Training Data Selection and Dimensionality Reduction for Polynomial and Artificial Neural Network MIMO Adaptive Digital Predistortion
IEEE Transactions on Microwave Theory and Techniques(2022)
摘要
In 5G and beyond radios, the increased bandwidth, the fast-changing waveform scenarios, and the operation of large array multiple-input multiple-output (MIMO) transmitter architectures have challenged both the polynomial and the artificial neural network (ANN) MIMO adaptive digital predistortion (DPD) schemes. This article proposes training data selection methods and dimensionality reduction techniques that can be combined to enable relevant reductions of the DPD training time and the implementation complexity for MIMO transmitter architectures. In this work, the combination of an efficient uncorrelated equation selection (UES) mechanism together with orthogonal least squares (OLS) is proposed to reduce the training data length and the number of basis functions at every behavioral modeling matrix in the polynomial MIMO DPD scheme. For ANN MIMO DPD architectures, applying UES and principal component analysis (PCA) is proposed to reduce the input dataset length and features, respectively. The UES-OLS and the UES-PCA techniques are experimentally validated for a 2 x 2 MIMO test setup with strong power amplifier (PA) input and output crosstalk.
更多查看译文
关键词
Artificial neural networks (ANNs),digital predistortion (DPD),machine learning multiple-input multiple-output (MIMO),power amplifier (PA)
AI 理解论文
溯源树
样例
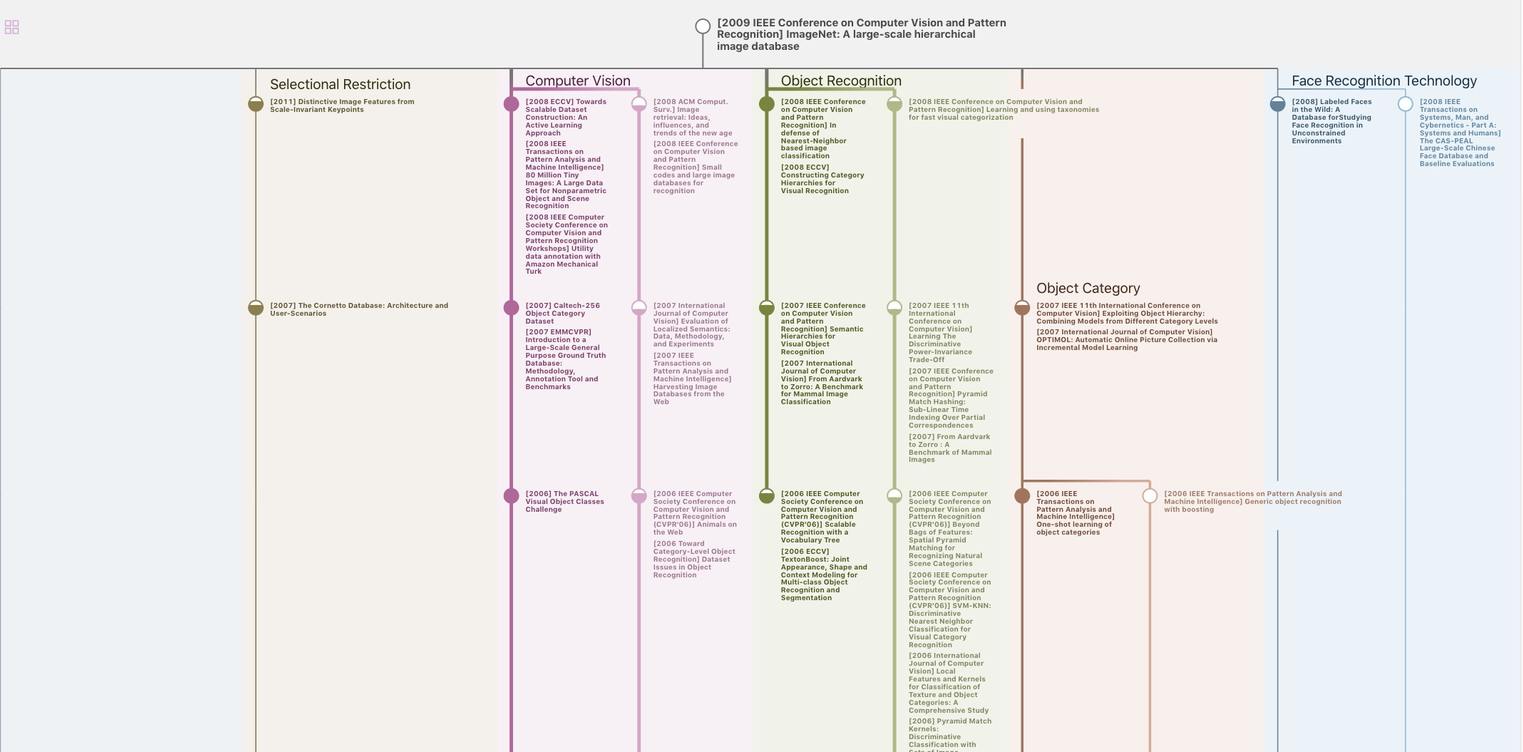
生成溯源树,研究论文发展脉络
Chat Paper
正在生成论文摘要