Self-training method based on GCN for semi-supervised short text classification
Information Sciences(2022)
摘要
Semi-supervised short text classification is a challenging problem due to the sparsity and limited labeled data. Due to the lack of labeled data, many models focus on the generation of text samples, which is cumbersome and has poor scalability. To overcome this deficiency, in this paper, we propose a Self-Training Text method based on Graph Convolutional Networks (ST-Text-GCN). Differently from the previous literature, our self-training method is convenient. The labeled information is propagated to target samples along the structure of the manifold, instead of introducing the extra knowledge. Specifically, instead of adding text training samples, our method adds keywords to training set. The model will calculate the confidence of each word. Confidence indicates the degree of ambiguity of a word. Some words with high confidence are automatically marked as pseudo-labeled data. Meanwhile, word confidence is added to the calculation of the edge weights of the graph to reduce the classification error caused by word ambiguity. Our method makes full use of the keywords in short texts when labeled data is scarce. Extensive experimental results have demonstrated that our proposed method outperforms state-of-the-art models on multiple benchmark datasets.
更多查看译文
关键词
Self-training,Graph convolutional networks (GCN),Semi-supervised,Short text classification
AI 理解论文
溯源树
样例
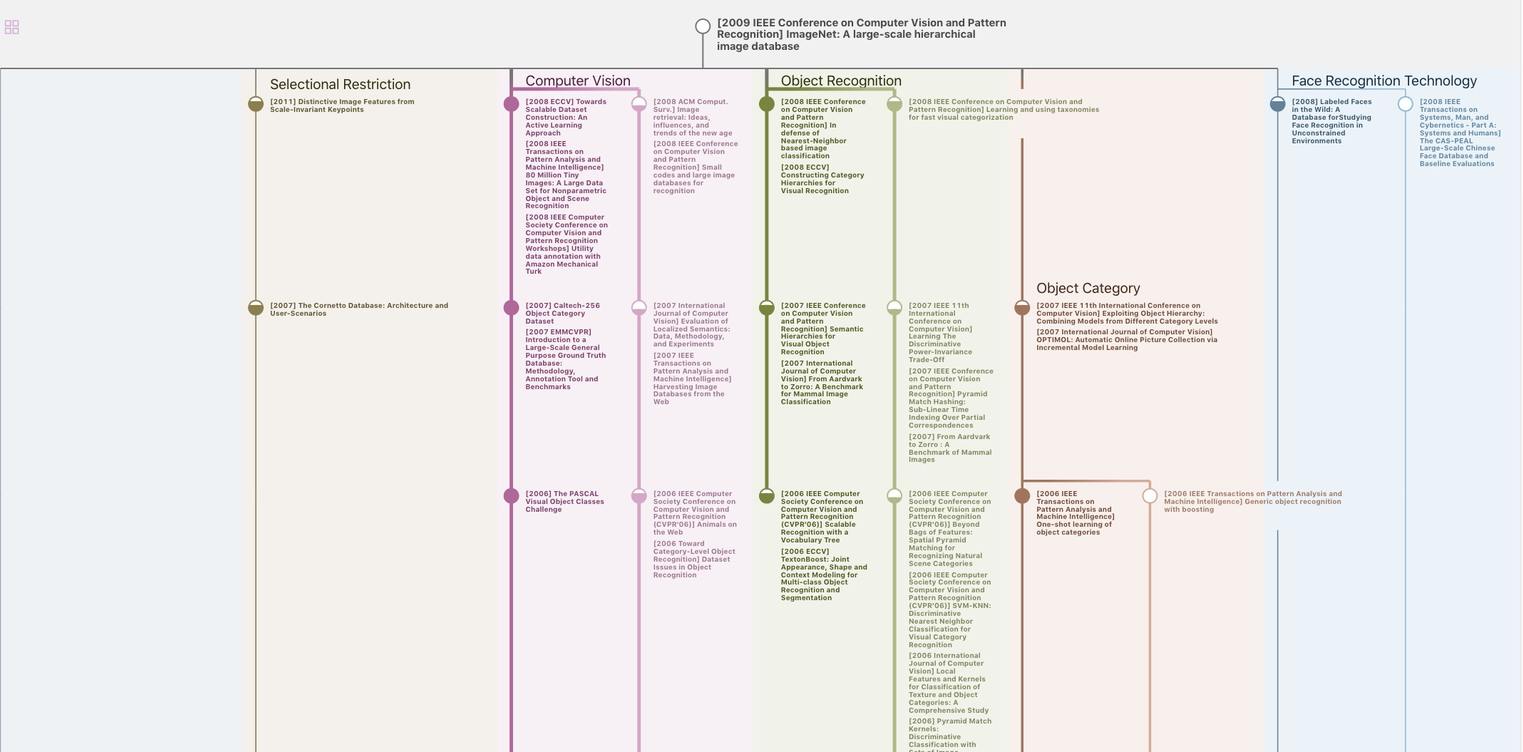
生成溯源树,研究论文发展脉络
Chat Paper
正在生成论文摘要