Automatic detection of bridge surface crack using improved YOLOv5s
International Journal of Pattern Recognition and Artificial Intelligence(2022)
摘要
Bridge crack detection is a key task in the structural health monitoring of Civil Engineering. In the traditional bridge crack detection methods, there exist some problems such as high cost, low speed, and complex structure. This paper developed a bridge surface crack detection system based on improved YOLOv5s. The GhostBottleneck module was employed to replace the classic C3 module of the YOLOv5s backbone network, meanwhile the channel attention module namely ECA-Net was also added to the network, which not only reduced the amount of calculation, but also enhanced the ability of the network in extracting cross-channel information features. The adaptive spatial feature fusion (ASFF) was introduced to address the conflict problem caused by the inconsistency of feature scale in the network feature fusion stage, and the transfer learning was utilized to train the network. The experimental results showed that the improved YOLOv5s performed better than Faster R-CNN, SSD, YOLOv3, and YOLOv5s, with the Precision of 93.6%, Recall of 95.4%, and mAP of 98.4%. Further, the improved YOLOv5s was deployed in PyQt5 to realize the real-time detection of bridge cracks. This research showed that the proposed model not only provides a novel solution for bridge surface crack detection, but also has certain industrial application value.
更多查看译文
关键词
Bridge crack detection,YOLOv5s,attention mechanism,feature fusion,transfer learning
AI 理解论文
溯源树
样例
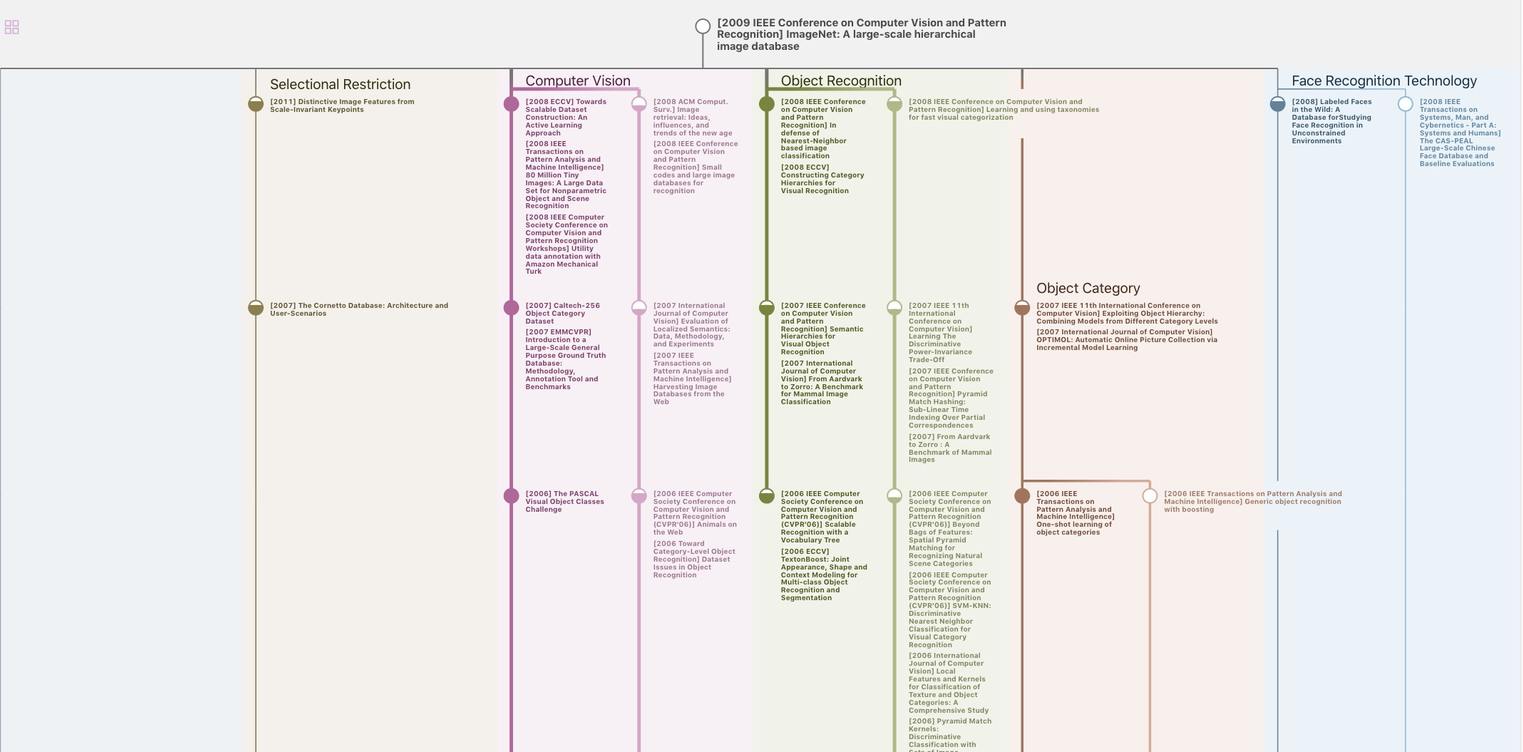
生成溯源树,研究论文发展脉络
Chat Paper
正在生成论文摘要