Knowledge-based turbomachinery design system via a deep neural network and multi-output Gaussian process
Knowledge-Based Systems(2022)
摘要
The requirements of future aeroengines challenge turbomachinery designs to be quieter, greener, and more efficient; furthermore, they must be developed at considerably reduced cycles and costs. Traditional turbomachinery design systems involve the iterative design–evaluation–improvement process, leading to high computational costs and considerable reliance on expert experience. In this paper, a knowledge-based turbomachinery aerodynamic design system consisting of a design of experiment framework and a two-stage modeling is proposed. In the first phase of the two-stage modeling, a deep neural network (DNN)-enhanced multi-output Gaussian process model is constructed. This model determines the optimal loading distribution from reference designs that are optimized for the desired performance. In the second stage, a DNN model is developed to predict the turbine profile geometry for a given loading distribution. This formulation enables the automatic determination of the optimal turbine geometry without an iterative process. Furthermore, the knowledge in the design system grows with the increasing database. The results indicate that the L2 relative errors for predicting the optimal pressure coefficient distribution and turbine profile geometry are less than 0.25% and 1.7%, respectively. A design case of a low-pressure turbine is used to validate the entire design system. The results reveal that the turbine geometry generated by the proposed design system outperforms that of the benchmark case in terms of the design and off-design aerodynamic performance. The foregoing observations are finally confirmed through experiments.
更多查看译文
关键词
Turbomachinery design system,Deep neural network,Multi-output Gaussian process,Knowledge-based modeling,Bayesian optimization
AI 理解论文
溯源树
样例
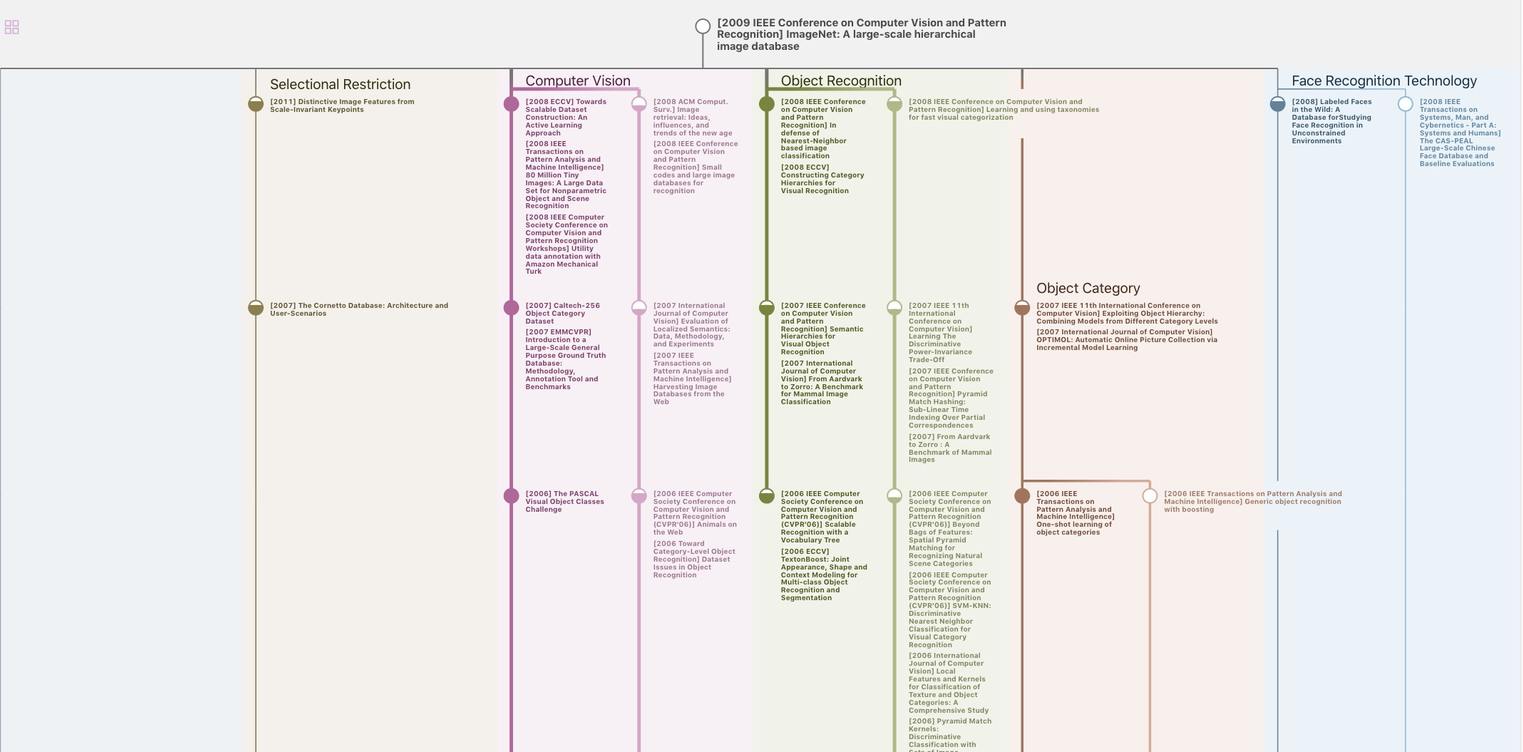
生成溯源树,研究论文发展脉络
Chat Paper
正在生成论文摘要