Designing lattices for impact protection using transfer learning
Matter(2022)
摘要
Like many specialty applications, the pace of designing structures for impact protection is limited by its reliance on specialized testing. Here, we develop a transfer learning approach to determine how more widely available quasi-static testing can be used to predict impact protection. We first extensively test a parametric family of lattices in both impact and quasi-static domains and train a model that predicts impact performance to within 8% using only quasi-static measurements. Next, we test the transferability of this model using a distinct family of lattices and find that performance rank was well predicted even for structures whose behavior extrapolated beyond the training set. Finally, we combine 812 quasi-static and 141 impact tests to train a model that predicts absolute impact performance of novel lattices with 18% error. These results highlight a path for accelerating design for specialty applications and that transferrable mechanical insight can be obtained in a data-driven manner.
更多查看译文
关键词
impact protection,transfer learning,automated experimentation,structured matter,additive manufacturing,lattice design,machine learning
AI 理解论文
溯源树
样例
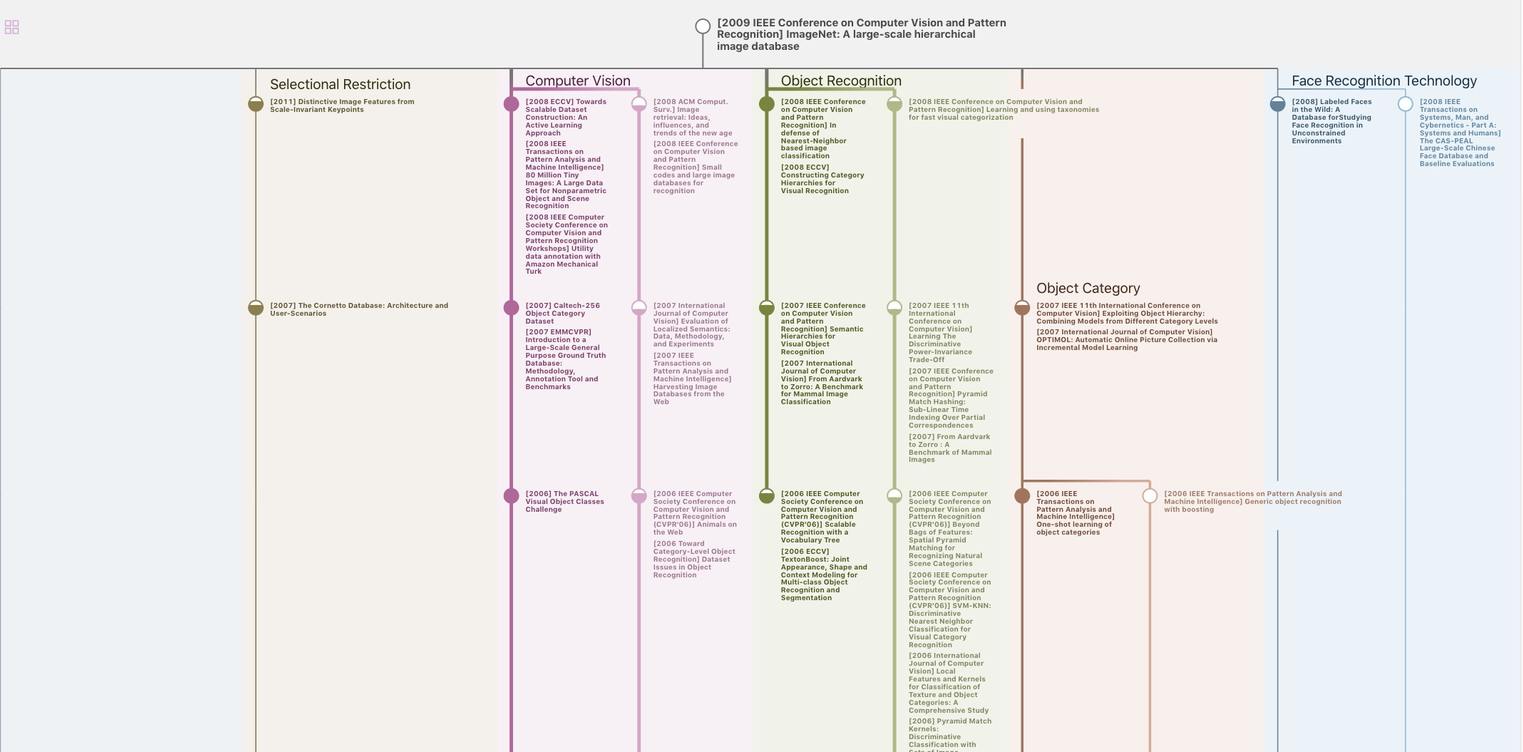
生成溯源树,研究论文发展脉络
Chat Paper
正在生成论文摘要