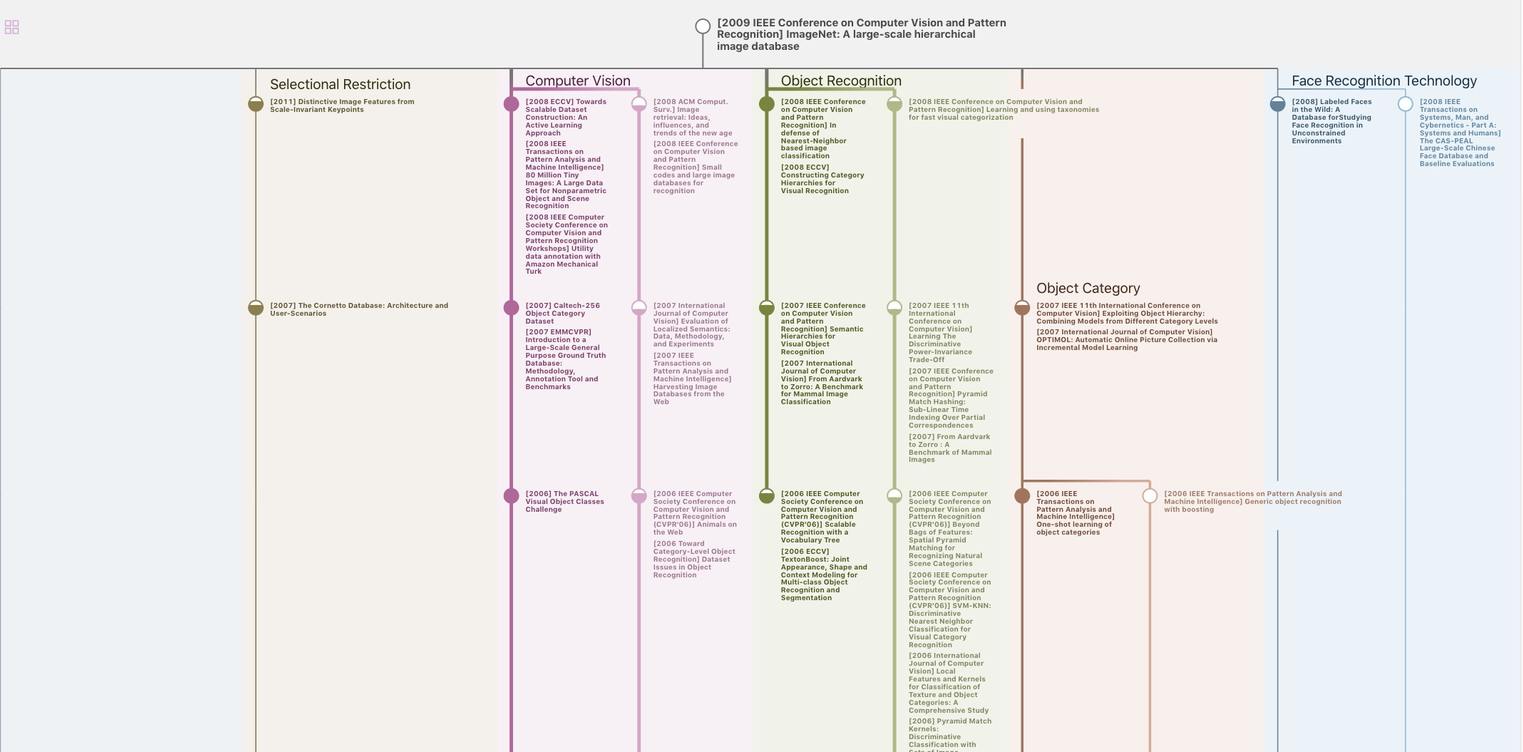
生成溯源树,研究论文发展脉络
Chat Paper
正在生成论文摘要