A Data-Driven Analysis of Behaviors in Data Curation Processes
ACM Transactions on Information Systems(2022)
摘要
Understanding how data workers interact with data, and various pieces of information related to data preparation, is key to designing systems that can better support them in exploring datasets. To date, however, there is a paucity of research studying the strategies adopted by data workers as they carry out data preparation activities. In this work, we investigate a specific data preparation activity, namely data quality discovery , and aim to (i) understand the behaviors of data workers in discovering data quality issues, (ii) explore what factors (e.g., prior experience) can affect their behaviors, as well as (iii) understand how these behavioral observations relate to their performance. To this end, we collect a multi-modal dataset through a data-driven experiment that relies on the use of eye-tracking technology with a purpose-designed platform built on top of iPython Notebook. The experiment results reveal that: (i) ‘copy–paste–modify’ is a typical strategy for writing code to complete tasks; (ii) proficiency in writing code has a significant impact on the quality of task performance, while perceived difficulty and efficacy can influence task completion patterns; and (iii) searching in external resources is a prevalent action that can be leveraged to achieve better performance. Furthermore, our experiment indicates that providing sample code within the system can help data workers get started with their task, and surfacing underlying data is an effective way to support exploration. By investigating data worker behaviors prior to each search action, we also find that the most common reasons that trigger external search actions are the need to seek assistance in writing or debugging code and to search for relevant code to reuse. Based on our experiment results, we showcase a systematic approach to select from the top best code snippets created by data workers and assemble them to achieve better performance than the best individual performer in the dataset. By doing so, our findings not only provide insights into patterns of interactions with various system components and information resources when performing data curation tasks, but also build effective and efficient data curation processes through data workers’ collective intelligence.
更多查看译文
关键词
Interaction behavior,search pattern,data curation
AI 理解论文
溯源树
样例
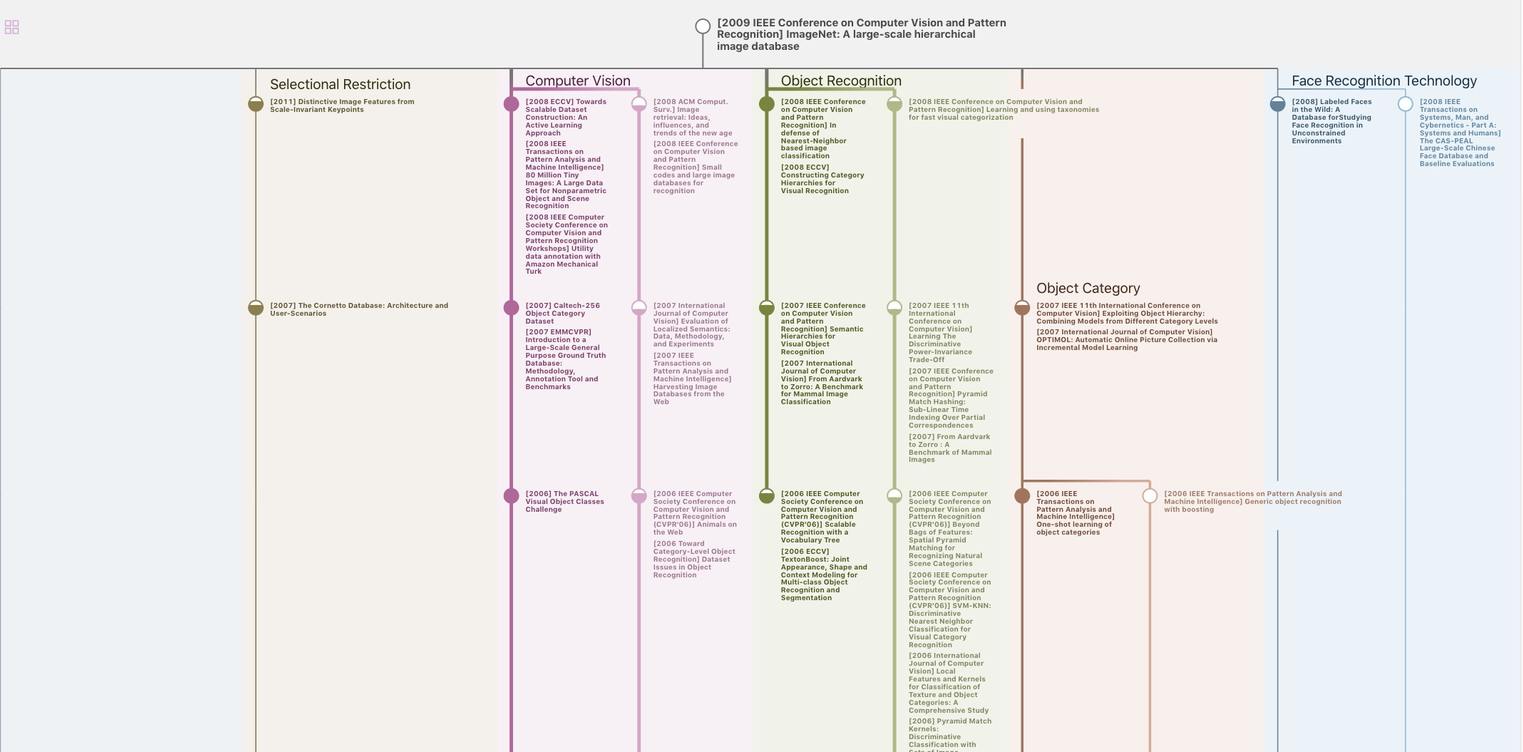
生成溯源树,研究论文发展脉络
Chat Paper
正在生成论文摘要