Approximate inferring with confidence predicting based on uncertain knowledge graph embedding
Information Sciences(2022)
摘要
Uncertainty is a natural character of knowledge, while it is still tough to be encoded into the knowledge graph embedding space that can be employed for machine learning tasks. However, the approximate inference could be performed in the embedding space, if confidence, real-value representation of the uncertainty of knowledge facts, can be learned by neural networks. To tackle this, a simple yet effective confidence predicting method is proposed, and several approximate inferring are efficiently performed based on these predictions. The model is a two-step model: knowledge elements embedding step, in which knowledge facts regarded as short sentences are fed into the natural language model to get entity and relation embedding vectors; and confidence learning step, in which the confidence distribution of knowledge facts in the knowledge graph are learned utilizing the recurrent neural network in order to carry out approximate inference. The experience demonstrates that the model achieves better results than state-of-the-art on the link prediction task over uncertain knowledge graph embedding. Uncertainty inferring grounded on predicted confidence is more accurate, feasible, and meaningful for serval knowledge inferring tasks: transitivity, composition inferring, and probabilistic soft logic inferring. Likewise, the proposed approach achieves the best tradeoff between efficiency and accuracy of uncertain knowledge graph embedding and inferring, and can be used to handle large size knowledge graphs at lower time consumption because of the simplicity.
更多查看译文
关键词
Knowledge presentation,Knowledge graphs,Uncertainty embedding,Approximate inferring,Recurrent neural network
AI 理解论文
溯源树
样例
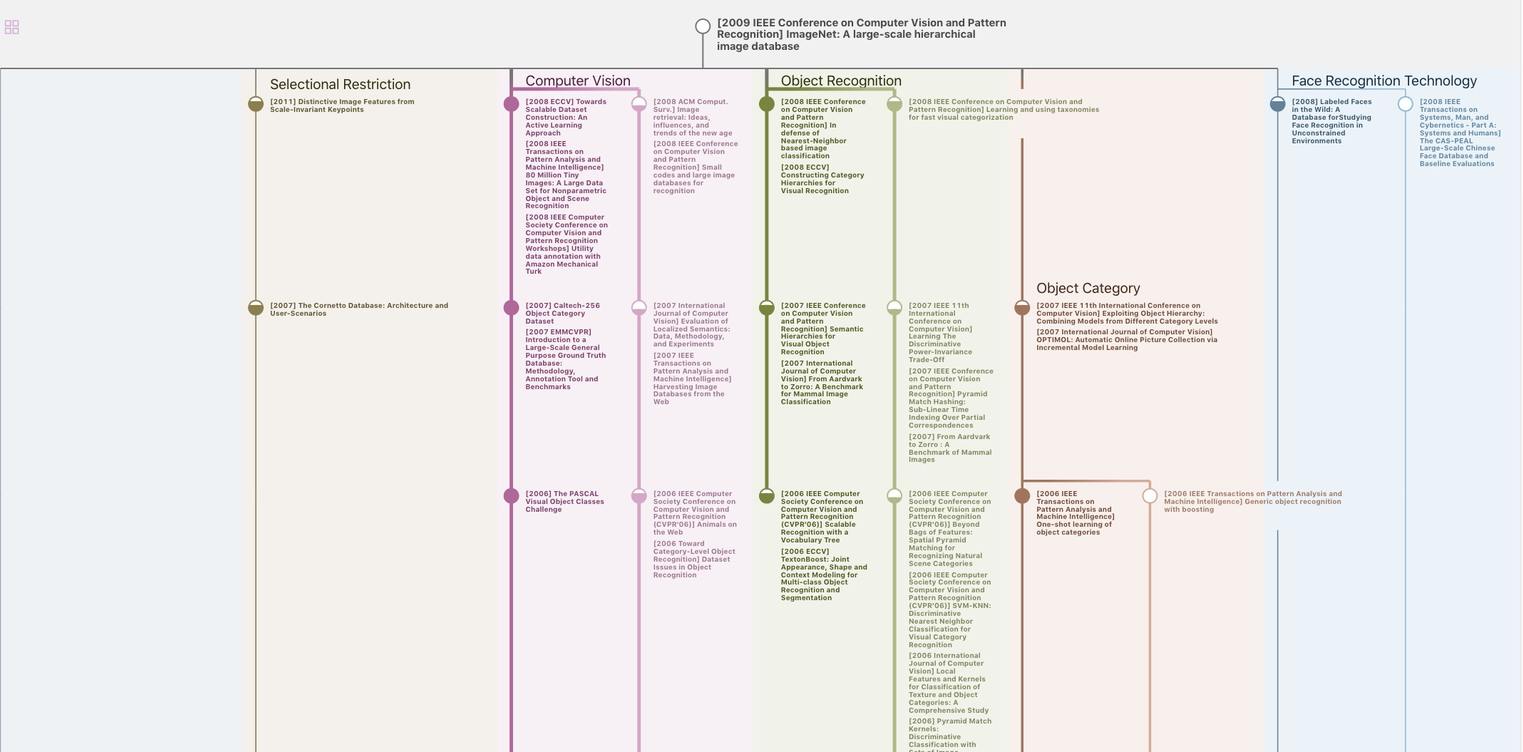
生成溯源树,研究论文发展脉络
Chat Paper
正在生成论文摘要