Structural model updating using variational inference
Proceedings of the 13th International Workshop on Structural Health Monitoring(2022)
摘要
Monte Carlo sampling approaches are frequently used for probabilistic model updating of physics-based models under parametric uncertainty due to their high accuracy. The model updating framework produces a model that represents the real system more accurately than the prior knowledge or assumptions. This statistically updated model may prove useful if Structural Health Monitoring (SHM) techniques are to be applied. However, the updating of the models requires the use of a high number of samples, implying a high computational cost. Another additional disadvantage of these methods is that most of them require the calibration of a high number of parameters for their algorithm to become sampling efficient. Variational inference (VI) is an alternative approach for inference often used by the machine learning community. An optimization algorithm is employed to choose from a family of distributions the member that best approximates the posterior. In the method described in this paper the variational posterior that maximises the evidence lower bound (ELBO) is chosen. An approach based on VI is proposed and implemented on two different numerical examples to infer the uncertain parameters by postulating a variational posterior distribution given by a multivariate Gaussian approximation. It has been found that the number of samples required for the calculation of the posterior is reduced compared with Monte Carlo sampling approaches, however this occurs at the cost of some accuracy. The methodology will be helpful for the development of enhanced SHM strategies that require fast inference under a limited computational budget.
更多查看译文
关键词
variational inference,structural model
AI 理解论文
溯源树
样例
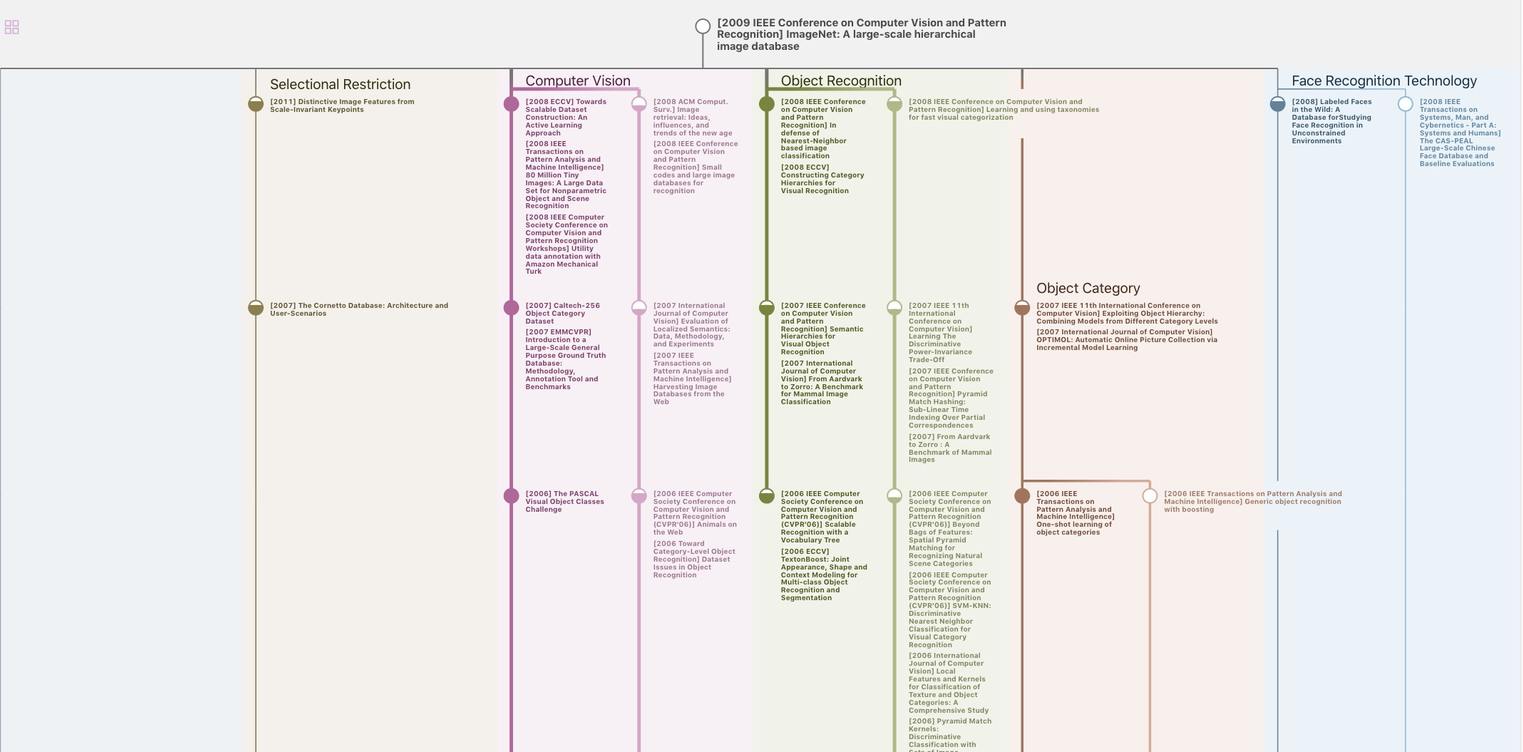
生成溯源树,研究论文发展脉络
Chat Paper
正在生成论文摘要