Federated low-rank tensor projections for sequential recommendation
Knowledge-Based Systems(2022)
摘要
Recommender systems have achieved great success in many fields, benefiting from the accessibility of massive amounts of user behavior data. However, the increasing concern about privacy issues brings new research challenges to traditional data-centralized recommender systems. As a privacy-preserving machine learning technique, federated learning provides potential solutions to these research challenges, by jointly training perceptive global recommendation models based on the decentralized user behavior data. Although existing federated recommendation methods have achieved promising results, they still suffer the following limitations. Firstly, they mainly focus on the horizontal federated recommendation scenarios where the participants are individual users, without considering the scenarios where participants are organizations. Secondly, they tend to model static user preferences and cannot learn the preference evolution in users’ interaction sequences. To address these limitations, we propose a horizontal Federated recommendation framework for Sequential Recommendation (FedSeqRec), where the participants are organizations. As the data in different organizations are usually not independent and identically distributed (Non-IID), federated training tends to yield suboptimal results. To alleviate this problem, we propose a federated re-average algorithm, which takes the consistency of the client’s data distribution with the overall data distribution into consideration. Moreover, we also leverage a low-rank tensor projection to model user long-term preferences. Experimental results on two real-world datasets demonstrate that FedSeqRec outperforms state-of-the-art federated recommendation methods. The implementation code of FedSeqRec is available at https://github.com/MuziLee-x/FedSeqRec.
更多查看译文
关键词
Federated learning,Federated recommendation,Sequential recommendation
AI 理解论文
溯源树
样例
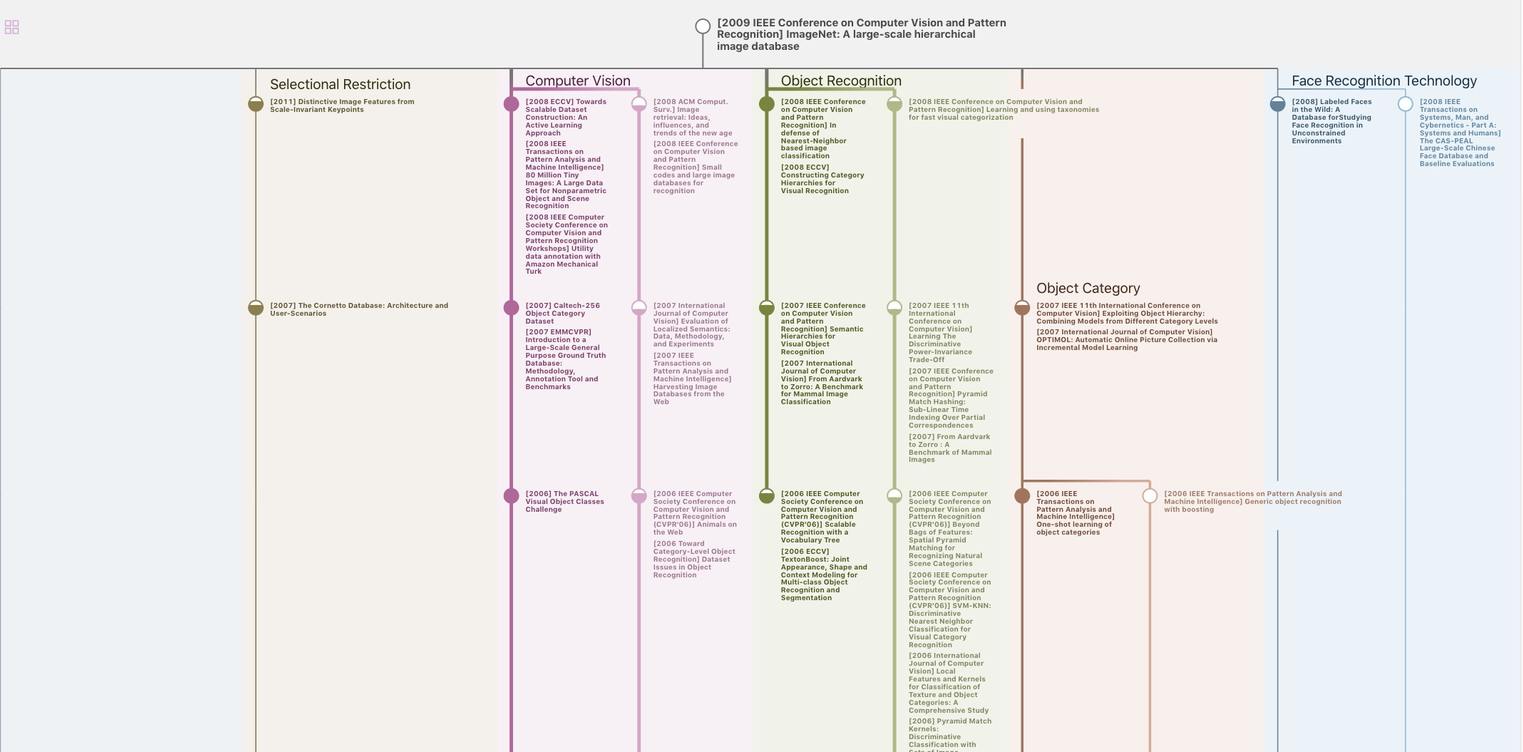
生成溯源树,研究论文发展脉络
Chat Paper
正在生成论文摘要