CLAD: A realistic Continual Learning benchmark for Autonomous Driving.
Neural networks : the official journal of the International Neural Network Society(2023)
摘要
In this paper we describe the design and the ideas motivating a new Continual Learning benchmark for Autonomous Driving (CLAD), that focuses on the problems of object classification and object detection. The benchmark utilises SODA10M, a recently released large-scale dataset that concerns autonomous driving related problems. First, we review and discuss existing continual learning benchmarks, how they are related, and show that most are extreme cases of continual learning. To this end, we survey the benchmarks used in continual learning papers at three highly ranked computer vision conferences. Next, we introduce CLAD-C, an online classification benchmark realised through a chronological data stream that poses both class and domain incremental challenges; and CLAD-D, a domain incremental continual object detection benchmark. We examine the inherent difficulties and challenges posed by the benchmark, through a survey of the techniques and methods used by the top-3 participants in a CLAD-challenge workshop at ICCV 2021. We conclude with possible pathways to improve the current continual learning state of the art, and which directions we deem promising for future research.
更多查看译文
关键词
Benchmark,Challenge report,Classification,Continual learning,Object detection
AI 理解论文
溯源树
样例
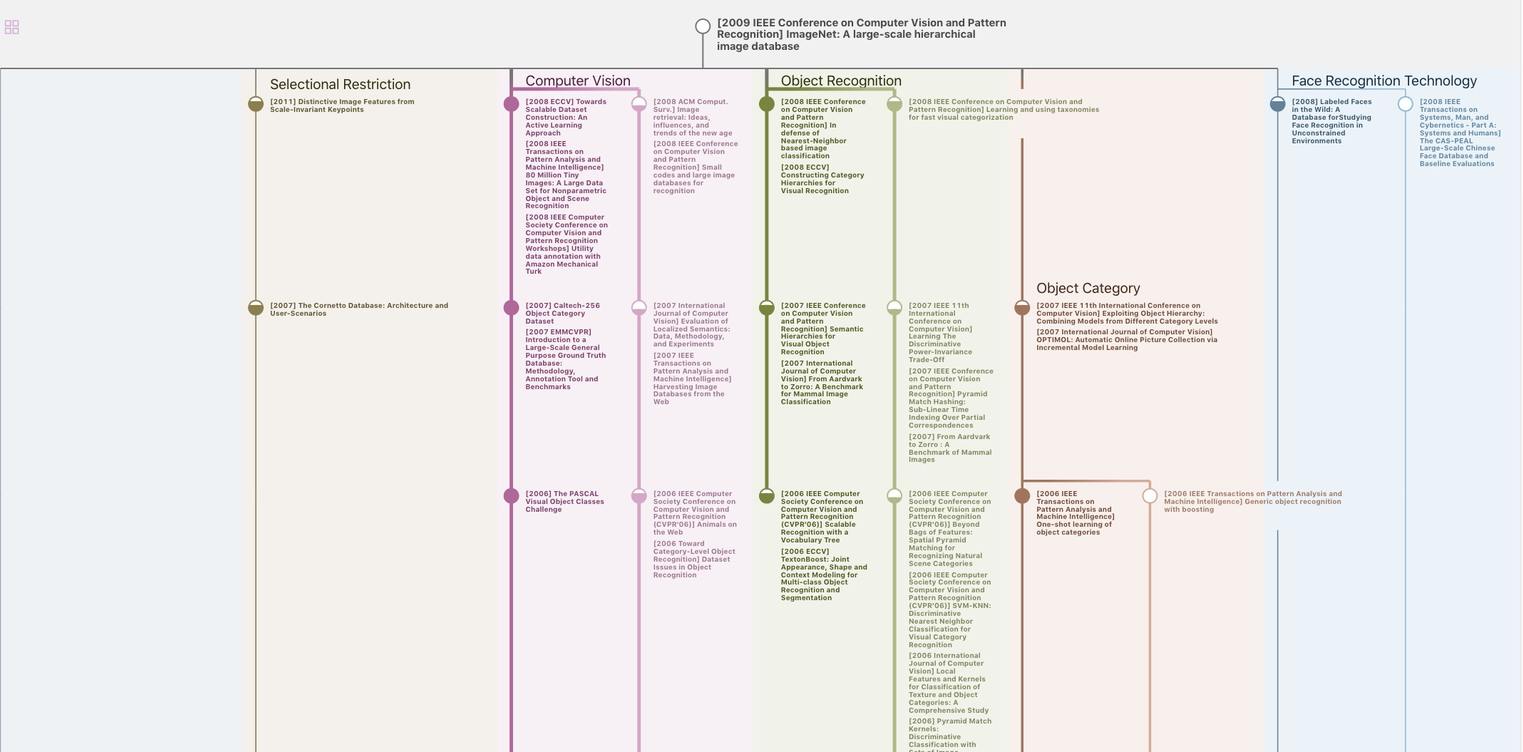
生成溯源树,研究论文发展脉络
Chat Paper
正在生成论文摘要