A Contextual Bandit Approach for Value-Oriented Prediction Interval Forecasting
IEEE TRANSACTIONS ON SMART GRID(2024)
摘要
Prediction interval (PI) is an effective tool to quantify uncertainty and usually serves as an input to downstream robust optimization. Traditional approaches focus on improving the quality of PI in the view of statistical scores and assume the improvement in quality will lead to a higher value in the power systems operation. However, such an assumption cannot always hold in practice. In this paper, we propose a value-oriented PI forecasting approach, which aims at reducing operational costs in downstream operations. For that, it is required to issue PIs with the guidance of operational costs in robust optimization, which is addressed within the contextual bandit framework here. Concretely, the agent is used to select the optimal quantile proportion, while the environment reveals the costs in operations as rewards to the agent. As such, the agent can learn the policy of quantile proportion selection for minimizing the operational cost. The numerical study regarding the day-ahead and real-time operation of a virtual power plant verifies the superiority of the proposed approach in terms of operational value. And it is especially evident in the context of extensive penetration of wind power.
更多查看译文
关键词
Wind forecasting,Forecasting,Costs,Wind power generation,Optimization,Decision making,Real-time systems,Prediction interval,forecast value,decision-making,uncertainty
AI 理解论文
溯源树
样例
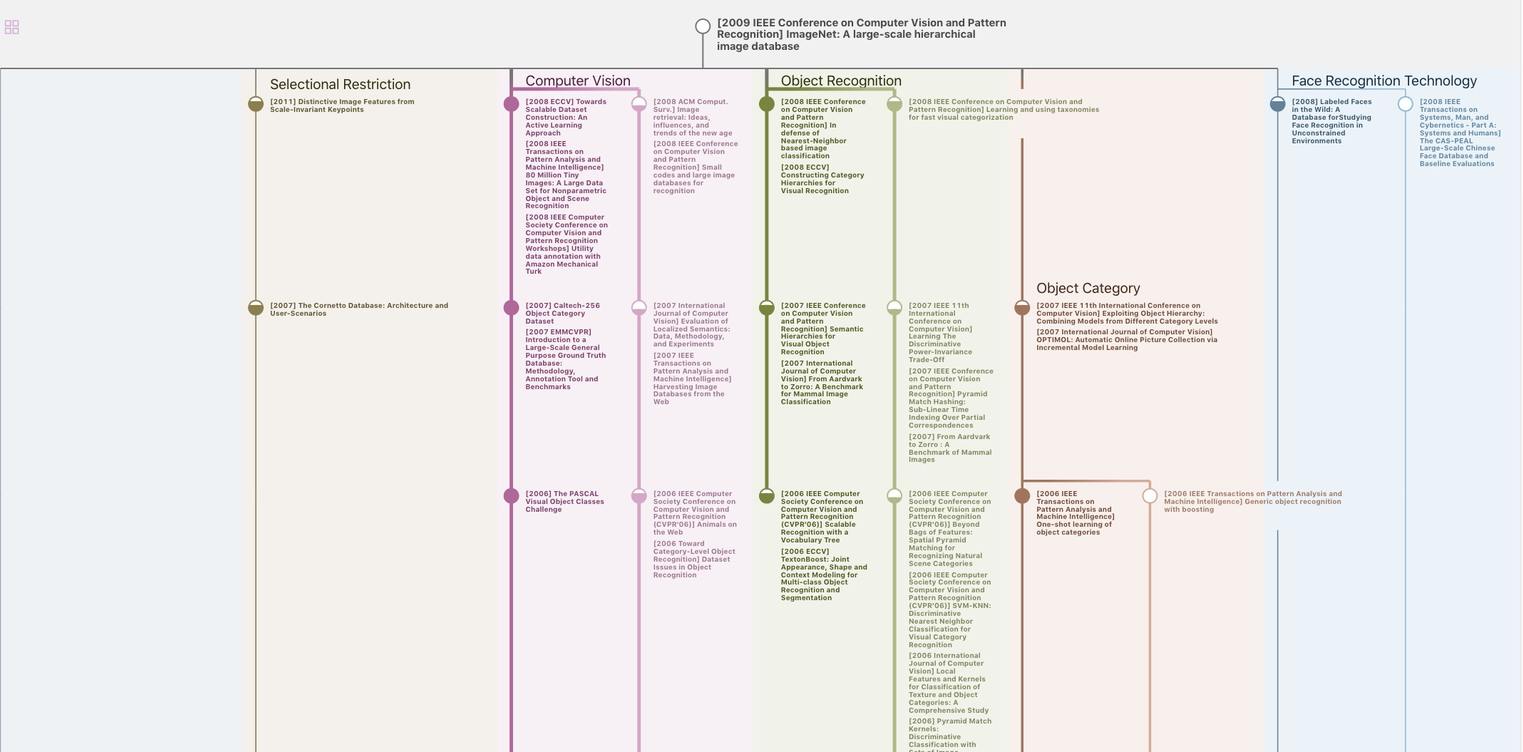
生成溯源树,研究论文发展脉络
Chat Paper
正在生成论文摘要