A majorization–minimization based solution to penalized nonnegative matrix factorization with orthogonal regularization
Journal of Computational and Applied Mathematics(2023)
摘要
Nonnegative matrix factorization (NMF) is a dimension reduction and clustering technique for data analysis which has been widely used in image processing, text analysis and hyperspectral decomposition because of its stronger practical significance and better interpretability. Approximate matrix factorization techniques with both nonnegativity and orthogonality constraints, referred to as orthogonal NMF (ONMF), have been shown to work remarkably better for clustering tasks than NMF. At present, a large number of algorithms have been used to solve the ONMF problems, but these methods usually cannot take into account the classification accuracy and calculation speed. In this paper, we propose a new form of penalized NMF with orthogonal regularization that combines the decomposition residual minimization based on the Euclidean distance and the orthogonality maximization based on the Kullback–Leibler divergence. This paper uses Majorization–Minimization (MM) method by minimizing a majorization function of the original problem and obtains a new iterative scheme (MM-ONMF). Comparing with several traditional ONMF methods on eight datasets, experimental results show that the proposed method has better clustering results and less computing time.
更多查看译文
关键词
Kullback–Leibler divergence,Majorization–Minimization method,Nonnegative matrix factorization,Orthogonal regularization
AI 理解论文
溯源树
样例
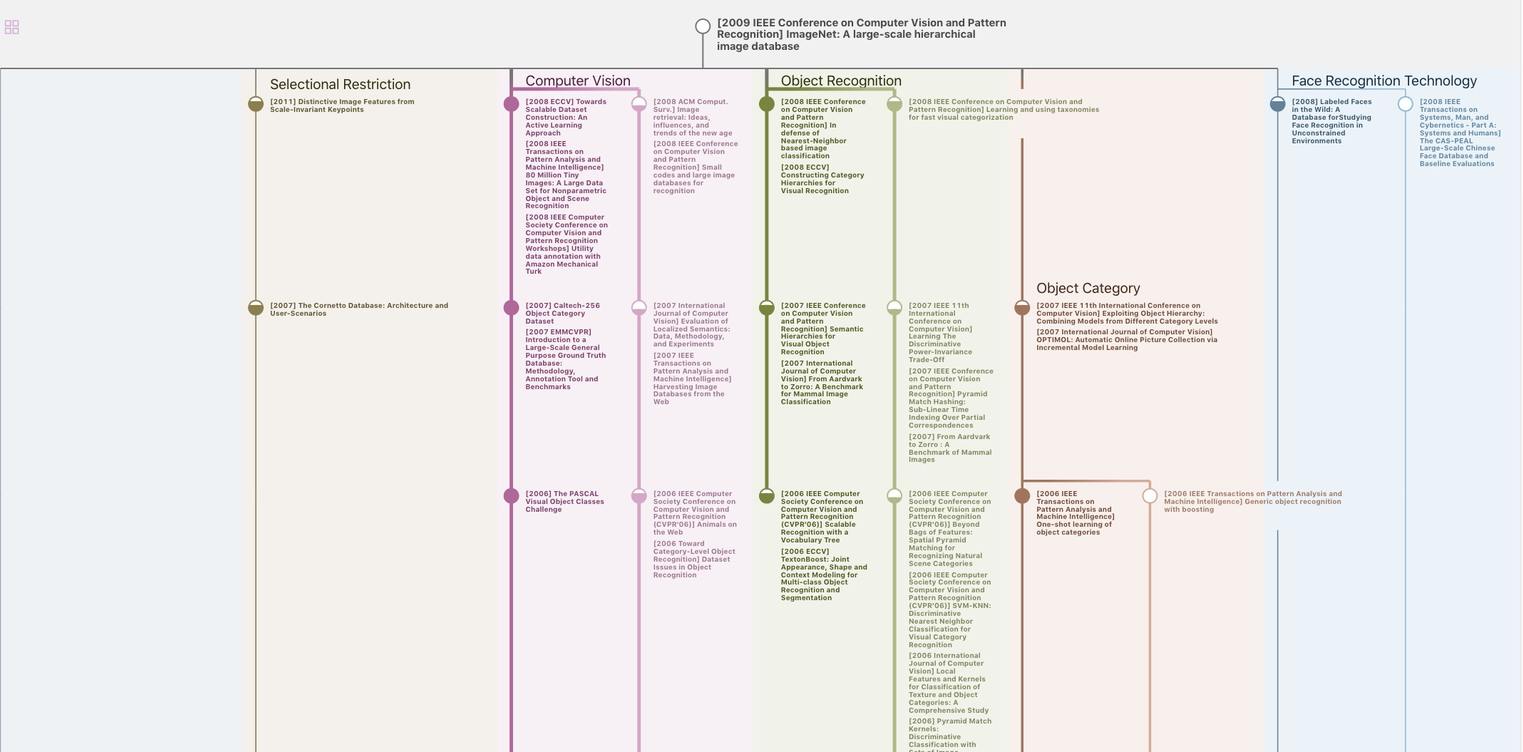
生成溯源树,研究论文发展脉络
Chat Paper
正在生成论文摘要