FedAda: Fast-convergent adaptive federated learning in heterogeneous mobile edge computing environment
World Wide Web(2022)
摘要
With rapid advancement of Internet of Things (IoT) and social networking applications generating large amounts of data at or close to the network edge, Mobile Edge Computing (MEC) has naturally been proposed to bring model training closer to where data is produced. However, there still exists privacy concern since typical MEC frameworks need to transfer sensitive data resources from data collection end devices/clients to MEC server. So the concept of Federated Learning (FL) has been introduced which supports privacy-preserved collaborative machine learning involving multiple clients coordinated by the MEC server without centralizing the private data. Unfortunately, FL is prone to multiple challenges: 1) systems heterogeneity between clients causes straggler issue, and 2) statistical heterogeneity between clients brings about objective inconsistency problem, both of which may lead to a significant slow-down in the convergence speed in heterogeneous MEC environment. In this paper, we propose a novel framework, FedAda (Federated Adaptive Training), that incorporates systems capabilities and data characteristics of the clients to adaptively assign appropriate workload to each client. The key idea is that instead of running a fixed number of local training iterations as in Federated Averaging (FedAvg), our algorithm adopts an adaptive workload assignment strategy by minimizing the runtime gap between clients and maximizing convergence gain in heterogeneous MEC environment. Moreover, we design a light mechanism extending FedAda to accelerate the convergence speed by further fine-tuning the workload assignment based on the global convergence status in each communication round. We evaluate FedAda on CIFAR-10 dataset to explore the performance of the algorithm in the simulated heterogeneous MEC environment. Experimental results show that FedAda is able to assign appropriate amount of workload to each client and substantially reduces the convergence time by up to 49.5% compared to FedAvg in heterogeneous MEC environment. In addition, we demonstrate that fine-tuning the workload assignment can help FedAda improve the learning performance in heterogeneous mobile edge computing environment.
更多查看译文
关键词
Mobile edge computing, Federated learning, Systems and statistical heterogeneity, Convergence speed, Adaptive workload assignment
AI 理解论文
溯源树
样例
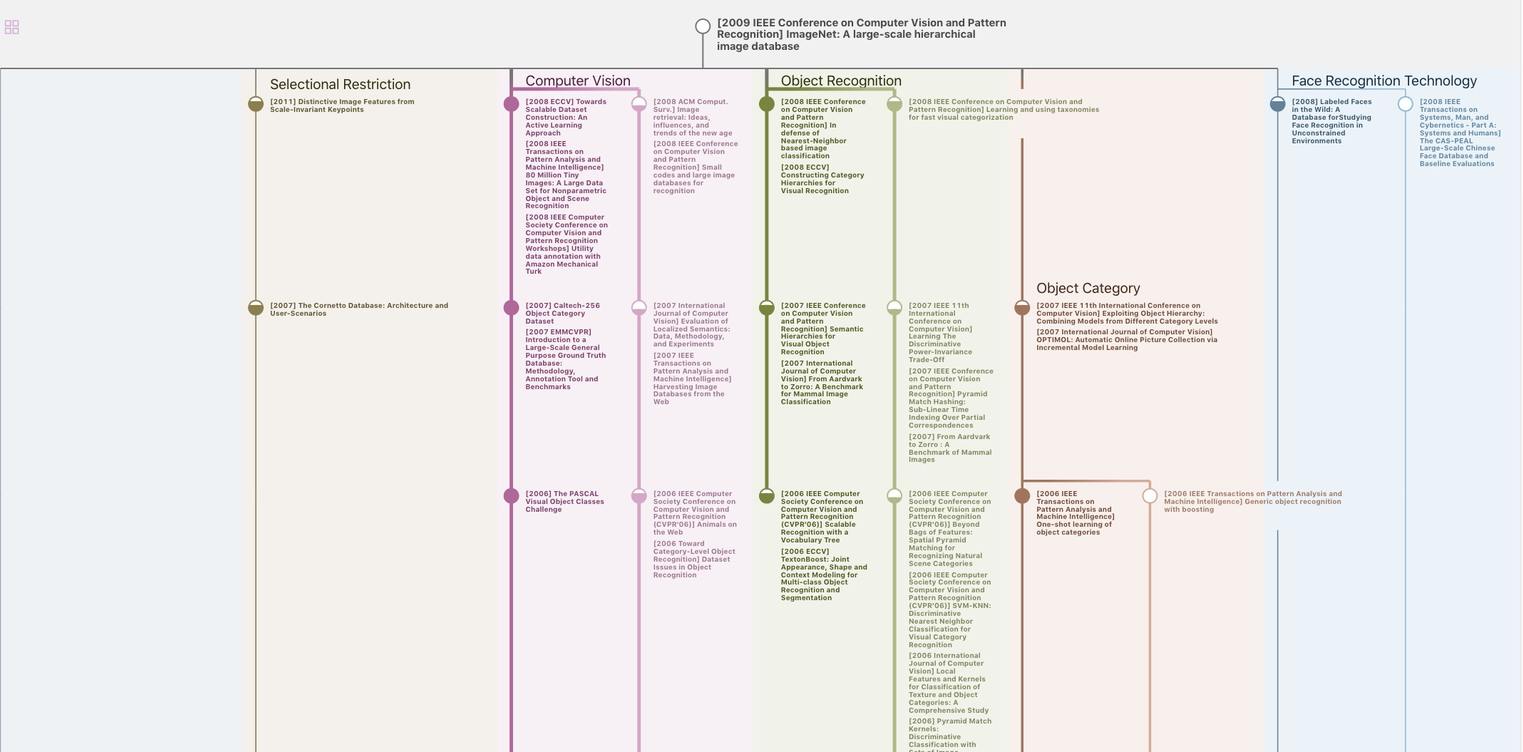
生成溯源树,研究论文发展脉络
Chat Paper
正在生成论文摘要