High quality monocular depth estimation with parallel decoder
Scientific reports(2022)
摘要
Monocular depth estimation aims to recover the depth information in three-dimensional (3D) space from a single image efficiently, but it is an ill-posed problem. Recently, Transformer-based architectures have achieved excellent accuracy in monocular depth estimation. However, due to the characteristics of Transformer, the model parameters are huge and the inference speed is slow. In traditional convolutional neural network–based architectures, many encoder-decoders perform serial fusion of the multi-scale features of each stage of the encoder and then output predictions. However, in these approaches it may be difficult to recover the spatial information lost by the encoder during pooling and convolution. To enhance this serial structure, we propose a structure from the decoder perspective, which first predicts global and local depth information in parallel and then fuses them. Results show that this structure is an effective improvement over traditional methods and has accuracy comparable with that of state-of-the-art methods in both indoor and outdoor scenes, but with fewer parameters and computations. Moreover, results of ablation studies verify the effectiveness of the proposed decoder.
更多查看译文
关键词
monocular depth estimation,high quality
AI 理解论文
溯源树
样例
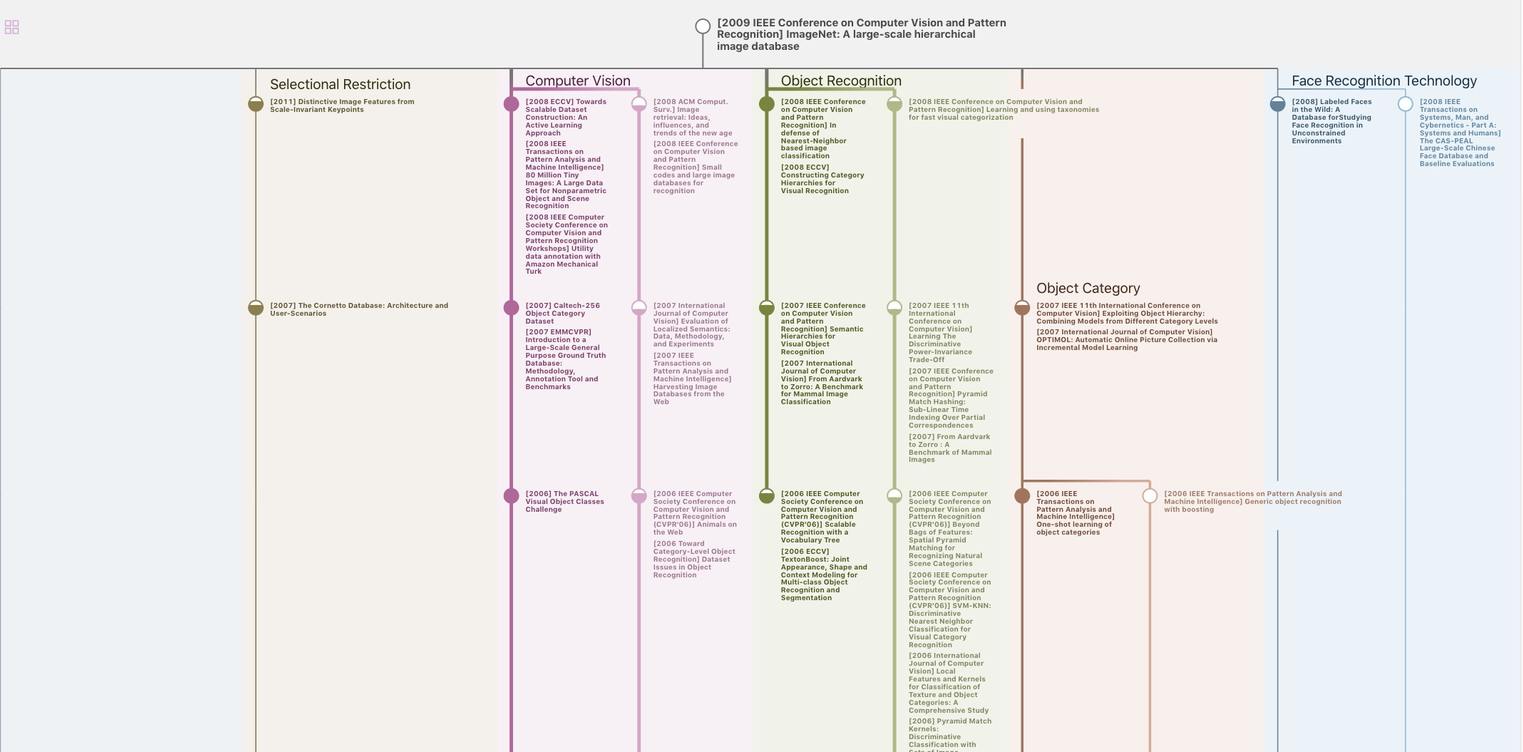
生成溯源树,研究论文发展脉络
Chat Paper
正在生成论文摘要