Two-stage unsupervised facial image quality measurement
Information Sciences(2022)
摘要
The performance of face recognition methods predominantly depends on the quality of facial images. Therefore, by filtering low-quality facial images, face recognizers can be effectively implemented. Moreover, the factors causing degradation in the quality of facial images include the face acquisition environment (e.g., illumination, motion blur, and resolution) and facial characteristics (e.g., pose and occlusion). Therefore, in this study, we propose a novel two-stage unsupervised facial image quality measure (TUFIQM) algorithm to evaluate the degradations caused by the acquisition environment and face factors. The proposed TUFIQM consists of an environmental degradation estimation module (EDEM) and a face factor degradation measurement module (FFDMM). Specifically, the EDEM introduces meta-learning to learn prior knowledge from the subjective quality assessment of natural scene images; subsequently, it employs the acquired prior knowledge to estimate the face quality degradation caused by the face acquisition environment. The design principle of the EDEM is based on the similar effect demonstrated by the acquisition environment on the quality of facial and natural scene images. Considering that face factor degradation leads to loss of highly identifiable high-frequency facial features, the FFDMM quantifies the amount of high-frequency information in visible face areas to represent the degradation in quality due to varying facial factors. The EDEM and FFDMM were combined in this study to achieve the proposed TUFIQM. Experiments on public datasets showed that the proposed TUFIQM algorithm is better than other state-of-the-art image quality measurement methods devised for natural scene and facial images.
更多查看译文
关键词
Face recognition,Facial image quality measurement,Environmental degradation estimation,Face factor degradation measurement
AI 理解论文
溯源树
样例
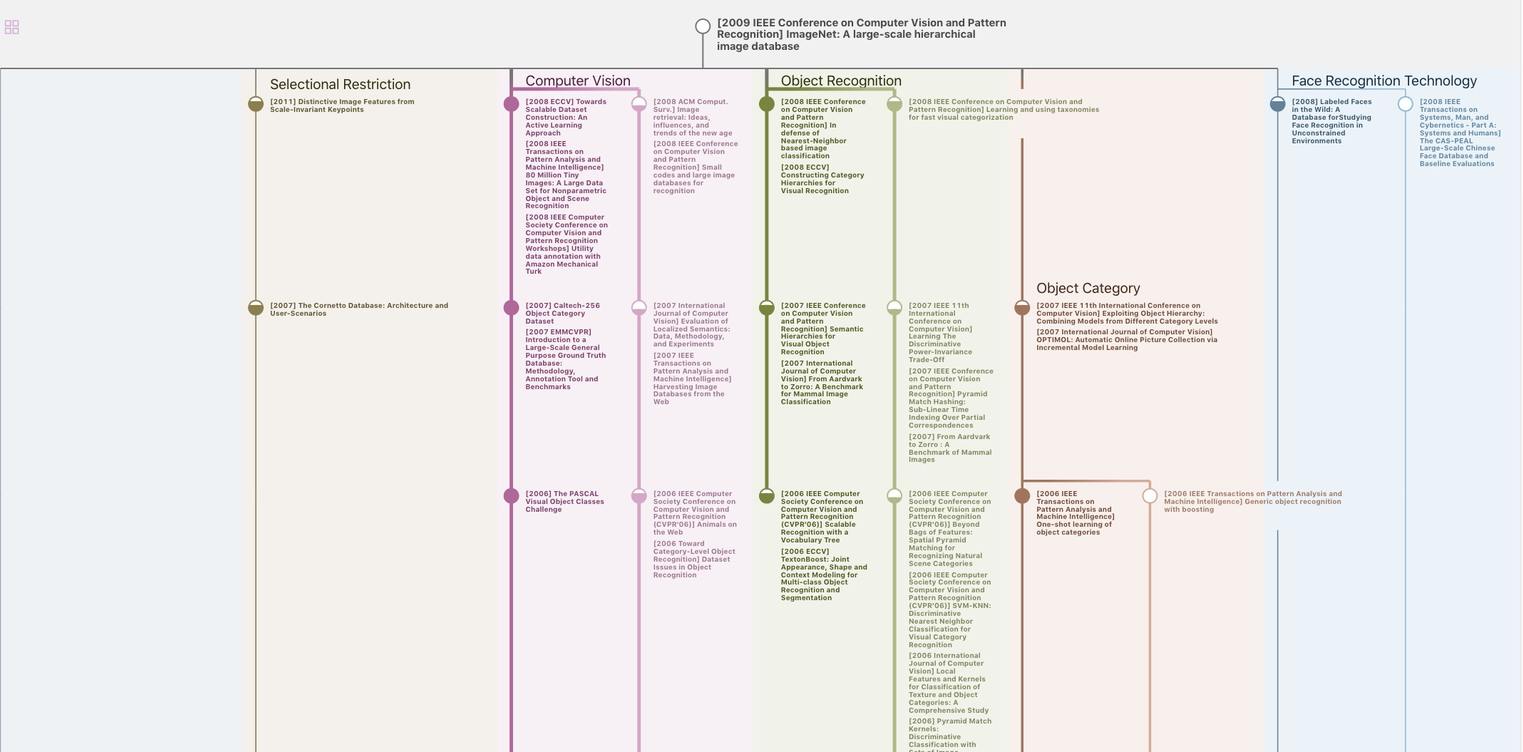
生成溯源树,研究论文发展脉络
Chat Paper
正在生成论文摘要