Feature fusion and Ensemble learning-based CNN model for mammographic image classification
Journal of King Saud University - Computer and Information Sciences(2022)
摘要
In recent times, the world has faced an alarming situation regarding breast cancer patients. The early diagnosis of this deadly disease can make the treatment more accessible and practical. In this regard, a Computer-Aided Diagnosis (CAD) system can assist the radiologists in distinguishing the normal and abnormal tissues and diagnosing the pathological stages. The classification task is challenging in CAD systems because of noisy and low contrast mammogram images, tumors' shape and location variations, and the high resemblance between the normal and tumor regions of interest (ROI). We propose a novel deep convolution neural network (DCNN) approach based on feature fusion and ensemble learning strategies to improve the detection and classification of abnormalities in mammographic scans. The feature fusion helps to detect discriminative features between the classes properly, while ensemble learning in the last block better classify the normal and tumor ROIs to get more authenticated results. Moreover, the role of spatial dropout and depthwise separable convolution is investigated for mammogram classification to better deal with overfitting and small dataset problems in medical images. The proposed model is evaluated on two publicly available datasets, MIAS and BCDR, getting high sensitivity, specificity, and accuracy of 0.995, 0.994, and 0.994, respectively, on the MIAS dataset.
更多查看译文
关键词
Breast cancer,Computer-Aided Diagnosis (CAD),Feature fusion,Ensemble learning,Mammograms classification
AI 理解论文
溯源树
样例
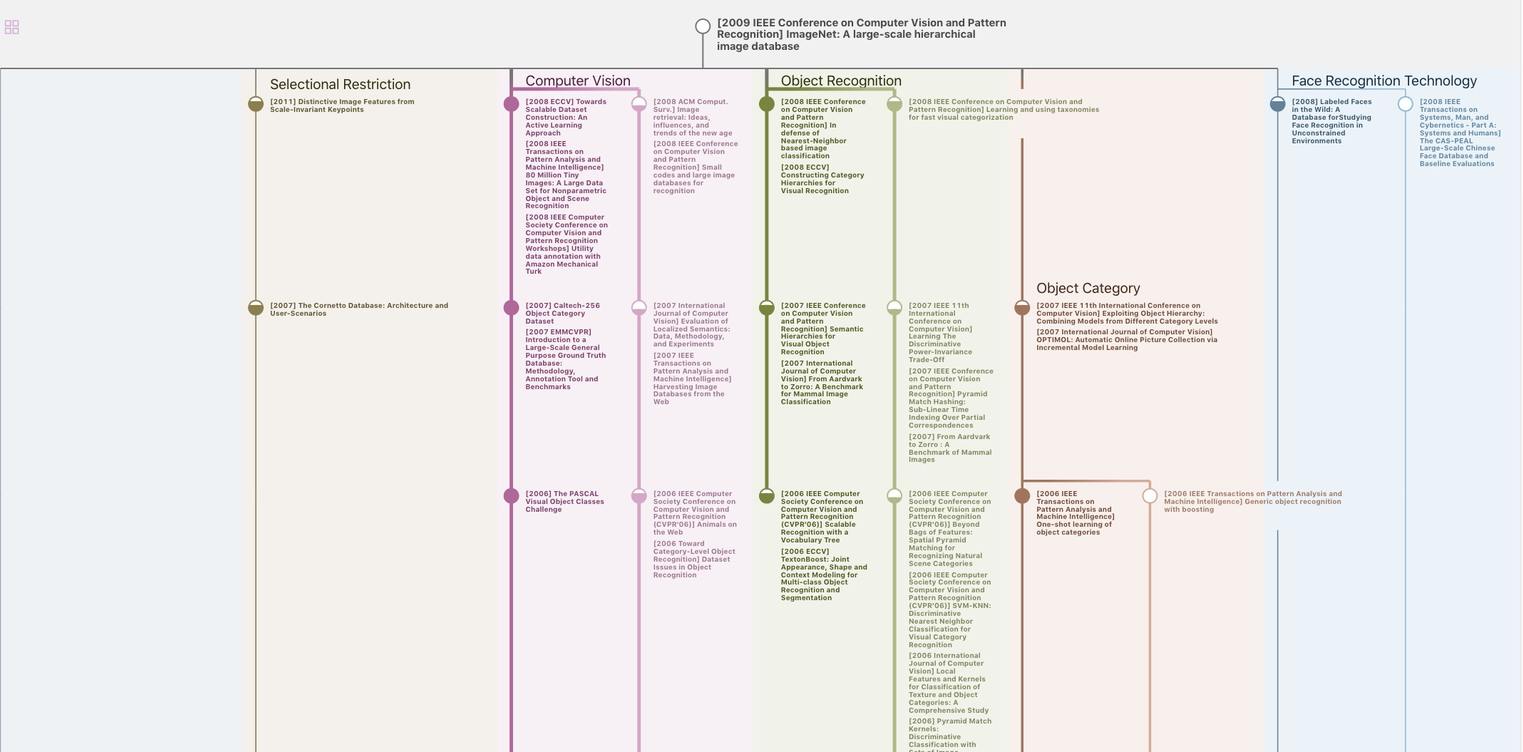
生成溯源树,研究论文发展脉络
Chat Paper
正在生成论文摘要