Network Intrusion Detection System Based on an Adversarial Auto-Encoder with Few Labeled Training Samples
Journal of Network and Systems Management(2022)
摘要
Network intrusion detection systems (NIDS) are critical to defending network systems from cyber attacks. Recently, machine learning has been applied to enhance NIDS capability. To train a supervised machine-learning model, a large number of labeled training samples are required to achieve practical performance. However, labeling data samples is a costly task. Additionally, obtaining anomaly data samples is difficult because trends in network traffic that are subject to NIDS change daily, and new attacks continue to be generated. To address this issue, we propose a semi-supervised machine-learning-based NIDS that reduces the required number of labeled training samples by applying an adversarial auto-encoder (AAE) technique. We evaluated the proposed method through a series of experiments and confirmed that the proposed AAE-based NIDS achieves performance comparable to that of multi-layer perceptron-based NIDS with only 0.1% of the labeled training samples. We also confirmed that the selection of data samples for annotation does not affect the performance of the proposed AAE-based NIDS. We also evaluated the relationship between the performance of the proposed method and the dimension of its latent-variable vector. The best performance as measured by recall and F1 score occurred when the dimensionality of the latent variable vector was 10, which suggests that this structure allows for accurate decomposition of attack and normal. This study presents promising results obtained by the proposed semi-supervised learning method with a reduced number of labeled training samples, which reduces the operational costs of a machine-learning-based NIDS.
更多查看译文
关键词
Adversarial auto-encoder,Network intrusion detection system,Semi-supervised learning
AI 理解论文
溯源树
样例
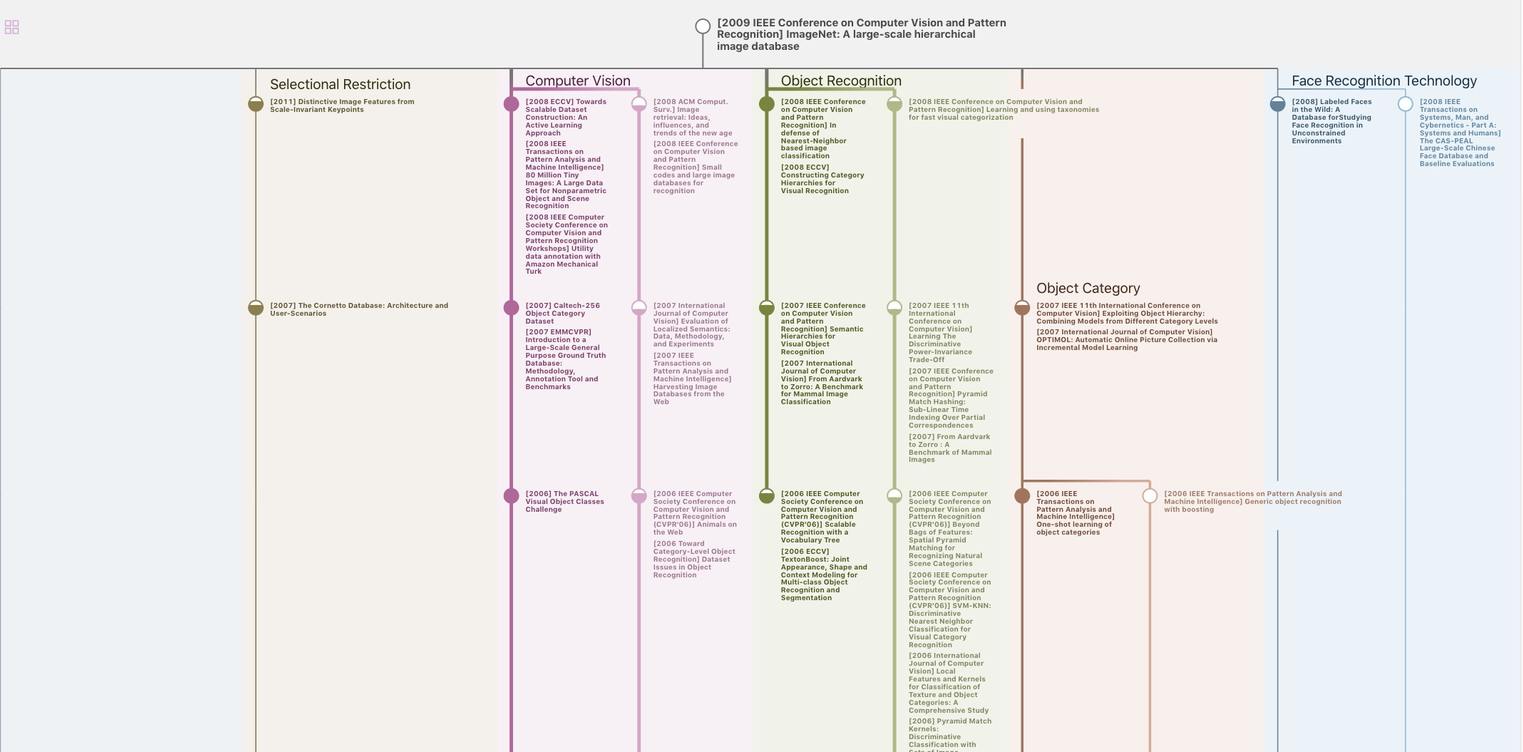
生成溯源树,研究论文发展脉络
Chat Paper
正在生成论文摘要