Discovering epistasis interactions in Alzheimer's disease using deep learning model
Gene Reports(2022)
摘要
Alzheimer's disease (AD) is the most common form of dementia. Single Nucleotide Polymorphisms (SNPs) are single nucleotide alterations that can be used as genomic markers disclosing susceptibility to complex diseases like AD. Epistasis has long been significant for recognizing the function of genetic pathways and the evolutionary dynamics of difficult genetic systems. Discovering epistasis interactions holds a vital key to personalized medicine (PM). PM needs a better understanding of the relationship between human genetic data and complex diseases. In this proposed work, a deep neural network (DNN) is applied using SHapley Additive exPlanations (SHAP) to get top 20, 100, 300, and 500 ranking SNPs responsible for AD risk through epistasis interactions. Multi-locus interaction analysis is performed on these identified SNPs using Multifactor Dimensionality Reduction (MDR). This constructive induction algorithm is integrated with DNN for discovering epistasis interactions in a computationally effective method. The proposed framework is applied to Alzheimer's Disease Neuroimaging Initiative (ADNI) dataset. The best accuracies are achieved using the top 500 SNPs, and the classification accuracies varied between 0.860 and 0.874 in the five-way interaction model. However, the classification accuracies of 2-way, 3-way, 4-way models varied between 0.663 and 0.670, 0.718 and 0.727, and 0.793 and 0.803, respectively. The results revealed that the reported accuracy scores of the proposed framework outperform the referenced literature work. The proposed framework presents high-ranked risk genes and promising epistasis interactions that may help in explaining the risk of AD.
更多查看译文
关键词
AD,SNPs,PM,DNN,SHAP,MDR,ADNI,GWAS,A,G,T,C,NB,TAN,QC,LD,BA
AI 理解论文
溯源树
样例
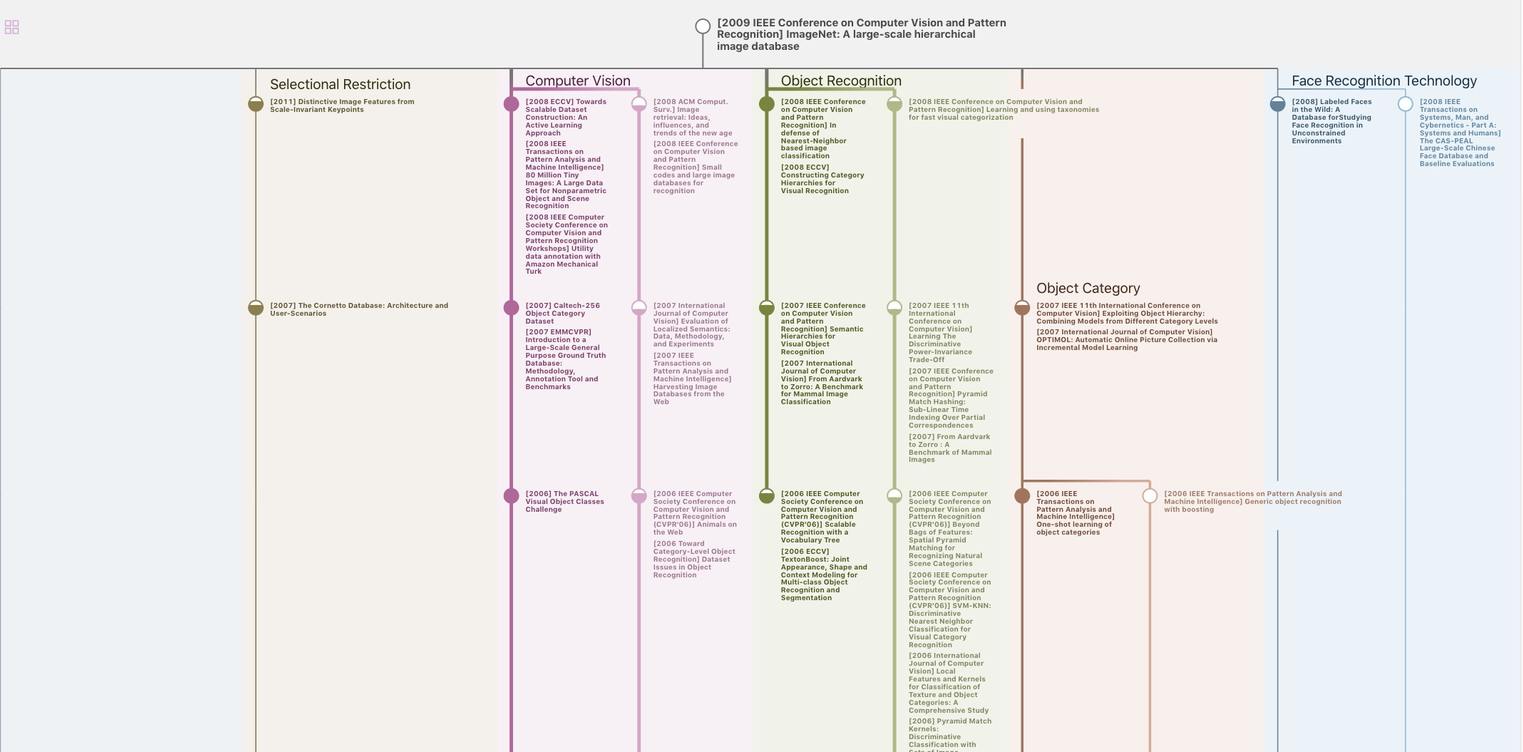
生成溯源树,研究论文发展脉络
Chat Paper
正在生成论文摘要