InfoOT: Information Maximizing Optimal Transport
ICLR 2023(2022)
摘要
Optimal transport aligns samples across distributions by minimizing the transportation cost between them, e.g., the geometric distances. Yet, it ignores coherence structure in the data such as clusters, does not handle outliers well, and cannot integrate new data points. To address these drawbacks, we propose InfoOT, an information-theoretic extension of optimal transport that maximizes the mutual information between domains while minimizing geometric distances. The resulting objective can still be formulated as a (generalized) optimal transport problem, and can be efficiently solved by projected gradient descent. This formulation yields a new projection method that is robust to outliers and generalizes to unseen samples. Empirically, InfoOT improves the quality of alignments across benchmarks in domain adaptation, cross-domain retrieval, and single-cell alignment.
更多查看译文
关键词
Optimal Transport,Unsupervised Alignment,Domain Adaptation,Mutual Information
AI 理解论文
溯源树
样例
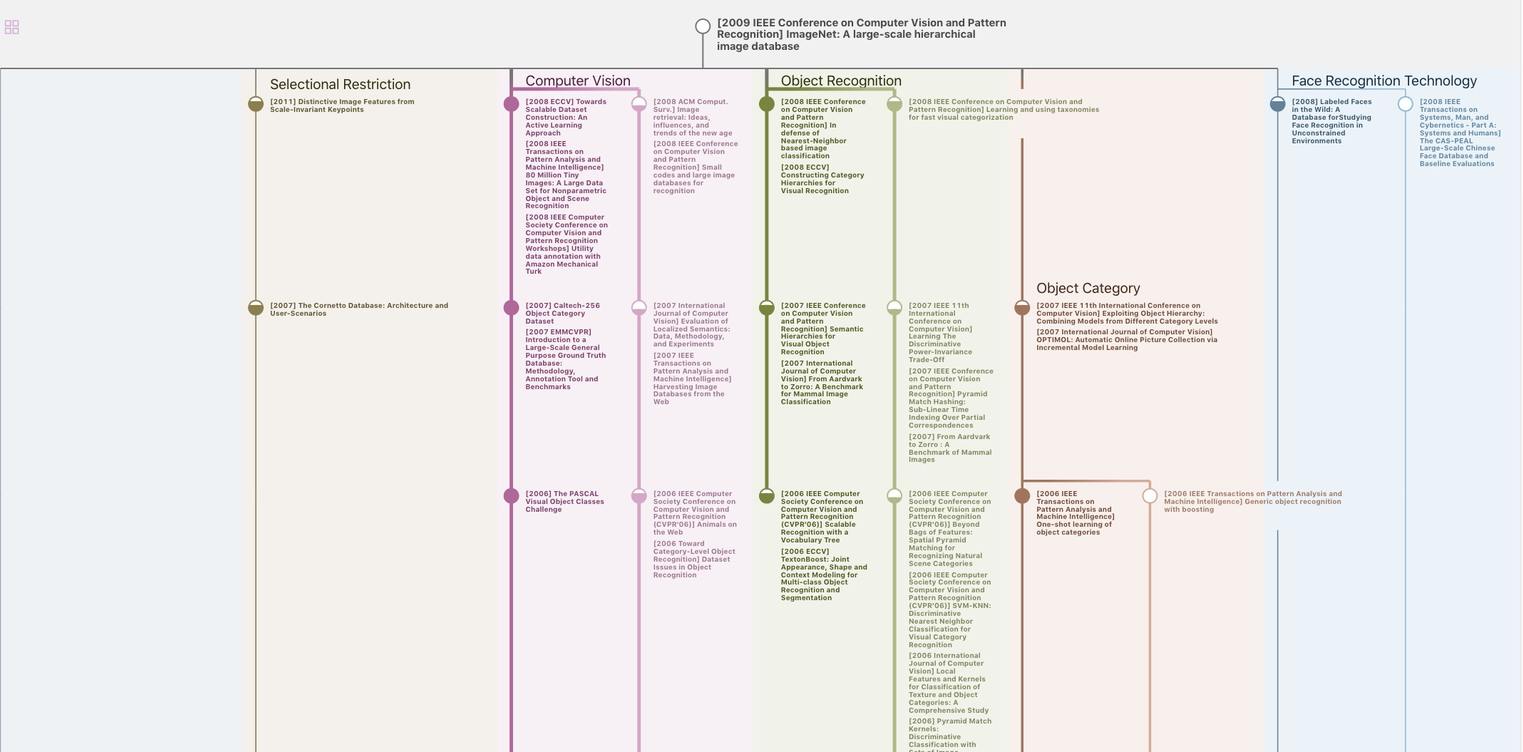
生成溯源树,研究论文发展脉络
Chat Paper
正在生成论文摘要