Predicting the magnetic measurements of first- and second-order phase transition magnetocaloric materials with artificial neural networks
Journal of Magnetism and Magnetic Materials(2022)
摘要
Due to the large innovative applications involving magnetocaloric materials, such as magnetic cooling devices and thermomagnetic engines, the number of studies on their characterization have been increasing over the past two decades. Although indispensable, magnetic measurements are considerably time-consuming, which sometimes hinder depth studies on the subject. We propose a method that uses artificial neural networks to predict magnetic measurements of magnetocaloric materials with first- and second-order phase transitions, aiming to reduce the required number of measured field dependent magnetization curves M(H) in standard studies. We validate the method for magnetic measurements of the first- and second-order phase transition materials Gd5Ge4 and Gd5Si2.4Ge1.6, respectively. The application of the proposed method results in very high accuracies, with r2>0.98, when comparing the prediction to measured M(H) curves. The entropy change vs temperature curves upon the application of 5 T magnetic fields are also predicted.
更多查看译文
关键词
Magnetocaloric material,Magnetic measurement,Artificial neural network,Machine learning,First-order phase transition,Second-order phase transition
AI 理解论文
溯源树
样例
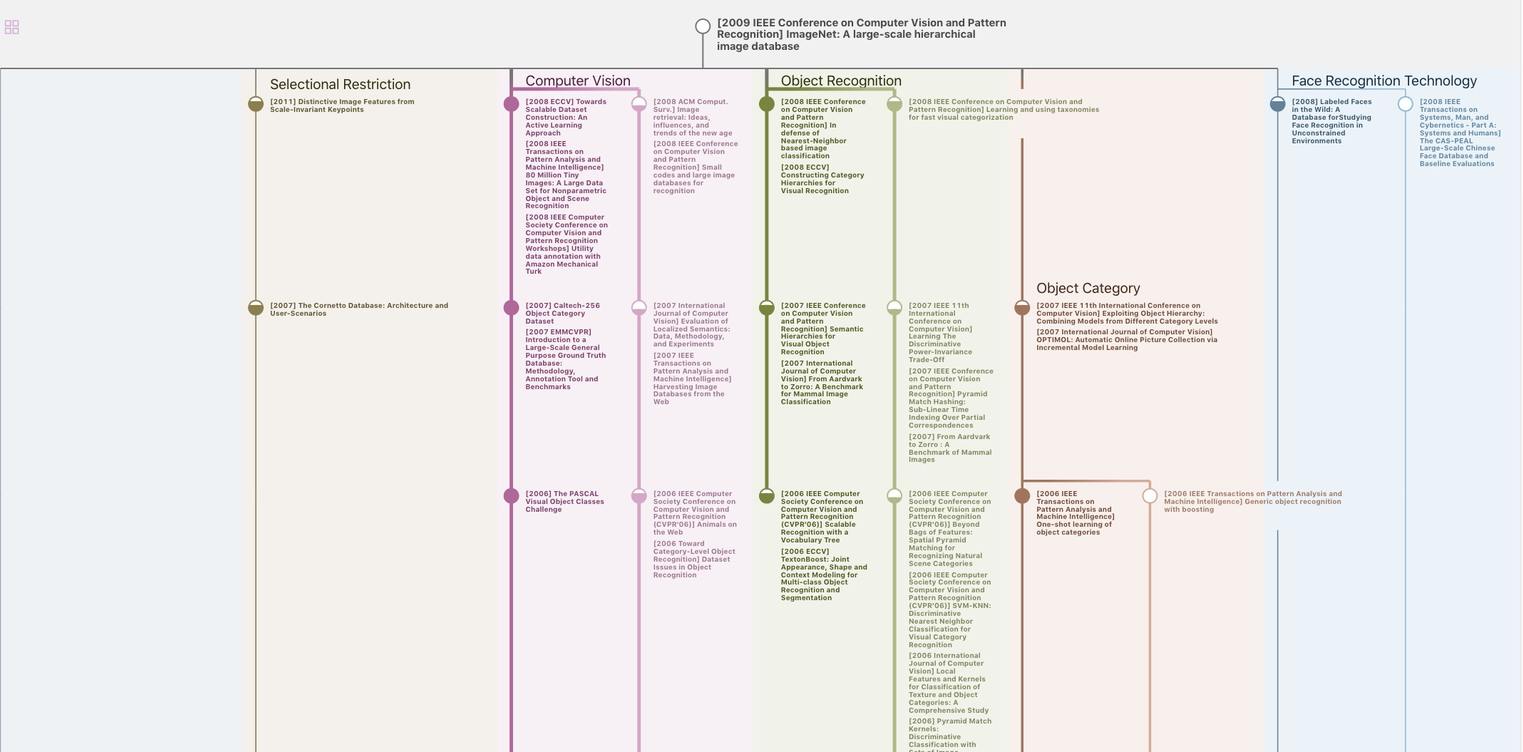
生成溯源树,研究论文发展脉络
Chat Paper
正在生成论文摘要