On Neural Consolidation for Transfer in Reinforcement Learning
arxiv(2022)
摘要
Although transfer learning is considered to be a milestone in deep reinforcement learning, the mechanisms behind it are still poorly understood. In particular, predicting if knowledge can be transferred between two given tasks is still an unresolved problem. In this work, we explore the use of network distillation as a feature extraction method to better understand the context in which transfer can occur. Notably, we show that distillation does not prevent knowledge transfer, including when transferring from multiple tasks to a new one, and we compare these results with transfer without prior distillation. We focus our work on the Atari benchmark due to the variability between different games, but also to their similarities in terms of visual features.
更多查看译文
关键词
neural consolidation,reinforcement learning,transfer
AI 理解论文
溯源树
样例
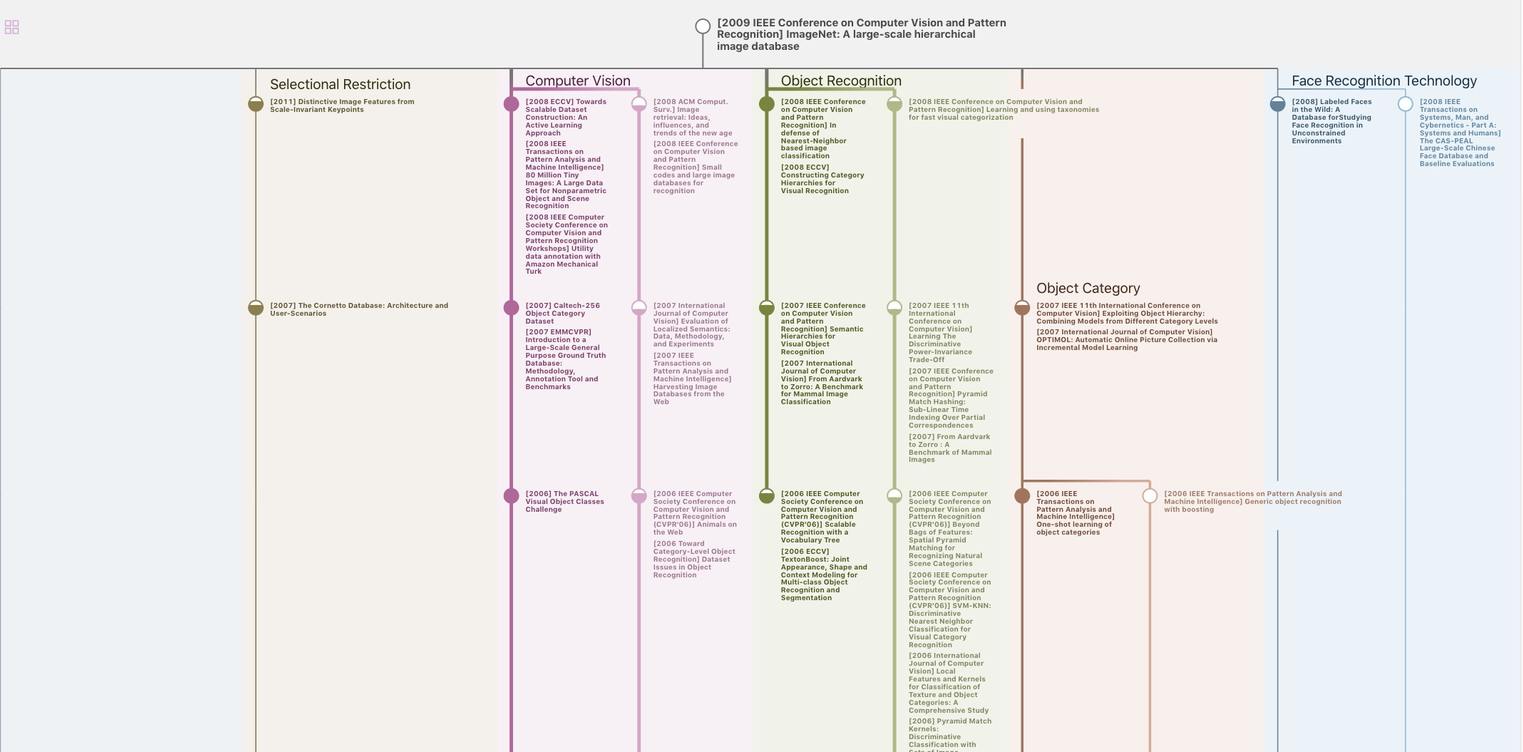
生成溯源树,研究论文发展脉络
Chat Paper
正在生成论文摘要