Improved hybrid resampling and ensemble model for imbalance learning and credit evaluation
Journal of Management Science and Engineering(2022)
摘要
A clustering-based undersampling (CUS) and distance-based near-miss method are widely used in current imbalanced learning algorithms, but this method has certain drawbacks. In particular, the CUS does not consider the influence of the distance factor on the majority of instances, and the near-miss method omits the inter-class(es) within the majority of samples. To overcome these drawbacks, this study proposes an undersampling method combining distance measurement and majority class clustering. Resampling methods are used to develop an ensemble-based imbalanced-learning algorithm called the clustering and distance-based imbalance learning model (CDEILM). This algorithm combines distance-based undersampling, feature selection, and ensemble learning. In addition, a cluster size-based resampling (CSBR) method is proposed for preserving the original distribution of the majority class, and a hybrid imbalanced learning framework is constructed by fusing various types of resampling methods. The combination of CDEILM and CSBR can be considered as a specific case of this hybrid framework. The experimental results show that the CDEILM and CSBR methods can achieve better performance than the benchmark methods, and that the hybrid model provides the best results under most circumstances. Therefore, the proposed model can be used as an alternative imbalanced learning method under specific circumstances, e.g., for providing a solution to credit evaluation problems in financial applications.
更多查看译文
关键词
Imbalanced learning,Clustering-based under-sampling,Ensemble methods,Hybrid methods,Credit risk evaluation
AI 理解论文
溯源树
样例
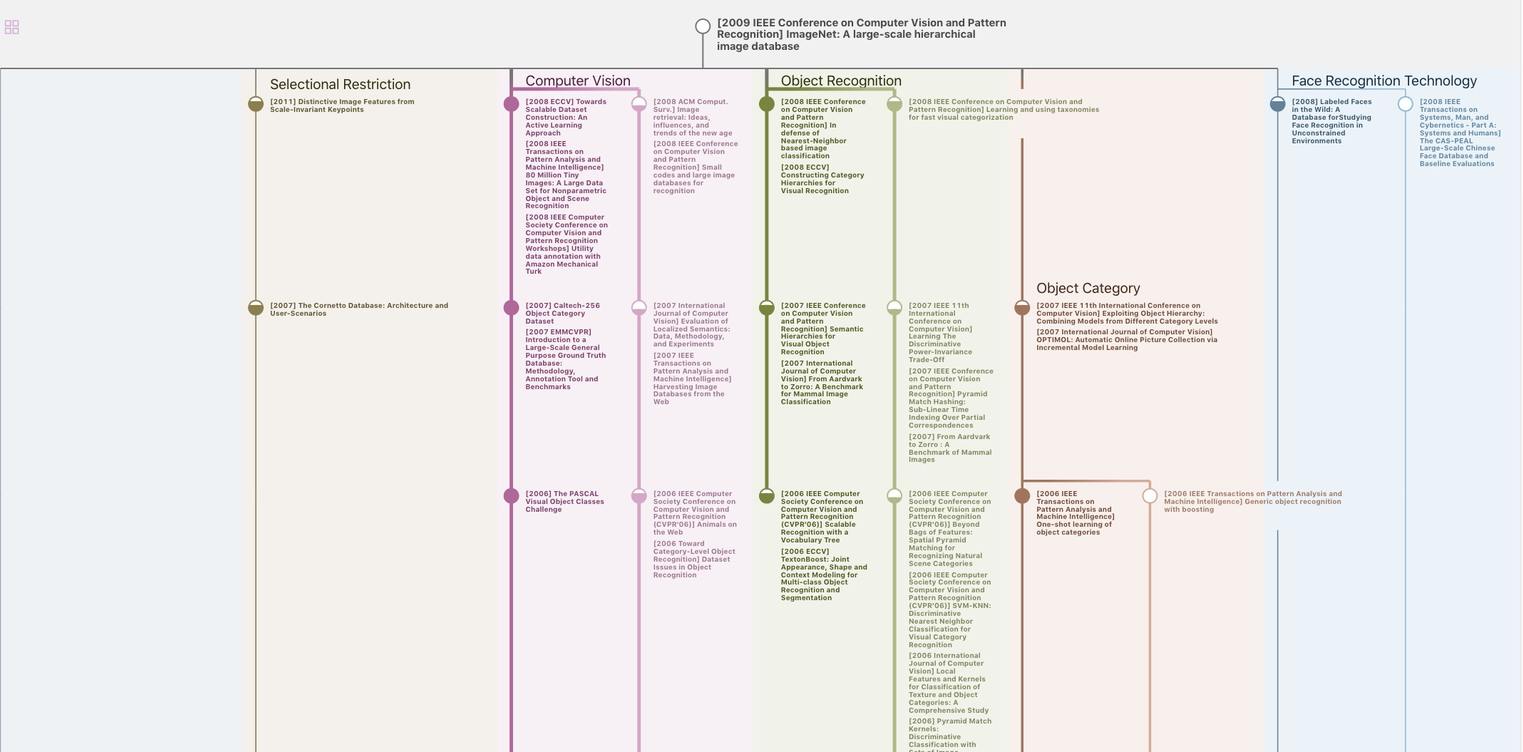
生成溯源树,研究论文发展脉络
Chat Paper
正在生成论文摘要