High-order cross-scale attention network for single image super-resolution
Digital Signal Processing(2022)
摘要
Deep convolutional neural networks (DCNN) have achieved remarkable performance for single image super-resolution (SISR). Although some recent works have introduced multi-scale representation into DCNN-based super-resolution, they neglect to explore the interdependencies across different scales. To address this issue, we propose a high-order cross-scale attention network (HOCSANet) for SISR reconstruction. Specifically, a novel high-order cross-scale attention block (HOCSABlock) is developed to integrate the multi-scale representation with attention mechanism, in which both in-scale and cross-scale feature correlations are exploited and utilized to adaptively rescale the multi-scale features. Moreover, we introduce a dual-nested residual block (DNRBlock), which combines plain residual block and wide-activated residual block and learns more informative features from the difference between them. Experimental results demonstrate the superiority of the proposed HOCSANet over several state-of-the-art SISR methods.
更多查看译文
关键词
Single image super-resolution,Convolutional neural network,Multi-scale representation,Attention mechanism,Cross-scale feature correlation
AI 理解论文
溯源树
样例
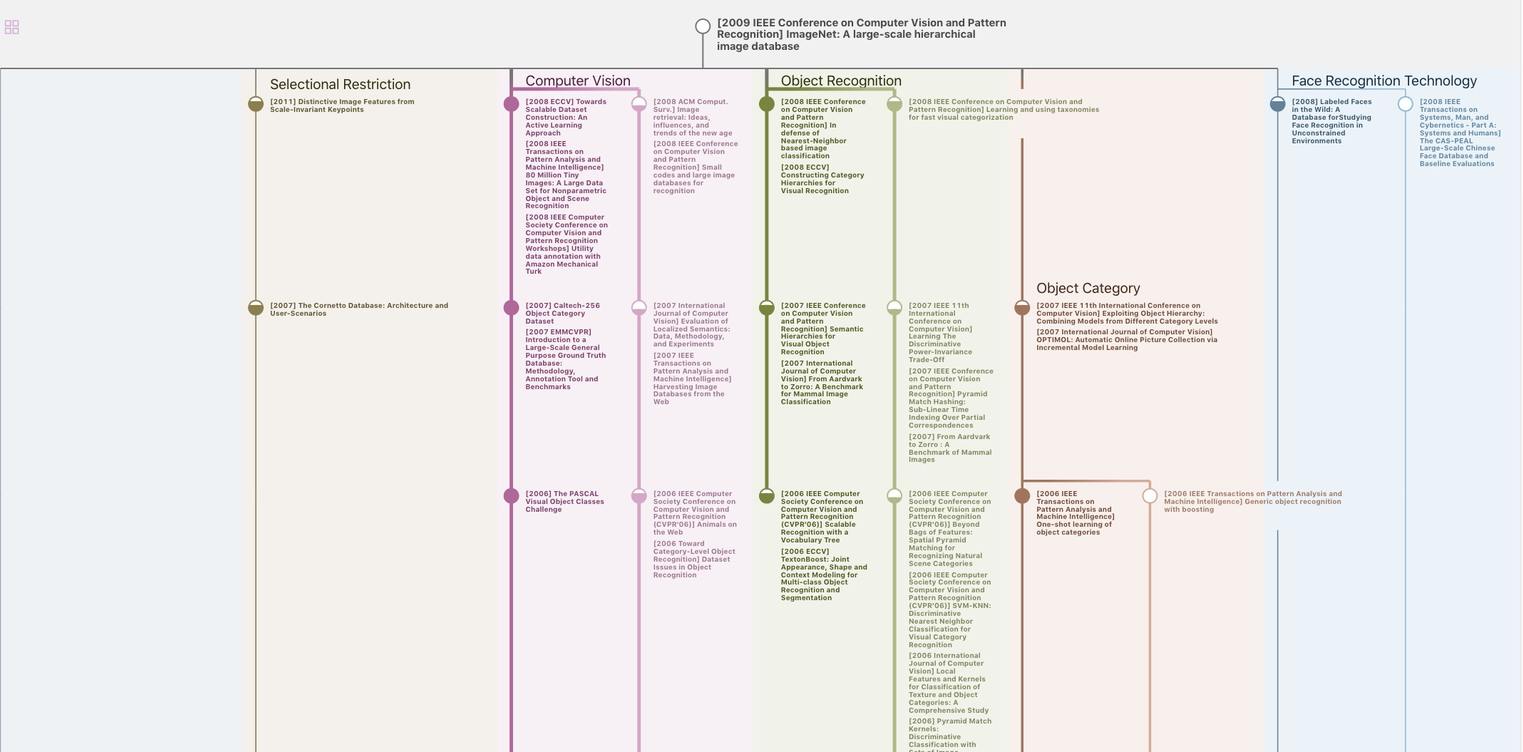
生成溯源树,研究论文发展脉络
Chat Paper
正在生成论文摘要