A Transformer based neural network for emotion recognition and visualizations of crucial EEG channels
Physica A: Statistical Mechanics and its Applications(2022)
摘要
With the rapid development of artificial intelligence and sensor technology, electroencephalogram-based (EEG) emotion recognition has attracted extensive attention. Various deep neural networks have been applied to it and achieved excellent results in classification accuracy. Except for classification accuracy, the interpretability of the feature extraction process is also considerable for model design for emotion recognition. In this study, we propose a novel neural network model (DCoT) with depthwise convolution and Transformer encoders for EEG-based emotion recognition by exploring the dependence of emotion recognition on each EEG channel and visualizing the captured features. Then we conduct subject-dependent and subject-independent experiments on a benchmark dataset, SEED, which contains EEG data of positive, neutral, and negative emotions. For subject-dependent experiments, the average accuracy of three classification tasks is 93.83%. For subject-independent experiments, the average accuracy of three classification tasks is 83.03%. Additionally, we assess the importance of each EEG channel in emotional activities by the DCoT model and visualize it as brain maps. Furthermore, satisfactory results are obtained by utilizing eight selected crucial EEG channels: FT7, T7, TP7, P3, FC6, FT8, T8, and F8, both in two classification tasks and three classification tasks. Using a small number of EEG channels for emotion recognition can reduce equipment costs and computing costs, which is suitable for practical applications.
更多查看译文
关键词
EEG,Emotion recognition,Transformer model,Deep learning,Time series analysis
AI 理解论文
溯源树
样例
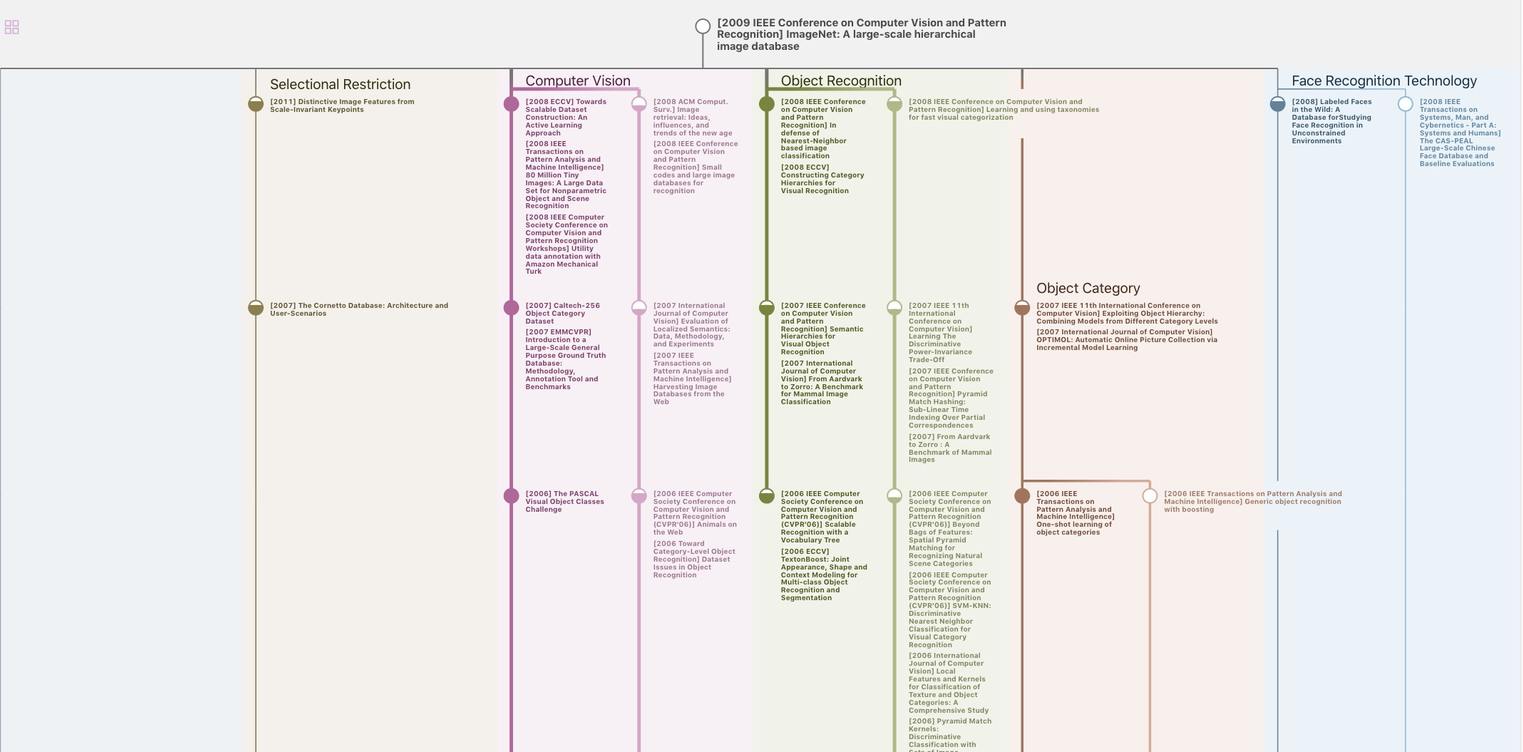
生成溯源树,研究论文发展脉络
Chat Paper
正在生成论文摘要