Cross-Modality Domain Adaptation for Freespace Detection: A Simple yet Effective Baseline
International Multimedia Conference(2022)
摘要
ABSTRACTAs one of the fundamental functions of autonomous driving system, freespace detection aims at classifying each pixel of the image captured by the camera as drivable or non-drivable. Current works of freespace detection heavily rely on large amount of densely labeled training data for accuracy and robustness, which is time-consuming and laborious to collect and annotate. To the best of our knowledge, we are the first work to explore unsupervised domain adaptation for freespace detection to alleviate the data limitation problem with synthetic data. We develop a cross-modality domain adaptation framework which exploits both RGB images and surface normal maps generated from depth images. A Collaborative Cross Guidance (CCG) module is proposed to leverage the context information of one modality to guide the other modality in a cross manner, thus realizing inter-modality intra-domain complement. To better bridge the domain gap between source domain (synthetic data) and target domain (real-world data), we also propose a Selective Feature Alignment (SFA) module which only aligns the features of consistent foreground area between the two domains, thus realizing inter-domain intra-modality adaptation. Extensive experiments are conducted by adapting three different synthetic datasets to one real-world dataset for freespace detection respectively. Our method performs closely to fully supervised freespace detection methods (93.08% v.s. 97.50% F1 score) and outperforms other general unsupervised domain adaptation methods for semantic segmentation with large margins, which shows the promising potential of domain adaptation for freespace detection.
更多查看译文
关键词
freespace detection,domain,cross-modality
AI 理解论文
溯源树
样例
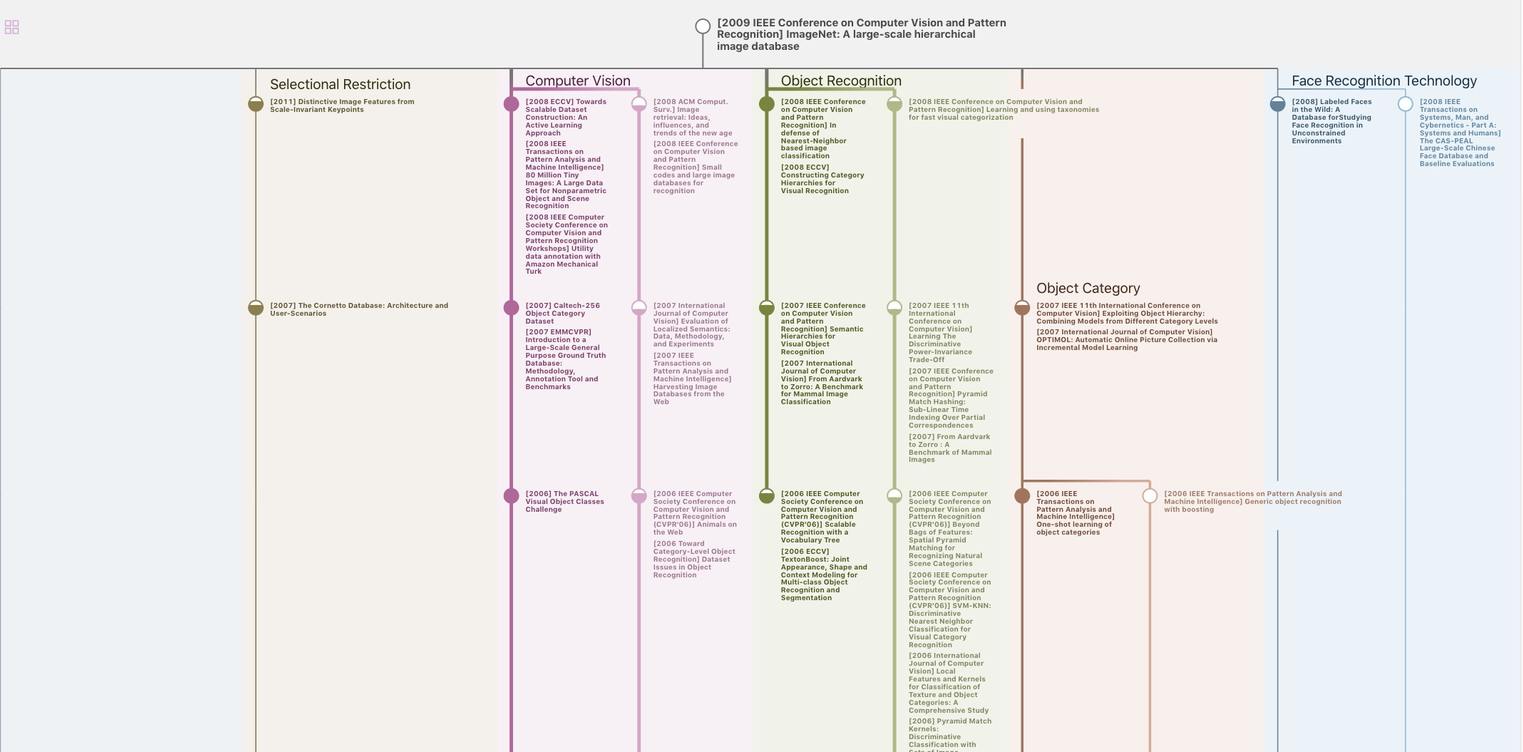
生成溯源树,研究论文发展脉络
Chat Paper
正在生成论文摘要