Towards Light Weight Object Detection System.
CoRR(2022)
摘要
Transformers are a popular choice for classification tasks and as backbones for object detection tasks. However, their high latency brings challenges in their adaptation to lightweight object detection systems. We present an approximation of the self-attention layers used in the transformer architecture. This approximation reduces the latency of the classification system while incurring minimal loss in accuracy. We also present a method that uses a transformer encoder layer for multi-resolution feature fusion. This feature fusion improves the accuracy of the state-of-the-art lightweight object detection system without significantly increasing the number of parameters. Finally, we provide an abstraction for the transformer architecture called Generalized Transformer (gFormer) that can guide the design of novel transformer-like architectures.
更多查看译文
关键词
detection,light
AI 理解论文
溯源树
样例
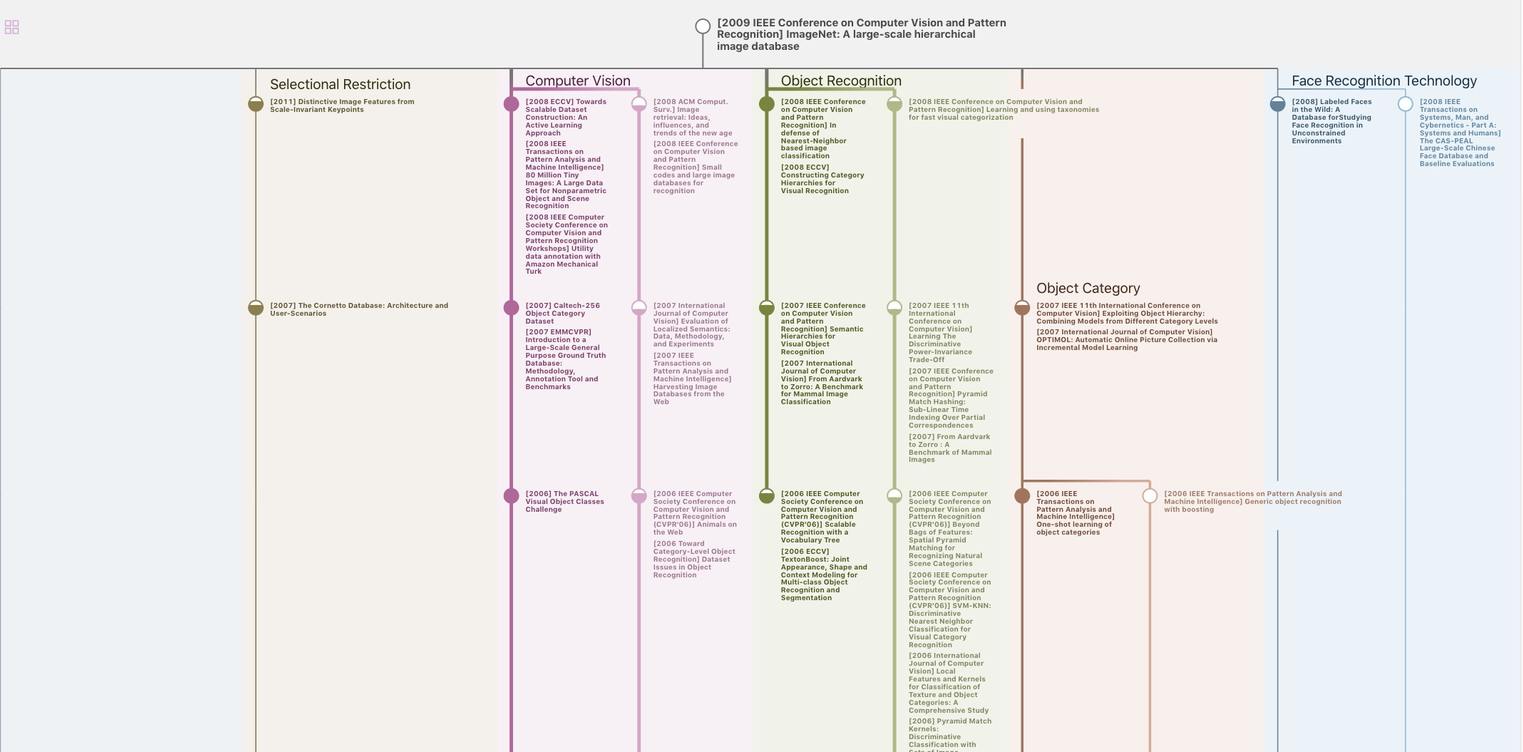
生成溯源树,研究论文发展脉络
Chat Paper
正在生成论文摘要