LieGG: Studying Learned Lie Group Generators
NeurIPS 2022(2022)
摘要
Symmetries built into a neural network have appeared to be very beneficial for a wide range of tasks as it saves the data to learn them. We depart from the position that when symmetries are not built into a model a priori, it is advantageous for robust networks to learn symmetries directly from the data to fit a task function. In this paper, we present a method to extract symmetries learned by a neural network and to evaluate the degree to which a network is invariant to them. With our method, we are able to explicitly retrieve learned invariances in a form of the generators of corresponding Lie-groups without prior knowledge of symmetries in the data. We use the proposed method to study how symmetrical properties depend on a neural network's parameterization and configuration. We found that the ability of a network to learn symmetries generalizes over a range of architectures. However, the quality of learned symmetries depends on the depth and the number of parameters.
更多查看译文
关键词
invariance,equivariance,symmetry,Lie groups,interpretability
AI 理解论文
溯源树
样例
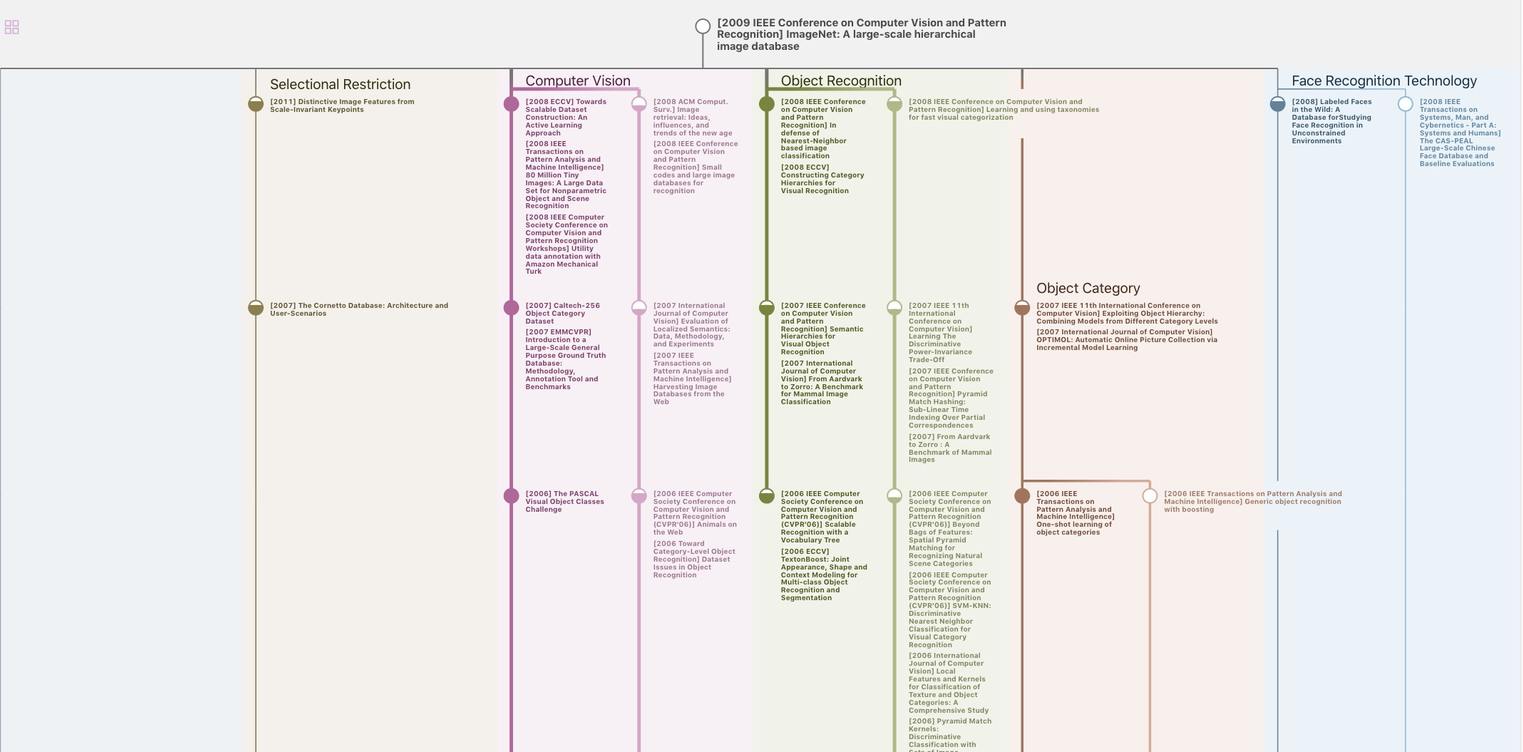
生成溯源树,研究论文发展脉络
Chat Paper
正在生成论文摘要