Few-Shot Generation of Personalized Neural Surrogates for Cardiac Simulation via Bayesian Meta-learning
MEDICAL IMAGE COMPUTING AND COMPUTER ASSISTED INTERVENTION, MICCAI 2022, PT VIII(2022)
摘要
Clinical adoption of personalized virtual heart simulations faces challenges in model personalization and expensive computation. While an ideal solution is an efficient neural surrogate that at the same time is personalized to an individual subject, the state-of-the-art is either concerned with personalizing an expensive simulation model, or learning an efficient yet generic surrogate. This paper presents a completely new concept to achieve personalized neural surrogates in a single coherent framework of meta-learning (metaPNS). Instead of learning a single neural surrogate, we pursue the process of learning a personalized neural surrogate using a small amount of context data from a subject, in a novel formulation of few-shot generative modeling underpinned by: 1) a set-conditioned neural surrogate for cardiac simulation that, conditioned on subject-specific context data, learns to generate query simulations not included in the context set, and 2) a meta-model of amortized variational inference that learns to condition the neural surrogate via simple feed-forward embedding of context data. As test time, metaPNS delivers a personalized neural surrogate by fast feed-forward embedding of a small and flexible number of data available from an individual, achieving - for the first time - personalization and surrogate construction for expensive simulations in one end-to-end learning framework. Synthetic and real-data experiments demonstrated that metaPNS was able to improve personalization and predictive accuracy in comparison to conventionally-optimized cardiac simulation models, at a fraction of computation.
更多查看译文
关键词
Cardiac electrophysiology, Personalization, Meta-learning
AI 理解论文
溯源树
样例
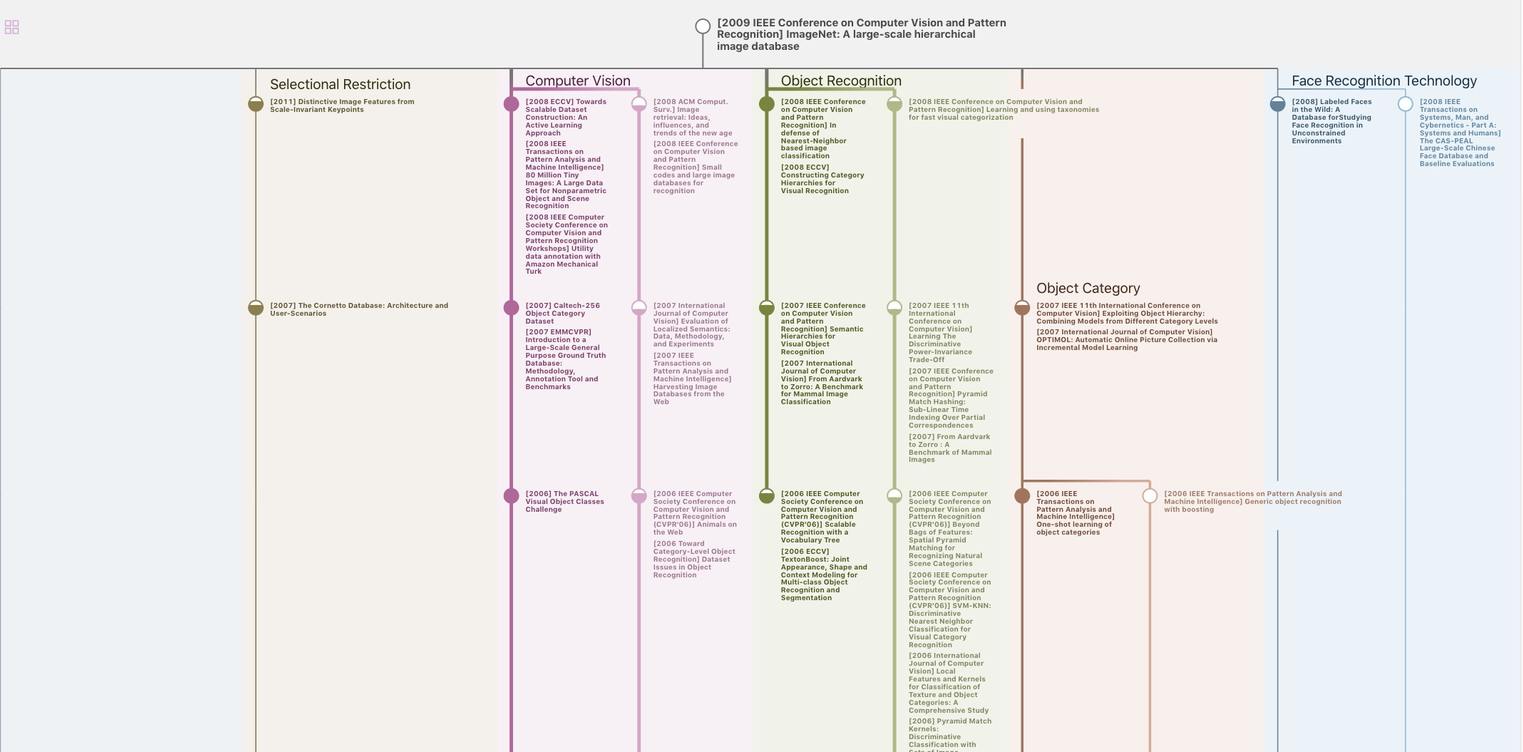
生成溯源树,研究论文发展脉络
Chat Paper
正在生成论文摘要