Federated Learning with Server Learning: Enhancing Performance for Non-IID Data
arxiv(2023)
摘要
Federated Learning (FL) has emerged as a means of distributed learning using local data stored at clients with a coordinating server. Recent studies showed that FL can suffer from poor performance and slower convergence when training data at clients are not independent and identically distributed. Here we consider a new complementary approach to mitigating this performance degradation by allowing the server to perform auxiliary learning from a small dataset. Our analysis and experiments show that this new approach can achieve significant improvements in both model accuracy and convergence time even when the server dataset is small and its distribution differs from that of the aggregated data from all clients.
更多查看译文
关键词
server learning,non-iid
AI 理解论文
溯源树
样例
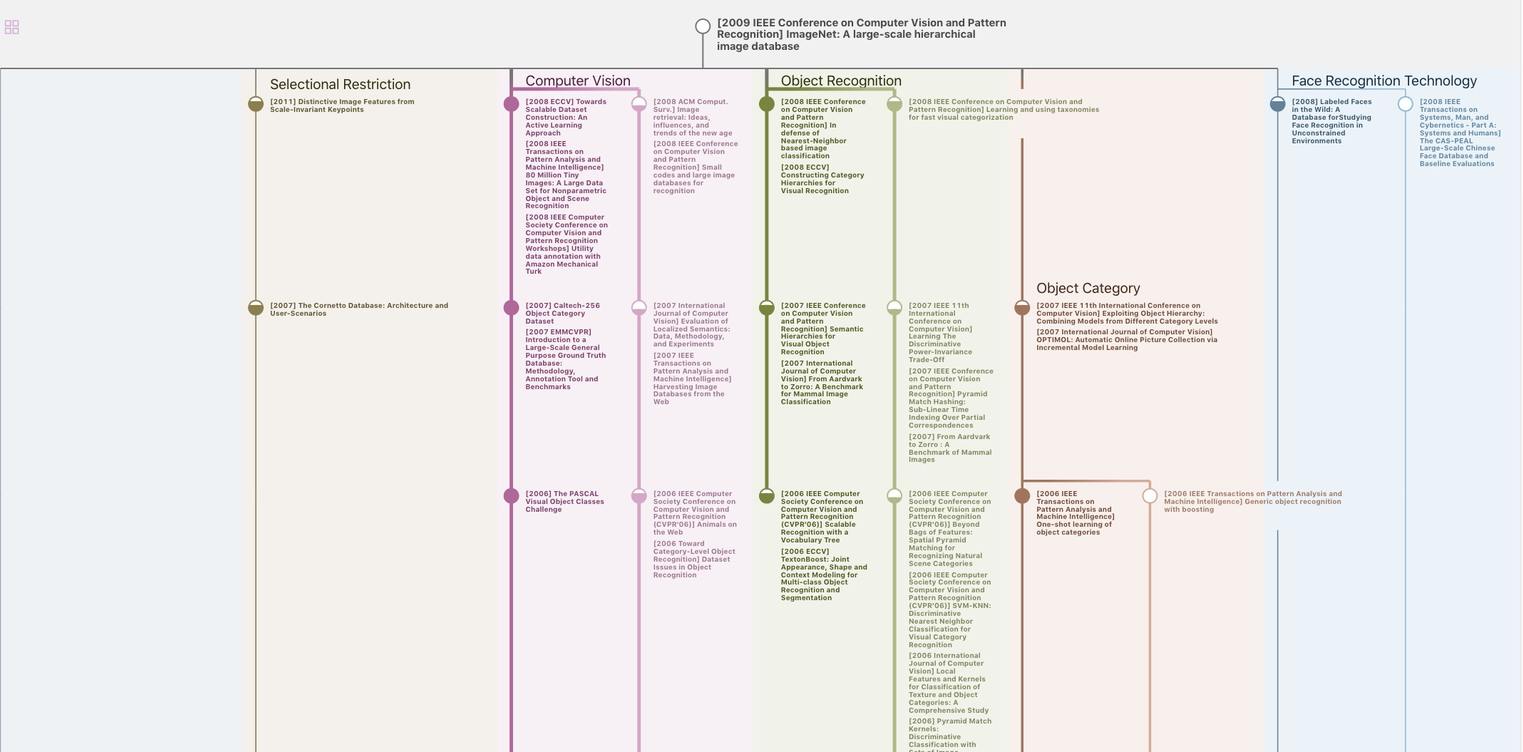
生成溯源树,研究论文发展脉络
Chat Paper
正在生成论文摘要