Leveraging Instance Features for Label Aggregation in Programmatic Weak Supervision
arxiv(2022)
摘要
Programmatic Weak Supervision (PWS) has emerged as a widespread paradigm to synthesize training labels efficiently. The core component of PWS is the label model, which infers true labels by aggregating the outputs of multiple noisy supervision sources abstracted as labeling functions (LFs). Existing statistical label models typically rely only on the outputs of LF, ignoring the instance features when modeling the underlying generative process. In this paper, we attempt to incorporate the instance features into a statistical label model via the proposed FABLE. In particular, it is built on a mixture of Bayesian label models, each corresponding to a global pattern of correlation, and the coefficients of the mixture components are predicted by a Gaussian Process classifier based on instance features. We adopt an auxiliary variable-based variational inference algorithm to tackle the non-conjugate issue between the Gaussian Process and Bayesian label models. Extensive empirical comparison on eleven benchmark datasets sees FABLE achieving the highest averaged performance across nine baselines.
更多查看译文
关键词
label aggregation,programmatic weak
AI 理解论文
溯源树
样例
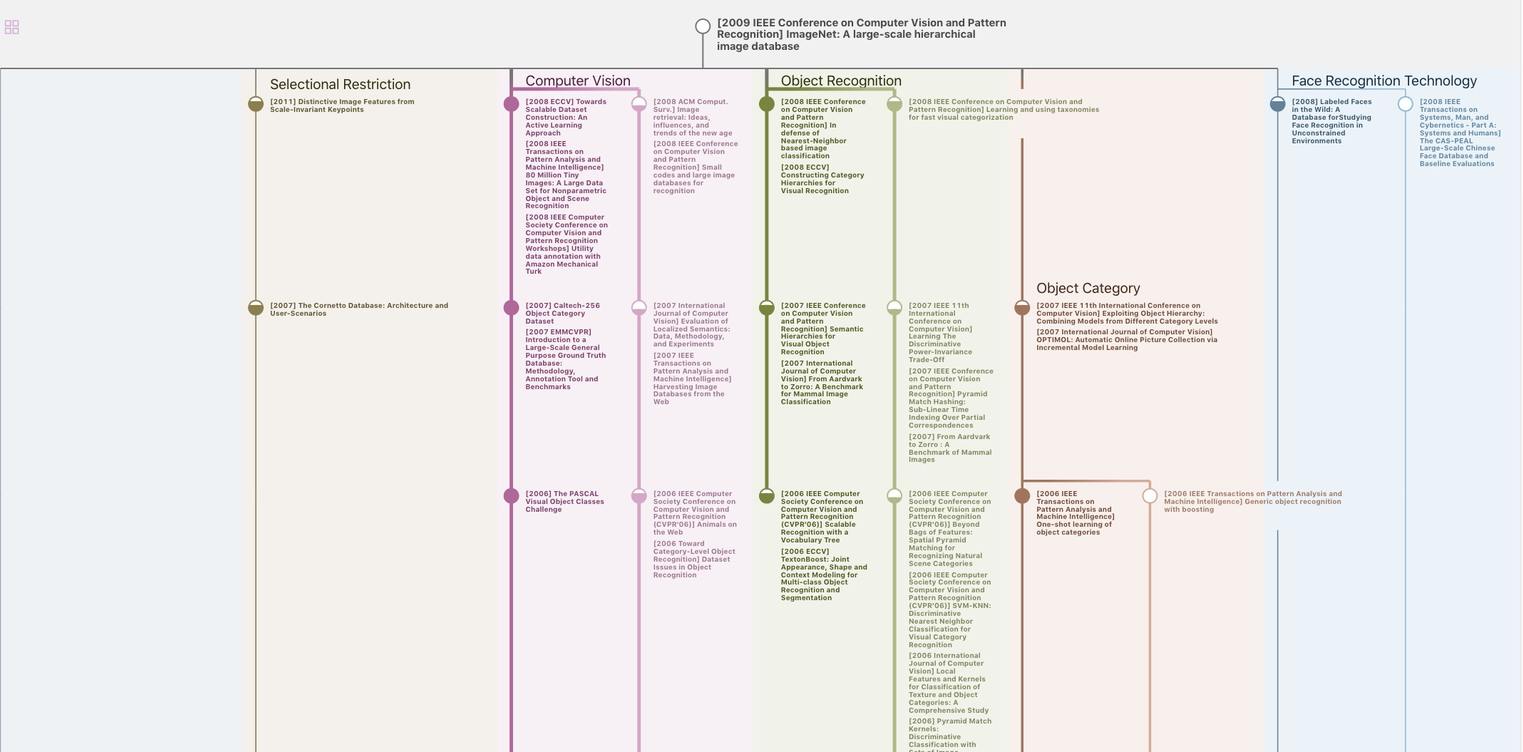
生成溯源树,研究论文发展脉络
Chat Paper
正在生成论文摘要