Symbolic aggregate approximation based data fusion model for dangerous driving behavior detection
Information Sciences(2022)
摘要
Detecting dangerous driving behavior is of great significance for reducing the occurrence of traffic accidents, and very challenging as it is affected by multiple factors. However, the existing methods are merely suitable for detecting dangerous driving behavior caused by single factor. Therefore, this paper proposes a novel Symbolic Aggregate approXimation based Data Fusion model (SAX-DF) to fuse multi-source data and applies it to accurately detect dangerous driving behavior. The proposed method considers multiple influencing factors at the same time and analyzes the related information between these factors. Further, a Positive Danger Mapping (PDM) algorithm is designed to unify the direction of multiple influencing factors. To verify the performance of SAX-DF, several experiments are carried out on traffic detector data and weather data. The experimental results illustrate that the proposed method can more effectively detect the dangerous driving behavior compared with the benchmark methods.
更多查看译文
关键词
Dangerous driving behavior detection,Symbolic aggregate approximation,Data fusion,Data mining,Urban computing
AI 理解论文
溯源树
样例
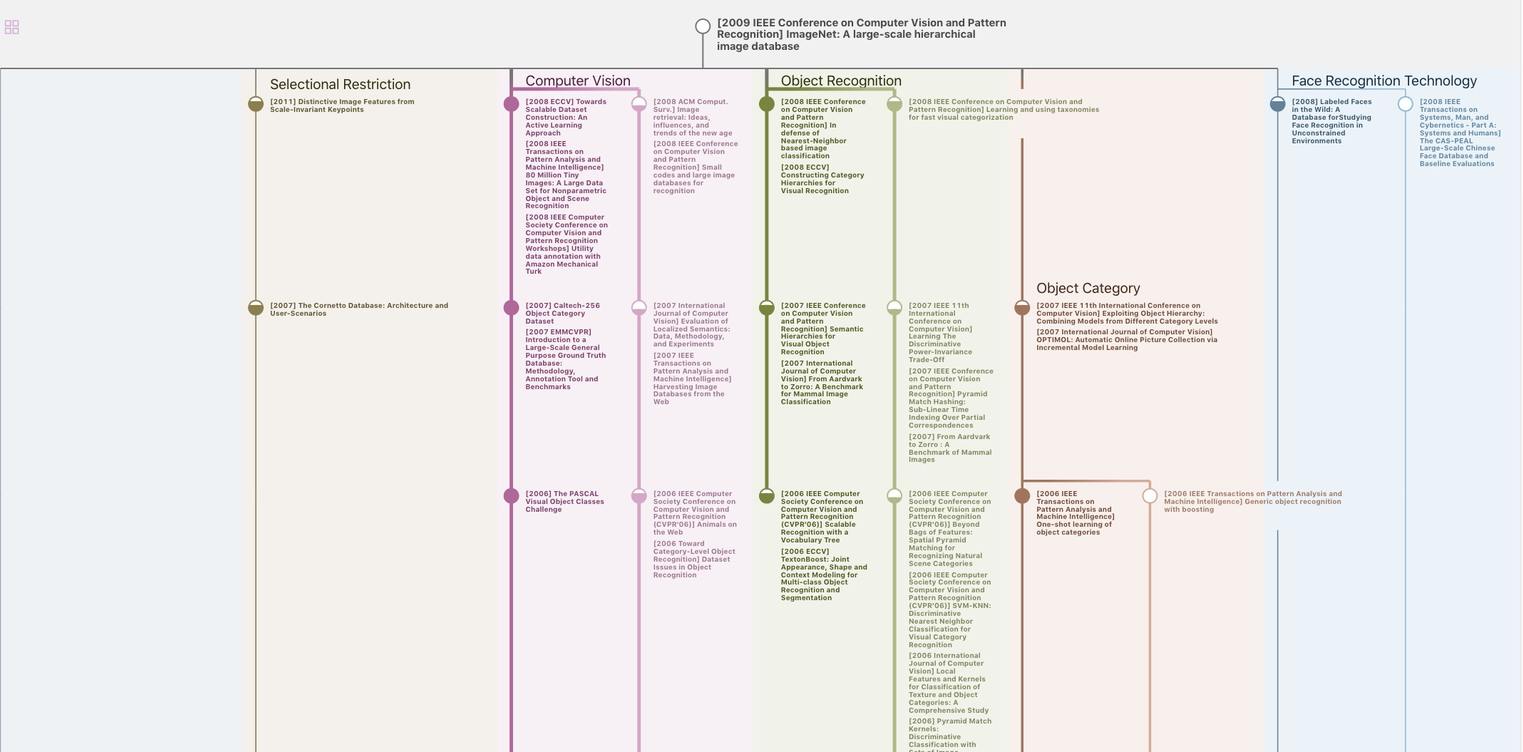
生成溯源树,研究论文发展脉络
Chat Paper
正在生成论文摘要