Adversarial attacks on graph-level embedding methods: a case study
Annals of Mathematics and Artificial Intelligence(2022)
摘要
As the number of graph-level embedding techniques increases at an unprecedented speed, questions arise about their behavior and performance when training data undergo perturbations. This is the case when an external entity maliciously alters training data to invalidate the embedding. This paper explores the effects of such attacks on some graph datasets by applying different graph-level embedding techniques. The main attack strategy involves manipulating training data to produce an altered model. In this context, our goal is to go in-depth about methods, resources, experimental settings, and performance results to observe and study all the aspects that derive from the attack stage.
更多查看译文
关键词
Adversarial attacks,Adversarial machine learning,Graph embedding,Graph neural networks,Graph classification
AI 理解论文
溯源树
样例
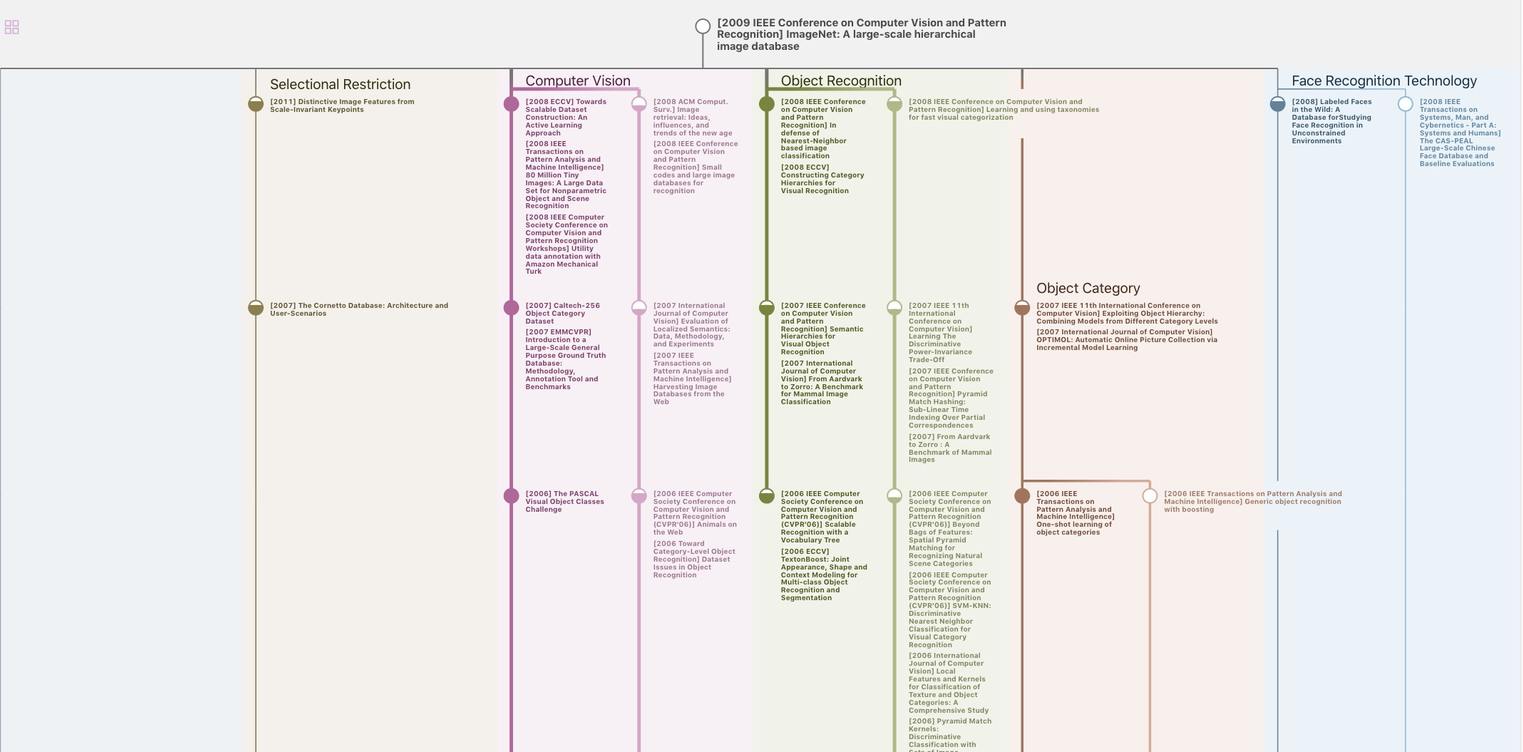
生成溯源树,研究论文发展脉络
Chat Paper
正在生成论文摘要