Dynamic transfer partial least squares for domain adaptive regression
Journal of Process Control(2022)
摘要
The traditional soft sensor models are based on the independent and identical distribution assumption, which are difficult to adapt to changes in data distribution under multiple operating conditions, resulting in model performance deterioration. The domain adaptive transfer learning methods learn knowledge in different domains by means of distribution alignment, which can reduce the impact of data distribution differences, and effectively improve the generalization ability of the model. However, most of the existing models established by domain adaptation methods are static models, which cannot reflect the dynamic characteristics of the system, and have limited prediction accuracy when applied to dynamic system modeling under multiple operating conditions. The dynamic system modeling methods can effectively extract the dynamic characteristics of the data, but they cannot deal with the concept drift problem caused by the change of data distribution. This paper proposes a new dynamic transfer partial least squares method, which maps the high-dimensional process data into the low-dimensional latent variable subspace, establishes the dynamic regression relationship between the latent variables and the labels, and realizes the systematic dynamic modeling, at the same time, the model adds regular terms for distribution alignment and structure preservation, which realizes dynamic alignment of data distribution difference. The effectiveness of the proposed method is validated on three publicly available industrial process datasets.
更多查看译文
关键词
Transfer learning,Dynamic partial least squares,Domain adaptive regression
AI 理解论文
溯源树
样例
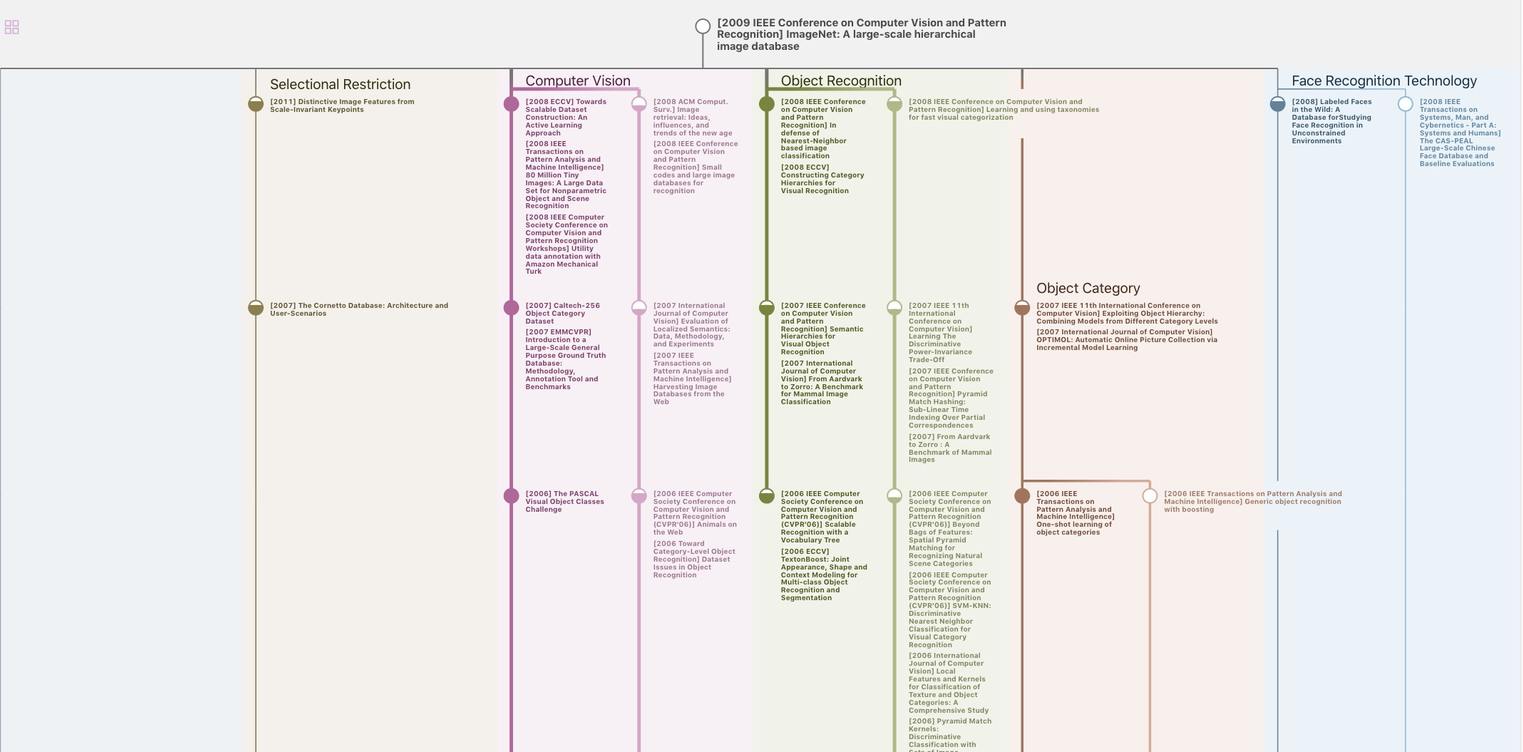
生成溯源树,研究论文发展脉络
Chat Paper
正在生成论文摘要