Learning Dynamic Abstract Representations for Sample-Efficient Reinforcement Learning
arxiv(2022)
摘要
In many real-world problems, the learning agent needs to learn a problem's abstractions and solution simultaneously. However, most such abstractions need to be designed and refined by hand for different problems and domains of application. This paper presents a novel top-down approach for constructing state abstractions while carrying out reinforcement learning. Starting with state variables and a simulator, it presents a novel domain-independent approach for dynamically computing an abstraction based on the dispersion of Q-values in abstract states as the agent continues acting and learning. Extensive empirical evaluation on multiple domains and problems shows that this approach automatically learns abstractions that are finely-tuned to the problem, yield powerful sample efficiency, and result in the RL agent significantly outperforming existing approaches.
更多查看译文
关键词
Sequential Decision-Making,Reinforcement Learning,Learning Abstract Representations
AI 理解论文
溯源树
样例
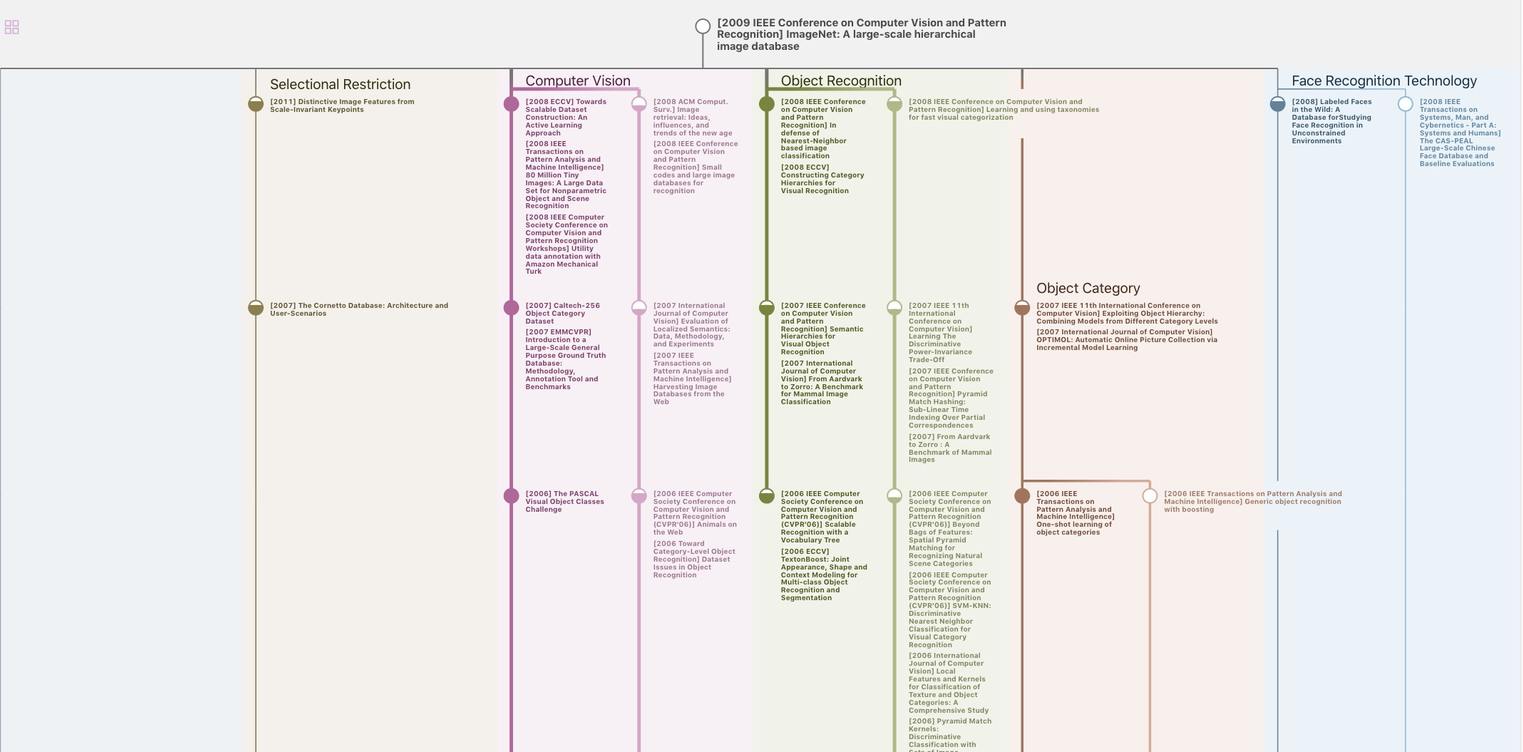
生成溯源树,研究论文发展脉络
Chat Paper
正在生成论文摘要