Safety-Aware Pursuit-Evasion Games in Unknown Environments Using Gaussian Processes and Finite-Time Convergent Reinforcement Learning
IEEE TRANSACTIONS ON NEURAL NETWORKS AND LEARNING SYSTEMS(2024)
摘要
This article develops a safe pursuit-evasion game for enabling finite-time capture, optimal performance as well as adaptation to an unknown cluttered environment. The pursuit-evasion game is formulated as a zero-sum differential game wherein the pursuer seeks to minimize its relative distance to the target while the evader attempts to maximize it. A critic-only reinforcement learning (RL)-based algorithm is then proposed for learning online and in finite time the pursuit-evasion policies and thus enabling finite-time capture of the evader. Safety is ensured by means of barrier functions associated with the obstacles, which are integrated into the running cost. Using Gaussian processes (GPs), a learning-based mechanism is devised for safely learning the unknown environment. Simulation results illustrate the efficacy of the proposed approach.
更多查看译文
关键词
Games,Safety,Gaussian processes,Target tracking,Reinforcement learning,Costs,Stability criteria,Differential games,finite-time stability,Gaussian processes (GPs),reinforcement learning (RL),safety
AI 理解论文
溯源树
样例
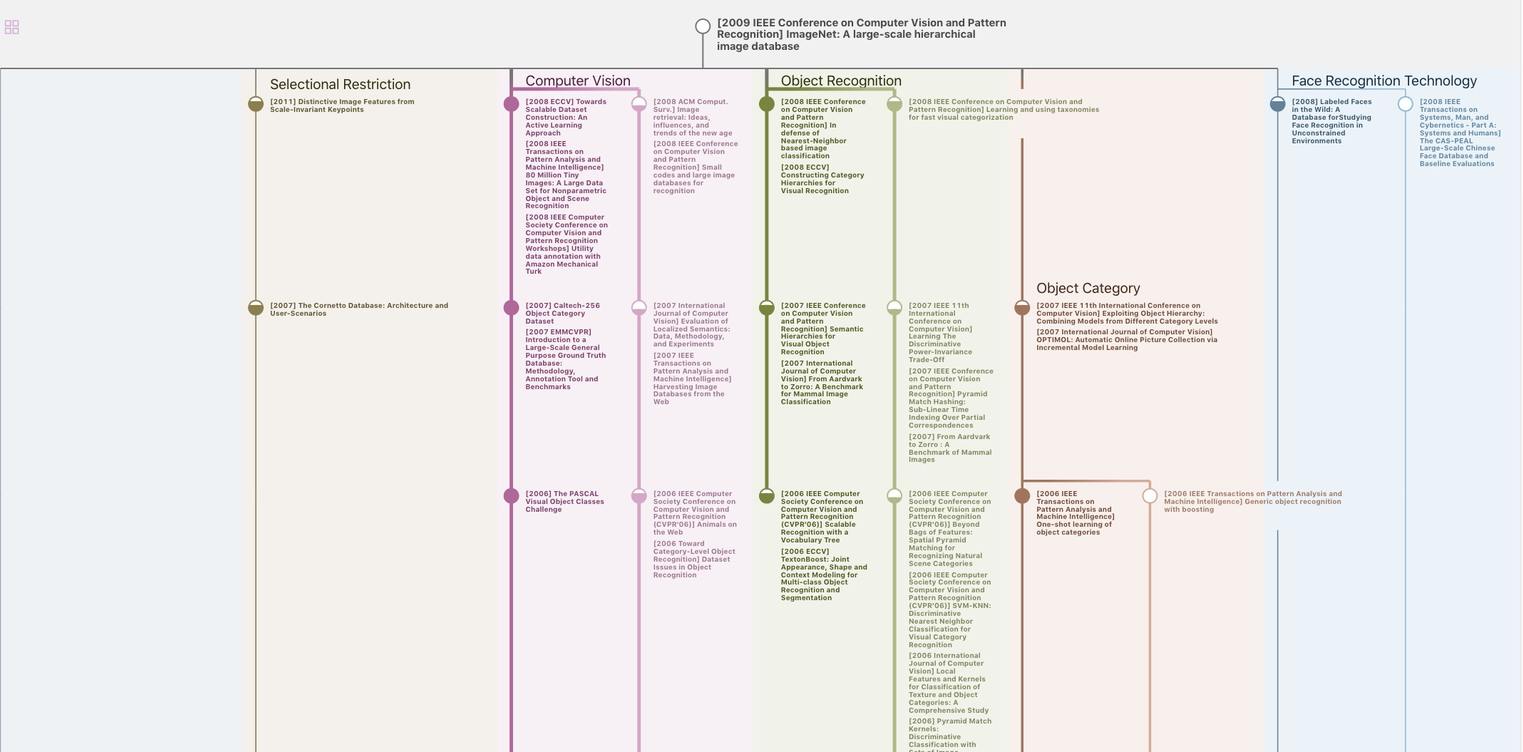
生成溯源树,研究论文发展脉络
Chat Paper
正在生成论文摘要